An Improved Vectorization-Based Emotion Detection Using Tuned Inverse Document Frequency Approach
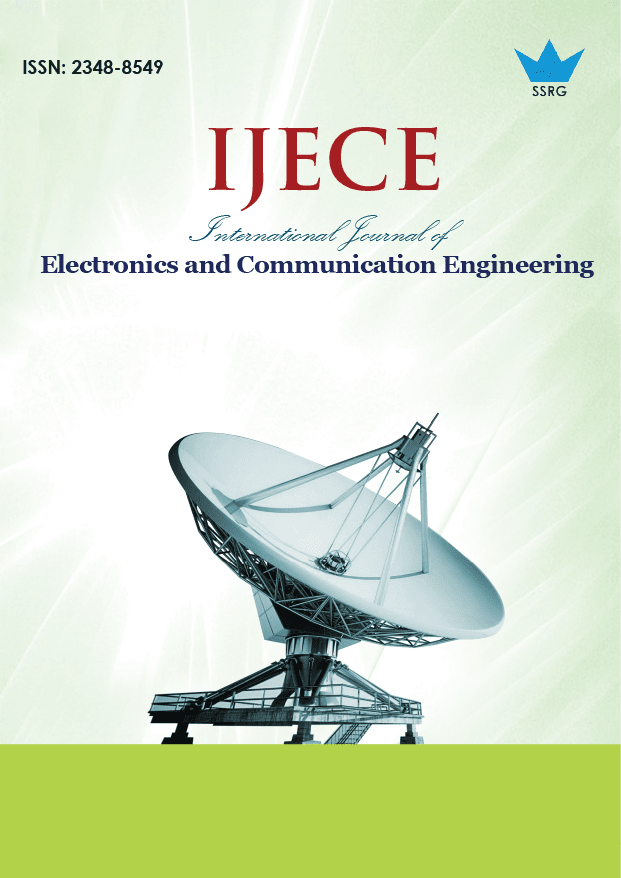
International Journal of Electronics and Communication Engineering |
© 2024 by SSRG - IJECE Journal |
Volume 11 Issue 3 |
Year of Publication : 2024 |
Authors : R. Vanitha, K. Sudharson, N. Sathish Kumar, S. Gunasundari |
How to Cite?
R. Vanitha, K. Sudharson, N. Sathish Kumar, S. Gunasundari, "An Improved Vectorization-Based Emotion Detection Using Tuned Inverse Document Frequency Approach," SSRG International Journal of Electronics and Communication Engineering, vol. 11, no. 3, pp. 106-114, 2024. Crossref, https://doi.org/10.14445/23488549/IJECE-V11I3P111
Abstract:
Emotion analysis of social media content has garnered significant attention due to its potential to reveal valuable insights into people’s feelings and opinions. This study is motivated by the need to understand better the emotions individuals express when posting their views on social media. The objective is to explore and compare the effectiveness of two machine learning methods, a Twin Support Vector Machine (TWSVM) and a novel approach called Tuned Inverse Document Frequency (TUNED-IDF) vectorizer, in accurately detecting emotions from textual data. To achieve this objective, the research process involves first applying the TWSVM algorithm, which examines the factors influencing emotions and their connection to the dependent variable. Next, our innovative TUNED-IDF vectorizer converts the textual content into numerical representations, leveraging its properties to improve accuracy in emotion analysis. The findings of this study showcase the remarkable performance of the TUNED-IDF approach, achieving an impressive accuracy level of 94.4%, surpassing existing methods in emotion detection. By employing this method, the research successfully predicts people’s emotions with higher precision and efficacy than traditional machine learning models. The significance of this research lies in its contribution to the field of emotion analysis, particularly in the context of social media. Understanding the emotions conveyed in online communication is crucial for various applications, such as sentiment analysis, market research, and public opinion monitoring. The insights gained from this study offer valuable opportunities for better comprehension of individuals’ sentiments in the digital age and lay the groundwork for enhanced emotion analysis techniques.
Keywords:
Vectorization, Tuned-IDF, Data mining, Classification, Supervised learning.
References:
[1] Pansy Nandwani, and Rupali Verma, “A Review on Sentiment Analysis and Emotion Detection from Text,” Social Network Analysis and Mining, vol. 11, 2021.
[CrossRef] [Google Scholar] [Publisher Link]
[2] Santosh Kumar Bharti et al., “Text-Based Emotion Recognition Using Deep Learning Approach,” Computational Intelligence and Neuroscience, vol. 2022, pp. 1-8, 2022.
