Develop A Hybrid Improved Smooth Support Vector with A Modified Pigeon Search Optimization to Detect the Diabetes Mellitus at Early Stage
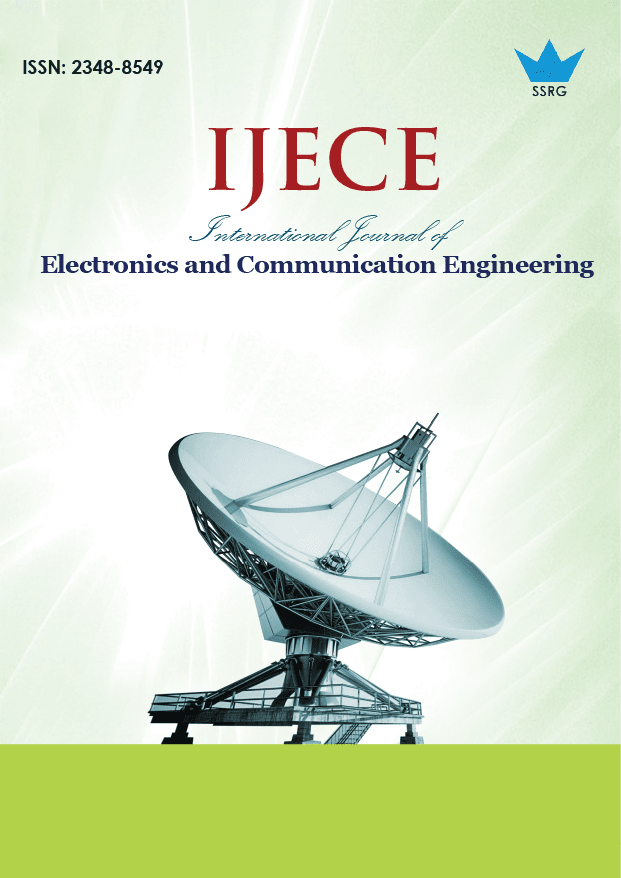
International Journal of Electronics and Communication Engineering |
© 2024 by SSRG - IJECE Journal |
Volume 11 Issue 3 |
Year of Publication : 2024 |
Authors : D. Arun, R. Annamalai Saravanan |
How to Cite?
D. Arun, R. Annamalai Saravanan, "Develop A Hybrid Improved Smooth Support Vector with A Modified Pigeon Search Optimization to Detect the Diabetes Mellitus at Early Stage," SSRG International Journal of Electronics and Communication Engineering, vol. 11, no. 3, pp. 63-76, 2024. Crossref, https://doi.org/10.14445/23488549/IJECE-V11I3P107
Abstract:
Diabetes Mellitus (DM) is a typical metabolic disease in which individuals struggle with high blood sugar (i.e., chronic hyperglycemia). It affects most of the body parts, such as the heart, kidneys, eyes, feet, skin, etc. Several competent Diabetes Diagnosis Systems (DDS) exploit different Machine Learning (ML) algorithms to gain valuable insights from the clinical datasets for DDS and disease management. However, trapping into local optimum solution, lack of privacy, missing values in the input dataset, and deficiency of incremental classification are major issues related to conventional ML-based diabetes classification algorithms. The primary objective of this study is to create an effective DM classification model that can reliably identify patient data as normal or diabetic. A hybrid Improved Smooth Support Vector Machine (ISSVM) with a Modified Pigeon Search Optimization (MPSO) algorithm was developed to detect the DM at an earlier stage. The empirical results reveal that the proposed classifiers outperform other related classifiers of existing systems regarding designated performance measures. These proposed algorithms can support clinicians in enabling the secured and accurate classification of DM with better accuracy and other performance measures.
Keywords:
DM, Machine Learning, Improved Smooth Support Vector Machine, Modified Pigeon Search Optimization, Diabetes Diagnosis Systems, Performance measures.
References:
[1] Victor Chang et al., “Pima Indians Diabetes Mellitus Classification Based on Machine Learning (ML) Algorithms,” Neural Computing and Applications, vol. 35, no. 22, pp. 16157-16173, 2023.
