Transforming 3D Brain MRI Data: Building a Robust Preprocessing Pipeline
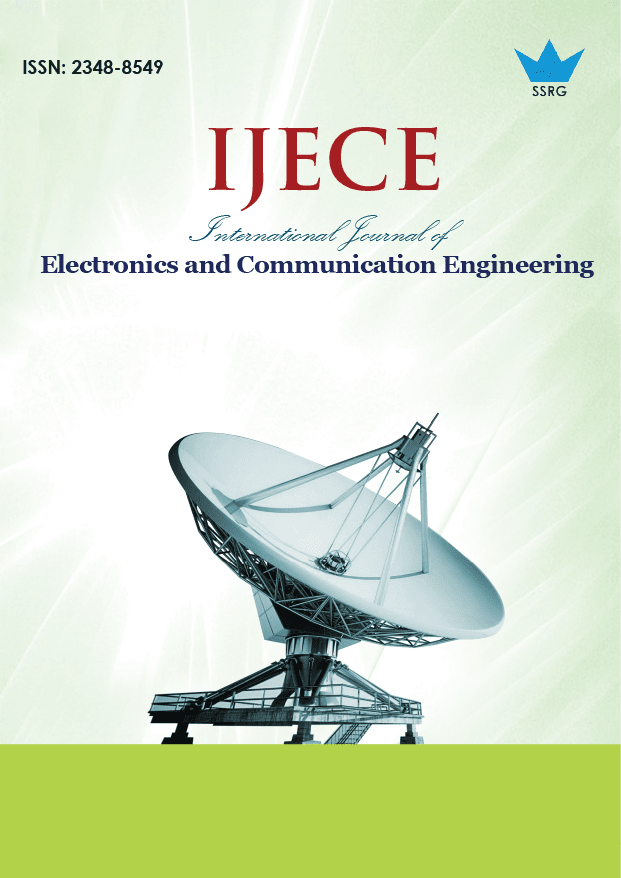
International Journal of Electronics and Communication Engineering |
© 2024 by SSRG - IJECE Journal |
Volume 11 Issue 4 |
Year of Publication : 2024 |
Authors : S. Yamuna, K. Vijayakumar |
How to Cite?
S. Yamuna, K. Vijayakumar, "Transforming 3D Brain MRI Data: Building a Robust Preprocessing Pipeline," SSRG International Journal of Electronics and Communication Engineering, vol. 11, no. 4, pp. 51-59, 2024. Crossref, https://doi.org/10.14445/23488549/IJECE-V11I4P106
Abstract:
Pre-processing pipelines for the conversion of 3D brain MRI (Magnetic Resonance Imaging) data into 2D formats are developed and optimized here with the goal of ensuring the quality of the data and compatibility with deep learning models. In order to prepare the MRI data for analysis, the pipeline involves several key steps, including data collection, pre-processing, and conversion. Pre-processing techniques are used to improve the quality and consistency of the MRI data, including denoising, motion correction, intensity normalization, and skull stripping. After pre-processing, the 3D MRI volumes are converted into 2D slices suitable for input into deep learning models, with consideration of slice selection and orientation. Data accuracy and reliability are ensured throughout the pipeline by rigorous quality control measures. Optimising pre-processing steps to align with model requirements to ensure compatibility with deep learning models is a priority. The resulting pre-processing pipeline facilitates the seamless integration of 3D brain MRI data into deep learning workflows, enabling advanced analysis and insights in the field of neuroimaging.
Keywords:
Brain MRI, Pre-processing pipeline, 3D to 2D conversion, Data quality, Denoising, Skull stripping.
References:
[1] Khurram Shahzad, and Wael Mati, “Advances in Magnetic Resonance Imaging (MRI),” Advances in Medical and Surgical Engineering, pp. 121-142, 2020.
[CrossRef] [Google Scholar] [Publisher Link]
[2] Abhinandan Banerjee et al., “High-Field Magnetic Resonance Imaging: Challenges, Advantages, and Opportunities for Novel Contrast Agents,” Chemical Physics Reviews, vol. 3, no. 1, 2022.
[CrossRef] [Google Scholar] [Publisher Link]
[3] Nivin N. Nyström et al., “A Genetically Encoded Magnetic Resonance Imaging Reporter Enables Sensitive Detection and Tracking of Spontaneous Metastases in Deep Tissues,” Cancer Research, vol. 83, no. 5, pp. 673-685, 2023.
[CrossRef] [Google Scholar] [Publisher Link]
[4] Hailong Hu et al., “Recent Advances of Bioresponsivenano-Sized Contrast Agents for Ultra-High-Field Magnetic Resonance Imaging,” Frontiers in Chemistry, vol. 8, pp. 1-20, 2020.
[CrossRef] [Google Scholar] [Publisher Link]
[5] Federico Bruno et al., “Advanced Magnetic Resonance Imaging (MRI) Techniques: Technical Principles and Applications in Nanomedicine,” Cancers, vol. 14, no. 7, pp. 1-17, 2022.
[CrossRef] [Google Scholar] [Publisher Link]
[6] Can Chen et al., “Ultrasmall Superparamagnetic Iron Oxide Nanoparticles: A Next Generation Contrast Agent for Magnetic Resonance Imaging,” Wiley Interdisciplinary Reviews: Nanomedicine and Nanobiotechnology, vol. 14, no. 1, 2022.
[CrossRef] [Google Scholar] [Publisher Link]
[7] Wei Zhao et al., “Advances and Prospects of RAFT Polymerization-Derived Nanomaterials in MRI-Assisted Biomedical Applications,” Progress in Polymer Science, vol. 146, 2023.
