Enhancing Fingerprint Image Resolution Using Auto-Encoder and Interpolation Techniques
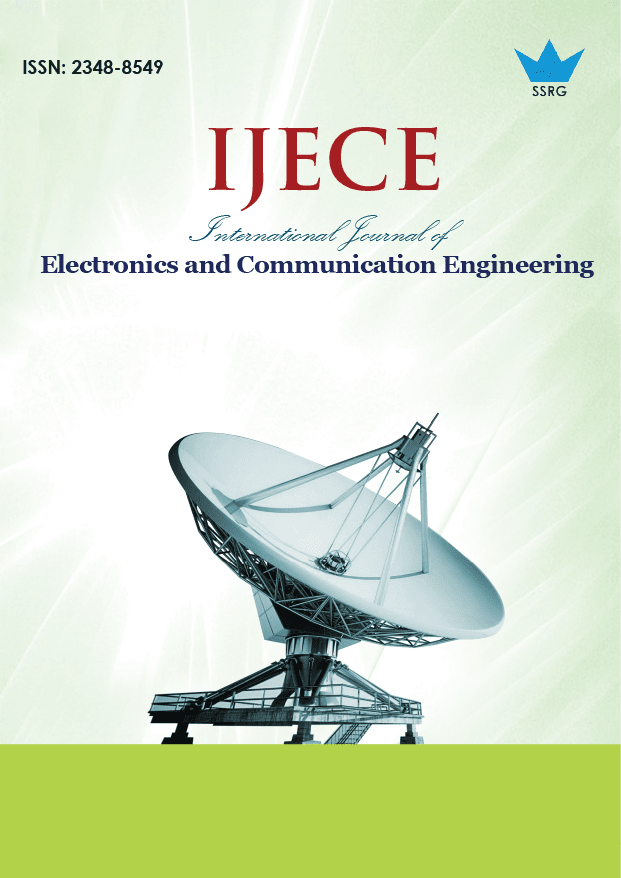
International Journal of Electronics and Communication Engineering |
© 2024 by SSRG - IJECE Journal |
Volume 11 Issue 4 |
Year of Publication : 2024 |
Authors : P.P. Lisha, V.K. Jayasree |
How to Cite?
P.P. Lisha, V.K. Jayasree, "Enhancing Fingerprint Image Resolution Using Auto-Encoder and Interpolation Techniques," SSRG International Journal of Electronics and Communication Engineering, vol. 11, no. 4, pp. 102-114, 2024. Crossref, https://doi.org/10.14445/23488549/IJECE-V11I4P111
Abstract:
Super-Resolution (SR) techniques play a vital role in enhancing the resolution of low-quality images, including fingerprint images, which are crucial for various applications such as biometric authentication and forensic analysis. However, achieving high-quality super-resolution of fingerprint images poses several challenges, including preserving fine details and minimizing artifacts. Existing methods often struggle to effectively enhance fingerprint images without sacrificing important features or introducing unwanted distortions. This study presents a novel approach for enhancing the resolution of low-resolution fingerprint images to high-resolution ones using an auto-encoder in combination with spline and bi-cubic interpolation methods. For effective analysis in a variety of applications, including satellite photography, surveillance, and medical imaging, highquality images are imperative. However, the transmission, storage, and processing of high-resolution images require substantial bandwidth, storage, and computational resources. Therefore, there is a need for computationally efficient and size-optimized resolution enhancement algorithms. The proposed method enhances fingerprint images from a size of 129 × 97 to 258 × 194. Utilizing spline and bi-cubic interpolation techniques ensures smoother curves and reduced artifacts during image up-sampling, preserving fidelity and detail. The model is trained on the FVC 2004 dataset and tested on both FVC 2004 and FVC 2002 datasets. Performance evaluation metrics such as SSIM, PSNR, and MSE yielded values of 35.14, 0.968, and 0.007, respectively. Furthermore, the identification accuracy of the proposed model, measured using the SIFT algorithm, achieved 100% on these datasets. The outcomes of the experiment show the effectiveness and superiority of the proposed approach in enhancing fingerprint image resolution, paving the way for improved accuracy and reliability in fingerprint recognition systems.