[CrossRef] [Google Scholar] [Publisher Link]
[2] Salliah Shafi Bhat et al., “A Risk Assessment and Prediction Framework for Diabetes Mellitus Using Machine Learning Algorithms,” Healthcare Analytics, vol. 4, pp. 1-12, 2023.
[CrossRef] [Google Scholar] [Publisher Link]
[3] Md. Jamal Uddin et al., “A Comparison of Machine Learning Techniques for the Detection of Type-2 Diabetes Mellitus: Experiences from Bangladesh,” Information, vol. 14, no. 7, pp. 1-19, 2023.
[CrossRef] [Google Scholar] [Publisher Link]
[4] Amin Mansoori et al., “Prediction of Type 2 Diabetes Mellitus Using Hematological Factors Based on Machine Learning Approaches: A Cohort Study Analysis,” Scientific Reports, vol. 13, pp. 1-11, 2023.
[CrossRef] [Google Scholar] [Publisher Link]
[5] Qiuhan Lu et al., “Longitudinal Metabolomics Integrated with Machine Learning Identifies Novel Biomarkers of Gestational Diabetes Mellitus,” Free Radical Biology and Medicine, vol. 209, pp. 9-17, 2023.
[CrossRef] [Google Scholar] [Publisher Link]
[6] Kirti Kangra, and Jaswinder Singh, “Comparative Analysis of Predictive Machine Learning Algorithms for Diabetes Mellitus,” Bulletin of Electrical Engineering and Informatics, vol. 12, no. 3, pp. 1728-1737, 2023.
[CrossRef] [Google Scholar] [Publisher Link]
[7] Chukwuebuka Joseph Ejiyi et al., “A Robust Predictive Diagnosis Model for Diabetes Mellitus Using Shapley-Incorporated Machine Learning Algorithms,” Healthcare Analytics, vol. 3, pp. 1-11, 2023.
[CrossRef] [Google Scholar] [Publisher Link]
[8] Hind Alamro et al., “Type 2 Diabetes Mellitus and Its Comorbidity, Alzheimer’s Disease: Identifying Critical microRNA Using Machine Learning,” Frontiers in Endocrinology, vol. 13, pp. 1-13, 2022.
[CrossRef] [Google Scholar] [Publisher Link]
[9] Rashmi Ashtagi et al., “IoT-Based Hybrid Ensemble Machine Learning Model for Efficient Diabetes Mellitus Prediction,” International Journal of Intelligent Systems and Applications in Engineering, vol. 11, no. 10s, pp. 714-726, 2023.
[Google Scholar] [Publisher Link]
[10] Durga Parkhi et al., “Prediction of Postpartum Prediabetes by Machine Learning Methods in Women with Gestational Diabetes Mellitus,” Iscience, vol. 26, no. 10, pp. 1-16, 2023.
[CrossRef] [Google Scholar] [Publisher Link]
[11] Ruth Sim et al., “Comparison of a Chronic Kidney Disease Predictive Model for Type 2 Diabetes Mellitus in Malaysia Using Cox Regression versus Machine Learning Approach,” Clinical Kidney Journal, vol. 16, no. 3, pp. 549-559, 2023.
[CrossRef] [Google Scholar] [Publisher Link]
[12] Jun Zhang et al., “Machine Learning for Post-Acute Pancreatitis Diabetes Mellitus Prediction and Personalized Treatment Recommendations,” Scientific Reports, vol. 13, pp. 1-10, 2023.
[CrossRef] [Google Scholar] [Publisher Link]
[13] Sven H. Loosen et al., “Prediction of New-Onset Diabetes Mellitus within 12 Months after Liver Transplantation-A Machine Learning Approach,” Journal of Clinical Medicine, vol. 12, no. 14, pp. 1-12, 2023.
[CrossRef] [Google Scholar] [Publisher Link]
[14] Yan Li et al., “Machine Learning-Based Models to Predict One-Year Mortality among Chinese Older Patients with Coronary Artery Disease Combined with Impaired Glucose Tolerance or Diabetes Mellitus,” Cardiovascular Diabetology, vol. 22, pp. 1-10, 2023.
