SmartGuard FusionNet: A YOLOv5-Based Multi-Sensor Data Fusion Framework for Superior Weapon Detection in Smart Surveillance Systems
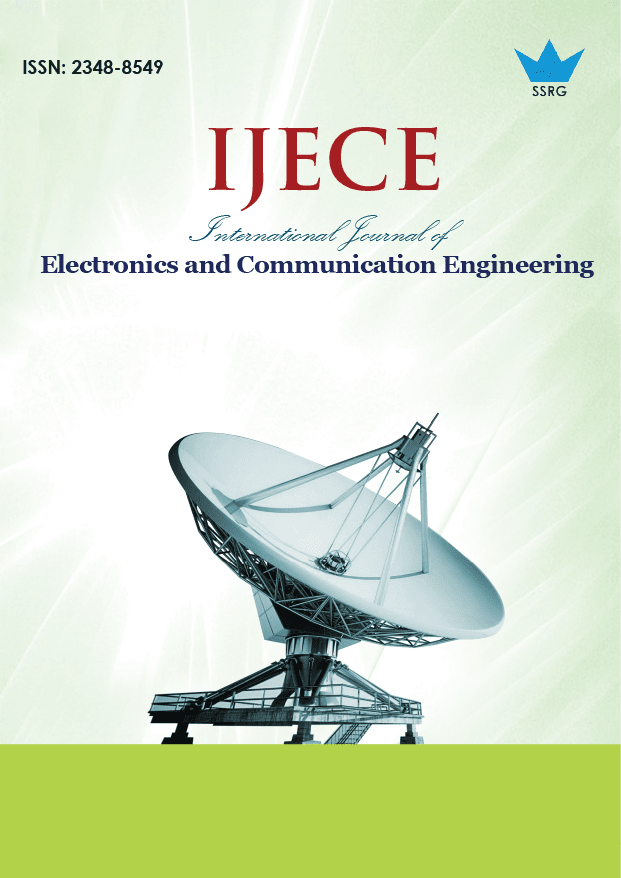
International Journal of Electronics and Communication Engineering |
© 2024 by SSRG - IJECE Journal |
Volume 11 Issue 5 |
Year of Publication : 2024 |
Authors : S. Vinay Kumar, V. Suresh, K. Ashfaq Ahmed, G. K. Nagaraju |
How to Cite?
S. Vinay Kumar, V. Suresh, K. Ashfaq Ahmed, G. K. Nagaraju, "SmartGuard FusionNet: A YOLOv5-Based Multi-Sensor Data Fusion Framework for Superior Weapon Detection in Smart Surveillance Systems," SSRG International Journal of Electronics and Communication Engineering, vol. 11, no. 5, pp. 1-17, 2024. Crossref, https://doi.org/10.14445/23488549/IJECE-V11I5P101
Abstract:
In this paper, we endeavor to substantially advance weapon detection in smart surveillance systems by synergizing the YOLOv5 object detection algorithm with a cutting-edge multi-sensor data fusion framework. This innovative integration aims to harness the precision and speed of YOLOv5, enriched by the depth and breadth of data from multiple sensors, including visual, infrared (IR), and thermal, to adeptly identify weapons in a variety of conditions. Through the development and rigorous evaluation of SmartGuard FusionNet, our approach has been quantitatively assessed across scenarios characterized by optimal lighting, low light, high traffic, and diverse backgrounds. Results demonstrate SmartGuard FusionNet’s superior performance, achieving an accuracy of up to 94.2%, precision of 96.8%, recall of 95.6%, and a detection speed of 43 frames per second, significantly surpassing existing surveillance models. These findings not only highlight the framework’s unparalleled detection accuracy and efficiency but also its robust adaptability across different environmental challenges. Conclusively, the integration of YOLOv5 with multi-sensor data fusion represents a significant leap forward in smart surveillance technology, offering enhanced capabilities for public safety and security in increasingly complex urban environments.
Keywords:
Weapon detection, Smart surveillance, YOLOv5, Multi-sensor data fusion, Robust performance, Real-world scenarios.
References:
[1] Safa Ben Atitallah et al., “Leveraging Deep Learning and IoT Big Data Analytics to Support the Smart Cities Development: Review and Future Directions,” Computer Science Review, vol. 38, 2020.