Keywords:
Fingerprint, Super Resolution, Auto encoder, Bi-cubic, Spline interpolation, Minutiae.
References:
[1] Davide Maltoni et al., Handbook of Fingerprint Recognition, 2nd ed., Springer London, 2009.
[CrossRef] [Google Scholar] [Publisher Link]
[2] Weixin Bian, Shifei Ding, and Yu Xue, “Fingerprint Image Super Resolution Using Sparse Representation with Ridge Pattern Prior by Classification Coupled Dictionaries,” IET Biometrics, vol. 6, no. 5, pp. 342-350, 2017.
[CrossRef] [Google Scholar] [Publisher Link]
[3] Patrick Schuch, Simon Schulz, and Christoph Busch, “Survey on the Impact of Fingerprint Image Enhancement,” IET Biometrics, vol. 7, no. 2, pp. 102-115, 2018.
[CrossRef] [Google Scholar] [Publisher Link]
[4] Kshitij Singh, and Gireesh Kumar Dixit, “Application of Machine Learning in Fingerprint Image Enhancement and Recognition: A Review,” Smart Moves Journal Ijoscience, vol. 7, no. 4, pp. 33-37, 2021.
[Google Scholar] [Publisher Link]
[5] Sergio Saponara, Abdussalam Elhanashi, and Qinghe Zheng, “Recreating Fingerprint Images by Convolutional Neural Network Autoencoder Architecture,” IEEE Access, vol. 9, pp. 147888-147899, 2021.
[CrossRef] [Google Scholar] [Publisher Link]
[6] Anand Deshpande, and Prashant P. Patavardhan, “Survey of Super Resolution Techniques,” ICTACT Journal on Image & Video Processing, vol. 9, no. 3, pp. 1927-1934, 2019.
[CrossRef] [Google Scholar] [Publisher Link]
[7] Sen Wang, and Yangsheng Wang, “Fingerprint Enhancement in the Singular Point Area,” IEEE Signal Processing Letters, vol. 11, no. 1, pp. 16-19, 2004.
[CrossRef] [Google Scholar] [Publisher Link]
[8] Alexandre Boucher, Phaedon C. Kyriakidis, and Collin Cronkite-Ratcliff, “Geostatistical Solutions for Super-Resolution Land Cover Mapping,” IEEE Transactions on Geoscience and Remote Sensing, vol. 46, no. 1, pp. 272-283, 2008.
[CrossRef] [Google Scholar] [Publisher Link]
[9] Kamal Nasrollahi, and Thomas B. Moeslund, “Super-Resolution: A Comprehensive Survey,” Machine Vision and Applications, vol. 25, pp. 1423-1468, 2014.
[CrossRef] [Google Scholar] [Publisher Link]
[10] Yoong Khang Ooi, and Haidi Ibrahim, “Deep Learning Algorithms for Single Image Super-Resolution: A Systematic Review,” Electronics, vol. 10, no. 7, pp. 1-33, 2021.
[CrossRef] [Google Scholar] [Publisher Link]
[11] Wenming Yang et al., “Deep Learning for Single Image Super-Resolution: A Brief Review,” IEEE Transactions on Multimedia, vol. 21, no. 12, pp. 3106-3121, 2019.
[CrossRef] [Google Scholar] [Publisher Link]
[12] Uttam U. Deshpande et al., “End-to-End Automated Latent Fingerprint Identification with Improved DCNN-FFT Enhancement,” Frontiers in Robotics and AI, vol. 7, pp. 1-18, 2020.
[CrossRef] [Google Scholar] [Publisher Link]
[13] Jeremy Vonderfecht, and Feng Liu, “Fingerprints of Super Resolution Networks,” Transactions on Machine Learning Research, pp. 1- 14, 2022.
[Google Scholar] [Publisher Link]
[14] Juan E. Tapia et al., “Selfie Periocular Verification Using an Efficient Super-Resolution Approach,” IEEE Access, vol. 10, pp. 67573- 67589, 2022.