[CrossRef] [Google Scholar] [Publisher Link]
[3] Kian Long Tan, Chin Poo Lee, and Kian Ming Lim, “A Survey of Sentiment Analysis: Approaches, Datasets, and Future Research,” Applied Sciences, vol. 13, no. 7, pp. 1-21, 2023.
[CrossRef] [Google Scholar] [Publisher Link]
[4] Muhammad Farrukh Bashir et al., “Context-Aware Emotion Detection from Low-Resource Urdu Language Using Deep Neural Network,” ACM Transactions on Asian and Low-Resource Language Information Processing, vol. 22, no. 5, pp. 1-30, 2023.
[CrossRef] [Google Scholar] [Publisher Link]
[5] Ranit Kumar Dey, and Asit Kumar Das, “Modified Term Frequency-Inverse Document Frequency Based Deep Hybrid Framework for Sentiment Analysis,” Multimedia Tools and Applications, vol. 82, pp. 32967-32990, 2023.
[CrossRef] [Google Scholar] [Publisher Link]
[6] Bagus Tris Atmaja, and Akira Sasou, “Sentiment Analysis and Emotion Recognition from Speech Using Universal Speech Representations,” Sensors, vol. 22, no. 17, pp. 1-11, 2022.
[CrossRef] [Google Scholar] [Publisher Link]
[7] Alaa Alslaity, and Rita Orji, “Machine Learning Techniques for Emotion Detection and Sentiment Analysis: Current State, Challenges, and Future Directions,” Behaviour & Information Technology, vol. 43, no. 1, pp. 139-164, 2022.
[CrossRef] [Google Scholar] [Publisher Link]
[8] Chris Jonathan D., and Sujitha Juliet, “Text-Based Emotion Recognition Using Sentiment Analysis,” 2022 International Conference on Applied Artificial Intelligence and Computing (ICAAIC), Salem, India, pp. 844-848, 2022.
[CrossRef] [Google Scholar] [Publisher Link]
[9] Pedamuthevi Kiran Kumar, and Ishan Kumar, “Emotion Detection and Sentiment Analysis of Text,” Proceedings of the International Conference on Innovative Computing & Communication (ICICC), pp. 1-4, 2021.
[CrossRef] [Google Scholar] [Publisher Link]
[10] Licai Sun et al., “Multimodal Emotion Recognition and Sentiment Analysis via Attention Enhanced Recurrent Model,” Proceedings of the 2nd on Multimodal Sentiment Analysis Challenge, pp. 15-20, 2021.
[CrossRef] [Google Scholar] [Publisher Link]
[11] Nilesh Shelke et al., “An Efficient Way of Text-Based Emotion Analysis from Social Media Using LRA-DNN,” Neuroscience Informatics, vol. 2, no. 3, pp. 1-10, 2022.
[CrossRef] [Google Scholar] [Publisher Link]
[12] Francisca Adoma Acheampong, Chen Wenyu, and Henry Nunoo-Mensah, “Text-Based Emotion Detection: Advances, Challenges, and Opportunities,” Engineering Reports, vol. 2, no. 7, 2020.
[CrossRef] [Google Scholar] [Publisher Link]
[13] Madhavi S. Darokar, Atul D. Raut, and Vilas M. Thakre, “Methodological Review of Emotion Recognition for Social Media: A Sentiment Analysis Approach,” 2021 International Conference on Computing, Communication and Green Engineering (CCGE), Pune, India, pp. 1- 5, 2021.
[CrossRef] [Google Scholar] [Publisher Link]
[14] Anjali Goswami et al., “Sentiment Analysis of Statements on Social Media and Electronic Media Using Machine and Deep Learning Classifiers,” Computational Intelligence and Neuroscience, vol. 2022, pp. 1-19, 2022.
[CrossRef] [Google Scholar] [Publisher Link]
[15] V. Jagadishwari et al., “Sentiment Analysis of Social Media Text-Emoticon Post with Machine Learning Models Contribution Title,” Journal of Physics: Conference Series, vol. 2070, pp. 1-8, 2021.
[CrossRef] [Google Scholar] [Publisher Link]
[16] Vong Anh Ho et al., “Emotion Recognition for Vietnamese Social Media Text,” International Conference of the Pacific Association for Computational Linguistics, pp. 319-333, 2020.
[CrossRef] [Google Scholar] [Publisher Link]
[17] Diandre de Paula, and Luís A. Alexandre, “Facial Emotion Recognition for Sentiment Analysis of Social Media Data,” Iberian Conference on Pattern Recognition and Image Analysis, pp. 207-217, 2022.