[CrossRef] [Google Scholar] [Publisher Link]
[2] Li Sun et al., “An Intelligent System for High-Density Small Target Pest Identification and Infestation Level Determination Based on an Improved YOLOv5 model,” Expert Systems with Applications, vol. 239, 2024.
[CrossRef] [Google Scholar] [Publisher Link]
[3] Paraskevi Theodorou, Kleomenis Tsiligkos, and Apostolos Meliones, “Multi-Sensor Data Fusion Solutions for Blind and Visually Impaired: Research and Commercial Navigation Applications for Indoor and Outdoor Spaces,” Sensors, vol. 23, no. 12, pp. 1-29, 2023.
[CrossRef] [Google Scholar] [Publisher Link]
[4] Vishva Payghode et al., “Object Detection and Activity Recognition in Video Surveillance Using Neural Networks,” International Journal of Web Information Systems, vol. 19, no. 3/4, pp. 123-138, 2023.
[CrossRef] [Google Scholar] [Publisher Link]
[5] John Philip Bhimavarapu et al., “Convolutional Neural Network-Based Object Detection System for Video Surveillance Application,” Concurrency and Computation: Practice and Experience, vol. 35, no. 3, 2022.
[CrossRef] [Google Scholar] [Publisher Link]
[6] Nikita Mohod, Prateek Agrawal, and Vishu Madaan, “YOLOv4 vs YOLOv5: Object Detection on Surveillance Videos,” International Conference on Advanced Network Technologies and Intelligent Computing, Varanasi, India, pp. 654-665, 2023.
[CrossRef] [Google Scholar] [Publisher Link]
[7] Malik Javed Akhtar et al., “A Robust Framework for Object Detection in a Traffic Surveillance System,” Electronics, vol. 11, no. 21, pp. 1-20, 2022.
[CrossRef] [Google Scholar] [Publisher Link]
[8] Ali Abbasi et al., “Sensor Fusion Approach for Multiple Human Motion Detection for Indoor Surveillance Use-Case,” Sensors, vol. 23, no. 8, pp. 1-12, 2023.
[CrossRef] [Google Scholar] [Publisher Link]
[9] Luis Patino et al., “Fusion of Heterogenous Sensor Data in Border Surveillance,” Sensors, vol. 22, no. 19, pp. 1-17, 2022.
[CrossRef] [Google Scholar] [Publisher Link]
[10] Jingxiang Qu et al., “Edge Computing-Enabled Multi-Sensor Data Fusion for Intelligent Surveillance in Maritime Transportation Systems,” 2022 IEEE International Conference on Dependable, Autonomic and Secure Computing, International Conference on Pervasive Intelligence and Computing, International Conference on Cloud and Big Data Computing, International Conference on Cyber Science and Technology Congress (DASC/PiCom/CBDCom/CyberSciTech), Falerna, Italy, pp. 1-8, 2022.
[CrossRef] [Google Scholar] [Publisher Link]
[11] T.M. Dhipu, Sapna, and R. Rajesh, “Deep Learning Based Multi Sensor Data Fusion for Radar and IFF,” 2022 IEEE International Conference on Electronics, Computing and Communication Technologies (CONECCT), Bangalore, India, pp. 1-6, 2022.
[CrossRef] [Google Scholar] [Publisher Link]
[12] Tsung-Yu Su, and Fang-Yie Leu, “The Design and Implementation of a Weapon Detection System Based on the YOLOv5 Object Detection Algorithm,” International Conference on Innovative Mobile and Internet Services in Ubiquitous Computing, pp. 283-293, 2023.
[CrossRef] [Google Scholar] [Publisher Link]
[13] Muhammad Ekmal Eiman Quyyum, and Mohd Haris Lye Abdullah, “Weapon Detection in Surveillance Videos Using Deep Neural Networks,” Proceedings of the Multimedia University Engineering Conference, pp. 183-195, 2022.
[CrossRef] [Google Scholar] [Publisher Link]
[14] Ravpreet Kaur, and Sarbjeet Singh, “A Comprehensive Review of Object Detection with Deep Learning,” Digital Signal Processing, vol. 132, 2023.