[CrossRef] [Google Scholar] [Publisher Link]
[15] Young Won Lee, Jung Soo Kim, and Kang Ryoung Park, “Ocular Biometrics with Low-Resolution Images Based on Ocular Super-Resolution CycleGAN,” Mathematics, vol. 10, no. 20, pp. 1-30, 2022.
[CrossRef] [Google Scholar] [Publisher Link]
[16] M.B. Shahbakhsh, and H. Hassanpour, “Empowering Face Recognition Methods Using a GAN-Based Single Image Super-Resolution Network,” International Journal of Engineering, vol. 35, no. 10, pp. 1858-1866, 2022.
[CrossRef] [Google Scholar] [Publisher Link]
[17] Wazir Muhammad et al., “Multi-Path Deep CNN with Residual Inception Network for Single Image Super-Resolution,” Electronics, vol. 10, no. 16, pp. 1-25, 2021. [CrossRef] [Google Scholar] [Publisher Link]
[18] Chi-En Huang et al., “Super-Resolution Generative Adversarial Network Based on the Dual Dimension Attention Mechanism for Biometric Image Super-Resolution,” Sensors, vol. 21, no. 23, pp. 1-22, 2021.
[CrossRef] [Google Scholar] [Publisher Link]
[19] Vijay Anand, and Vivek Kanhangad, “PoreNet: CNN-Based Pore Descriptor for High-Resolution Fingerprint Recognition,” IEEE Sensors Journal, vol. 20, no. 16, pp. 9305-9313, 2020.
[CrossRef] [Google Scholar] [Publisher Link]
[20] Ngoc Tuyen Le et al., “Fingerprint Enhancement Based on Tensor of Wavelet Subbands for Classification,” IEEE Access, vol. 8, pp. 6602-6615, 2020.
[CrossRef] [Google Scholar] [Publisher Link]
[21] Dogus Karabulut et al., “Cycle-Consistent Generative Adversarial Neural Networks Based Low Quality Fingerprint Enhancement,” Multimedia Tools and Applications, vol. 79, pp. 18569-18589, 2020.
[CrossRef] [Google Scholar] [Publisher Link]
[22] FVC2002, Fingerprint Verification Competition, Databases, 2002. [Online]. Available: http://bias.csr.unibo.it/fvc2002/databases.asp
[23] FVC2004, Fingerprint Verification Competition, Databases, [Online]. Available: http://bias.csr.unibo.it/fvc2004/databases.asp
[24] K.T. Gribbon, and D.G. Bailey, “A Novel Approach to Real-Time Bilinear Interpolation,” Proceedings. DELTA 2004. Second IEEE International Workshop on Electronic Design, Test and Applications, Perth, Australia, pp. 126-131, 2004.
[CrossRef] [Google Scholar] [Publisher Link]
[25] https://www.nist.gov/services-resources/software/org/ [26] Antonio Gulli, Amita Kapoor, and Sujit Pal, Deep Learning with TensorFlow 2 and Keras: Regression, ConvNets, GANs, RNNs, NLP, and more with TensorFlow 2 and the Keras API, 2nd ed., Packt Publishing, Birmingham, 2019.
[Google Scholar] [Publisher Link]
[27] A.T. Gowthami, and H.R. Mamatha, “Fingerprint Recognition Using Zone Based Linear Binary Patterns,” Procedia Computer Science, vol. 58, pp. 552-557, 2015.
[CrossRef] [Google Scholar] [Publisher Link]
[28] Andreea-Monica Dincă Lăzărescu, Simona Moldovanu, and Luminita Moraru, “A Fingerprint Matching Algorithm Using the Combination of Edge Features and Convolution Neural Networks,” Inventions, vol. 7, no. 2, pp. 1-13, 2022.
[CrossRef] [Google Scholar] [Publisher Link]
[29] Rashmi Gupta et al., “Fingerprint Image Enhancement and Reconstruction Using the Orientation and Phase Reconstruction,” Information Sciences, vol. 530, pp. 201-218. 2020.
[CrossRef] [Google Scholar] [Publisher Link]