[CrossRef] [Google Scholar] [Publisher Link]
[8] Uma Sharma, and Naranamangalam R. Jagannathan, “Magnetic Resonance Imaging (MRI) and MR Spectroscopic Methods in Understanding Breast Cancer Biology and Metabolism,” Metabolites, vol. 12, no. 4, pp. 1-23, 2022.
[CrossRef] [Google Scholar] [Publisher Link]
[9] Naranamangalam R. Jagannathan, “Potential of Magnetic Resonance (MR) Methods in Clinical Cancer Research,” Biomedical Translational Research, pp. 339-360, 2022.
[CrossRef] [Google Scholar] [Publisher Link]
[10] Saumya Prasad et al., “Optical and Magnetic Resonance Imaging Approaches for Investigating the Tumour Microenvironment: State-of-the-Art Review and Future Trends,” Nanotechnology, vol. 32, 2020.
[CrossRef] [Google Scholar] [Publisher Link]
[11] Roman Vyškovský et al., “Structural MRI-Based Schizophrenia Classification Using Autoencoders and 3D Convolutional Neural Networks in Combination with Various Pre-Processing Techniques,” Brain Sciences, vol. 12, no. 5, pp. 1-16, 2022.
[CrossRef] [Google Scholar] [Publisher Link]
[12] Firas Khader et al., “Denoising Diffusion Probabilistic Models for 3D Medical Image Generation,” Scientific Reports, vol. 13, pp. 1-12, 2023.
[CrossRef] [Google Scholar] [Publisher Link]
[13] Miao Yu et al., “RIRGAN: An End-to-End Lightweight Multi-Task Learning Method for Brain MRI Super-Resolution and Denoising,” Computers in Biology and Medicine, vol. 167, 2023.
[CrossRef] [Google Scholar] [Publisher Link]
[14] Antanas Kascenas et al., “The Role of Noise in Denoising Models for Anomaly Detection in Medical Images,” Medical Image Analysis, vol. 90, 2023.
[CrossRef] [Google Scholar] [Publisher Link]
[15] Muhammad Fathi Mohd Zain, Wan Mahani Hafizah Wan Mahmud, and Hong-Seng Gan, “Preliminary Analysis on the Effect of Different Denoising Techniques towards Texture Features of MRI Images of Alzheimer’s Disease,” Journal of Advanced Research in Applied Sciences and Engineering Technology, vol. 31, no. 2, pp. 234-244, 2023.
[CrossRef] [Google Scholar] [Publisher Link]
[16] Matthew Cieslak et al., “Diffusion MRI Head Motion Correction Methods are Highly Accurate but Impacted by Denoising and Sampling Scheme,” Human Brain Mapping, vol. 45, no. 2, 2024.
[CrossRef] [Google Scholar] [Publisher Link]
[17] Linmin Pei et al., “A General Skull Stripping of Multiparametric Brain MRIs Using 3D Convolutional Neural Network,” Scientific Reports, vol. 12, pp. 1-11, 2022.
[CrossRef] [Google Scholar] [Publisher Link]
[18] Qi Wang, “DISGAN: Wavelet-Informed Discriminator Guides GAN to MRI Super-Resolution with Noise Cleaning,” 2023 IEEE/CVF International Conference on Computer Vision Workshops (ICCVW), pp. 2444-2453, 2023.
[CrossRef] [Google Scholar] [Publisher Link]
[19] Darshil Mehta et al., “MRI Image Denoising Using U-Net and Image Processing Techniques,” 2022 5th International Conference on Advances in Science and Technology (ICAST), Mumbai, India, pp. 306-313, 2022.
[CrossRef] [Google Scholar] [Publisher Link]
[20] Ovidijus Grigas, Rytis Maskeliūnas, and Robertas Damaševičius, “Improving Structural MRI Preprocessing with Hybrid Transformer GANs,” Life, vol. 13, no. 9, pp. 1-22, 2023.
[CrossRef] [Google Scholar] [Publisher Link]
[21] Lenuta Pana, Simona Moldovanu, and Luminita Moraru, “Statistical Filters for Processing and Reconstruction of 3D Brain MRI,” 2021 25th International Conference on System Theory, Control and Computing (ICSTCC) Iasi, Romania, pp. 655-658, 2021.
[CrossRef] [Google Scholar] [Publisher Link]
[22] Faizad Ullah, “Brain MR Image Enhancement for Tumor Segmentation Using 3D U-Net,” Sensors, vol. 21, no. 22, pp. 1-14, 2021.
[CrossRef] [Google Scholar] [Publisher Link]
[23] Muhammad Fayaz et al., “An Efficient Methodology for Brain MRI Classification Based on DWT and Convolutional Neural Network,” Sensors, vol. 21, no. 22, pp. 1-19, 2021.
[CrossRef] [Google Scholar] [Publisher Link]
[24] Imayanmosha Wahlang, Goutam Saha, and Arnab Kumar Maji, “A Comparative Analysis on Denoising Techniques in Brain MRI and Cardiac Echo,” Proceedings of the International Conference on Computing and Communication Systems, pp. 381-391, 2021.
[CrossRef] [Google Scholar] [Publisher Link]
[25] Noorbakhsh Amiri Golilarz et al., “Adaptive Wavelet Based MRI Brain Image De-Noising,” Frontiers in Neuroscience, vol. 14, pp. 1-14, 2000.
[CrossRef] [Google Scholar] [Publisher Link]