[CrossRef] [Google Scholar] [Publisher Link]
[15] Alexander Toet, “Color Image Fusion for Concealed Weapon Detection,” Proceedings Sensors, and Command, Control, Communications, and Intelligence (C3i) Technologies for Homeland Defense and Law Enforcement II, vol. 5071, pp. 372-379, 2003.
[CrossRef] [Google Scholar] [Publisher Link]
[16] Nicholas C. Currie et al., “Imaging Sensor Fusion for Concealed Weapon Detection,” Proceedings Investigative Image Processing, vol. 2942, pp. 71-81, 1997.
[CrossRef] [Google Scholar] [Publisher Link]
[17] Gaurav Lokhande et al., “SpectraLink Cognitive Frameworks: Adaptive Fusion and Edge-Enhanced Real-Time Urban Sign Interpretation,” International Journal of Computer Engineering in Research Trends, vol. 11, no. 1, pp. 70-81, 2024.
[Publisher Link]
[18] Franklin S. Felber et al., “Fusion of Radar and Ultrasound Sensors for Concealed Weapons Detection,” Proceedings Signal Processing, Sensor Fusion, and Target Recognition V, vol. 2755, pp. 514-521, 1996.
[CrossRef] [Google Scholar] [Publisher Link]
[19] S. Veerabuthiran, and A.K. Razdan, “LIDAR for Detection of Chemical and Biological Warfare Agents,” Defence Science Journal, vol. 61, no. 3, pp. 241-250, 2011.
[CrossRef] [Google Scholar] [Publisher Link]
[20] M. Bhavsingh, and S. Jan Reddy, “Enhancing Safety and Security: Real-Time Weapon Detection in CCTV Footage Using YOLOv7,” International Journal of Computer Engineering in Research Trends, vol. 10, no. 6, pp. 1-8, 2023.
[CrossRef] [Publisher Link]
[21] F.S. Ishaq et al., “Evaluation of Industrial Based Object Detection Method Using Artificial Neural Network,” International Journal of Computer Engineering in Research Trends, vol. 5, no. 2, pp. 50-55, 2018.
[Google Scholar] [Publisher Link]
[22] M. Bhavsingh et al., “Integrating GAN-Based Image Enhancement with YOLOv5 Object Detection for Accurate Vehicle Number Plate Analysis,” International Journal of Computer Engineering in Research Trends, vol. 10, no. 6, pp. 9-14, 2023.
[CrossRef] [Publisher Link]
[23] Govindraj Chittapur, S. Murali, and Basavaraj S. Anami, “Forensic Approach for Object Elimination and Frame Replication Detection Using Noise Based Gaussian Classifier,” International Journal of Computer Engineering in Research Trends, vol. 7, no. 3, pp. 1-5, 2020.
[Google Scholar] [Publisher Link]
[24] Alexey Bochkovskiy, Chien-Yao Wang, and Hong-Yuan Mark Liao, “YOLOv4: Optimal Speed and Accuracy of Object Detection,” Arxiv, pp. 1-17, 2020.
[CrossRef] [Google Scholar] [Publisher Link]
[25] Mingxing Tan, Ruoming Pang, and Quoc V. Le, “EfficientDet: Scalable and Efficient Object Detection,” 2020 IEEE/CVF Conference on Computer Vision and Pattern Recognition, Seattle, WA, USA, pp. 10781-10790, 2020.
[CrossRef] [Google Scholar] [Publisher Link]
[26] Shaoqing Ren et al., “Faster R-CNN: Towards Real-Time Object Detection with Region Proposal Networks,” IEEE Transactions on Pattern Analysis and Machine Intelligence, vol. 39, no. 6, pp. 1137-1149, 2017.
[CrossRef] [Google Scholar] [Publisher Link]
[27] Wei Liu et al., “SSD: Single Shot Multibox Detector,” European Conference on Computer Vision, Amsterdam, Netherlands, vol. 9905, pp. 21-37, 2016.
[CrossRef] [Google Scholar] [Publisher Link]
[28] Kaiming He et al., “Mask R-CNN,” IEEE Transactions on Pattern Analysis and Machine Intelligence, vol. 42, no. 2, pp. 386-397, 2020.
[CrossRef] [Google Scholar] [Publisher Link]