[CrossRef] [Google Scholar] [Publisher Link]
[18] Maria Krommyda et al., “An Experimental Analysis of Data Annotation Methodologies for Emotion Detection in Short Text Posted on Social Media,” Informatics, vol. 8, no. 1, pp. 1-15, 2021.
[CrossRef] [Google Scholar] [Publisher Link]
[19] Amira Samy Talaat, “Sentiment Analysis Classification System Using Hybrid BERT Models,” Journal of Big Data, vol. 10, no. 1, pp. 1- 18, 2023.
[CrossRef] [Google Scholar] [Publisher Link]
[20] Yafeng Ren et al., “Context-Sensitive Twitter Sentiment Classification Using Neural Network,” Proceedings of the AAAI Conference on Artificial Intelligence, vol. 30, no. 1, pp. 215-221, 2016.
[CrossRef] [Google Scholar] [Publisher Link]
[21] Barakat AlBadani, Ronghua Shi, and Jian Dong, “A Novel Machine Learning Approach for Sentiment Analysis on Twitter Incorporating the Universal Language Model Fine-Tuning and SVM,” Applied System Innovation, vol. 5, no. 1, pp. 1-16, 2022.
[CrossRef] [Google Scholar] [Publisher Link]
[22] Praphula Kumar Jain, Vijayalakshmi Saravanan, and Rajendra Pamula, “A Hybrid CNN-LSTM: A Deep Learning Approach for Consumer Sentiment Analysis Using Qualitative User-Generated Contents,” ACM Transactions on Asian and Low-Resource Language Information Processing, vol. 20, no. 5, pp. 1-15, 2021.
[CrossRef] [Google Scholar] [Publisher Link]
[23] Sadaf Hussain Janjua et al., “Multi-Level Aspect Based Sentiment Classification of Twitter Data: Using Hybrid Approach in Deep Learning,” PeerJ Computer Science, vol. 7, pp. 1-25, 2021.
[CrossRef] [Google Scholar] [Publisher Link]
[24] Ushmani Babar et al., “Data Mining Techniques for Sentiment Analysis Social Media Networking Using Different Information Resources,” Global Sci-Tech, vol. 13, no. 3&4, pp. 138-147, 2021.
[CrossRef] [Google Scholar] [Publisher Link]
[25] Deepak Kumar Jain et al., “An Intelligent Cognitive-Inspired Computing with Big Data Analytics Framework for Sentiment Analysis and Classification,” Information Processing & Management, vol. 59, no. 1, 2022.
[CrossRef] [Google Scholar] [Publisher Link]
[26] Monika Kabir et al., “An Empirical Research on Sentiment Analysis Using Machine Learning Approaches,” International Journal of Computers and Applications, vol. 43, no. 10, pp. 1011-1019, 2019.
[CrossRef] [Google Scholar] [Publisher Link]
[27] Hetu Patel, and Anilkumar Suthar, “Sentiment Analysis for Rating Cooking Recipes Based on User’s Feedback Using Data Mining Techniques (Classification),” Gedrag & Organisatie Review, vol. 34, no. 1, pp. 75-80, 2021.
[Google Scholar] [Publisher Link]
[28] Ukhti Ikhsani Larasati et al., “Improve the Accuracy of Support Vector Machine Using Chi Square Statistic and Term Frequency Inverse Document Frequency on Movie Review Sentiment Analysis,” Scientific Journal of Informatics, vol. 6, no. 1, pp. 138-149, 2019.
[CrossRef] [Google Scholar] [Publisher Link]
[29] Nur Syafiqah Mohd Nafis, and Suryanti Awang, “An Enhanced Hybrid Feature Selection Technique Using Term Frequency-Inverse Document Frequency and Support Vector Machine-Recursive Feature Elimination for Sentiment Classification,” IEEE Access, vol. 9, pp. 52177-52192, 2021.
[CrossRef] [Google Scholar] [Publisher Link]
[30] C.S. Anita, and R. Sasikumar, “Learning Automata and Lexical Composition Method for Optimal and Load Balanced RPL Routing in IoT,” International Journal of Ad Hoc and Ubiquitous Computing, vol. 40, no. 4, pp. 288-300, 2022.
[CrossRef] [Google Scholar] [Publisher Link]
[31] B. Sai Mani Teja et al., “A CNN Based Facial Expression Recognizer,” Materials Today: Proceedings, vol. 37, Part 2, pp. 2578-2581, 2021.
[CrossRef] [Google Scholar] [Publisher Link]