[CrossRef] [Google Scholar] [Publisher Link]
[15] Mei-Yuan Liu et al., “Implementing A Novel Machine Learning System for Nutrition Education in Diabetes Mellitus Nutritional Clinic: Predicting 1-Year Blood Glucose Control,” Bioengineering, vol. 10, no. 10, pp. 1-13, 2023.
[CrossRef] [Google Scholar] [Publisher Link]
[16] J. Jeba Sonia et al., “Machine-Learning-Based Diabetes Mellitus Risk Prediction Using Multi-Layer Neural Network No-Prop Algorithm,” Diagnostics, vol. 13, no. 4, pp. 1-16, 2023.
[CrossRef] [Google Scholar] [Publisher Link]
[17] Kazuya Fujihara, and Hirohito Sone, “Machine Learning Approach to Drug Treatment Strategy for Diabetes Care,” Diabetes & Metabolism Journal, vol. 47, no. 3, pp. 325-332, 2023.
[CrossRef] [Google Scholar] [Publisher Link]
[18] Chengyi Feng et al., “Machine Learning Models for Prediction of Invasion Klebsiella Pneumoniae Liver Abscess Syndrome in Diabetes Mellitus: A Singled Centered Retrospective Study,” BMC Infectious Diseases, vol. 23, no. 1, pp. 1-12, 2023.
[CrossRef] [Google Scholar] [Publisher Link]
[19] Min Zhao et al., “A Machine Learning-Based Diagnosis Modeling of Type 2 Diabetes Mellitus with Environmental Metal Exposure,” Computer Methods and Programs in Biomedicine, vol. 235, 2023.
[CrossRef] [Google Scholar] [Publisher Link]
[20] Andrya J. Durr et al., “Machine Learning for Spatial Stratification of Progressive Cardiovascular Dysfunction in a Murine Model of Type 2 Diabetes Mellitus,” Plos One, vol. 18, no. 5, 2023.
[CrossRef] [Google Scholar] [Publisher Link]
[21] Ji-Yoon Lee, Doyeon Won, and Kiheon Lee, “Machine Learning‐Based Identification and Related Features of Depression in Patients with Diabetes Mellitus Based on the Korea National Health and Nutrition Examination Survey: A Cross-Sectional Study,” Plos One, vol. 18, no. 7, 2023.
[CrossRef] [Google Scholar] [Publisher Link]
[22] Khoula Al Sadi, and Wamadeva Balachandran, “Prediction Model of Type 2 Diabetes Mellitus for Oman Prediabetes Patients Using Artificial Neural Network and Six Machine Learning Classifiers,” Applied Sciences, vol. 13, no. 4, pp. 1-22, 2023.
[CrossRef] [Google Scholar] [Publisher Link]
[23] Aidar Alimbayev et al., “Predicting 1-Year Mortality of Patients with Diabetes Mellitus in Kazakhstan Based on Administrative Health Data Using Machine Learning,” Scientific Reports, vol. 13, pp. 1-12, 2023.
[CrossRef] [Google Scholar] [Publisher Link]
[24] Evangelos K. Oikonomou, and Rohan Khera, “Machine Learning in Precision Diabetes Care and Cardiovascular Risk Prediction,” Cardiovascular Diabetology, vol. 22, pp. 1-16, 2023.
[CrossRef] [Google Scholar] [Publisher Link]
[25] Jinru Ding et al., “Machine Learning for the Prediction of Atherosclerotic Cardiovascular Disease during 3‐Year Follow-Up in Chinese Type 2 Diabetes Mellitus Patients,” Journal of Diabetes Investigation, vol. 14, no. 11, pp. 1289-1302, 2023.
[CrossRef] [Google Scholar] [Publisher Link]
[26] Sen Wang et al., “Identification of Ferroptosis‐Related Genes in Type 2 Diabetes Mellitus Based on Machine Learning,” Immunity, Inflammation and Disease, vol. 11, no. 10, 2023.
[CrossRef] [Google Scholar] [Publisher Link]
[27] Apoorva S. Chauhan et al., “Prediction of Diabetes Mellitus Progression Using Supervised Machine Learning,” Sensors, vol. 23, no. 10, pp. 1-16, 2023.
[CrossRef] [Google Scholar] [Publisher Link]
[28] Yun-Nam Chan et al., “A Machine Learning Approach for Early Prediction of Gestational Diabetes Mellitus Using Elemental Contents in Fingernails,” Scientific Reports, vol. 13, pp. 1-11, 2023.
[CrossRef] [Google Scholar] [Publisher Link]
[29] Xuelun Wu et al., “Development of Machine Learning Models for Predicting Osteoporosis in Patients with Type 2 Diabetes Mellitus-A Preliminary Study,” Diabetes, Metabolic Syndrome and Obesity, vol. 16, pp. 1987-2003, 2024.
[CrossRef] [Google Scholar] [Publisher Link]
[30] Pooneh Khodabakhsh et al., “Prediction of In-Hospital Mortality Rate in COVID-19 Patients with Diabetes Mellitus Using Machine Learning Methods,” Journal of Diabetes & Metabolic Disorders, vol. 22, pp. 1177-1190, 2023.
[CrossRef] [Google Scholar] [Publisher Link]
[31] Junli Zhang et al., “Prediction of the Risk of Bone Mineral Density Decrease in Type 2 Diabetes Mellitus Patients Based on Traditional Multivariate Logistic Regression and Machine Learning: A Preliminary Study,” Diabetes, Metabolic Syndrome and Obesity, vol. 16, pp. 2885-2898, 2024.
[CrossRef] [Google Scholar] [Publisher Link]
[32] Yi-Ling Cheng et al., “Using Machine Learning for the Risk Factors Classification of Glycemic Control in Type 2 Diabetes Mellitus,” Healthcare, vol. 11, no. 8, pp. 1-9, 2023.
[CrossRef] [Google Scholar] [Publisher Link]
[33] Xiaoqi Hu et al., “Prediction Model for Gestational Diabetes Mellitus Using the XG Boost Machine Learning Algorithm,” Frontiers in Endocrinology, vol. 14, pp. 1-10, 2023.
[CrossRef] [Google Scholar] [Publisher Link]
[34] Qinpei Zhao et al., “Chinese Diabetes Datasets for Data-Driven Machine Learning,” Scientific Data, vol. 10, pp. 1-8, 2023.
[CrossRef] [Google Scholar] [Publisher Link]
[35] Hong Pan et al., “A Risk Prediction Model for Type 2 Diabetes Mellitus Complicated with Retinopathy Based on Machine Learning and Its Application in Health Management,” Frontiers in Medicine, vol. 10, pp. 1-15, 2023.
[CrossRef] [Google Scholar] [Publisher Link]
[36] Shubashini Rathina Velu, Vinayakumar Ravi, and Kayalvily Tabianan, “Machine Learning Implementation to Predict Type-2 Diabetes Mellitus Based on Lifestyle Behavior Patterns Using HBA1C Status,” Health and Technology, vol. 13, pp. 437-447, 2023.
[CrossRef] [Google Scholar] [Publisher Link]
[37] Vansh Gupta et al., “Comparative Study of Machine Learning Models for Early Gestational Diabetes Mellitus,” 2023 International Conference on Circuit Power and Computing Technologies (ICCPCT), pp. 1761-1766, 2023.
[CrossRef] [Google Scholar] [Publisher Link]
[38] Xinyu Liu et al., “Application of Machine Learning in Chinese Medicine Differentiation of Dampness-Heat Pattern in Patients with Type 2 Diabetes Mellitus,” Heliyon, vol. 9, no. 2, pp. 1-11, 2023.
[CrossRef] [Google Scholar] [Publisher Link]
[39] Ayasha Malik et al., “Prognosis of Diabetes Mellitus Based on Machine Learning Algorithms,” 2023 10th International Conference on Computing for Sustainable Global Development (INDIACom), Delhi, India, pp. 1466-1472, 2023.
[Google Scholar] [Publisher Link]
[40] Claudia C. Colmenares-Mejía et al., “Multivariable Prediction Model of Complications Derived from Diabetes Mellitus Using Machine Learning on Scarce Highly Unbalanced Data,” International Journal of Diabetes in Developing Countries, pp. 1-11, 2023.
[CrossRef] [Google Scholar] [Publisher Link]