Hybrid Whale Optimisation with Improved Pooling Based Recurrent Neural Network to Predict the Heart Murmur at Earlier Stage
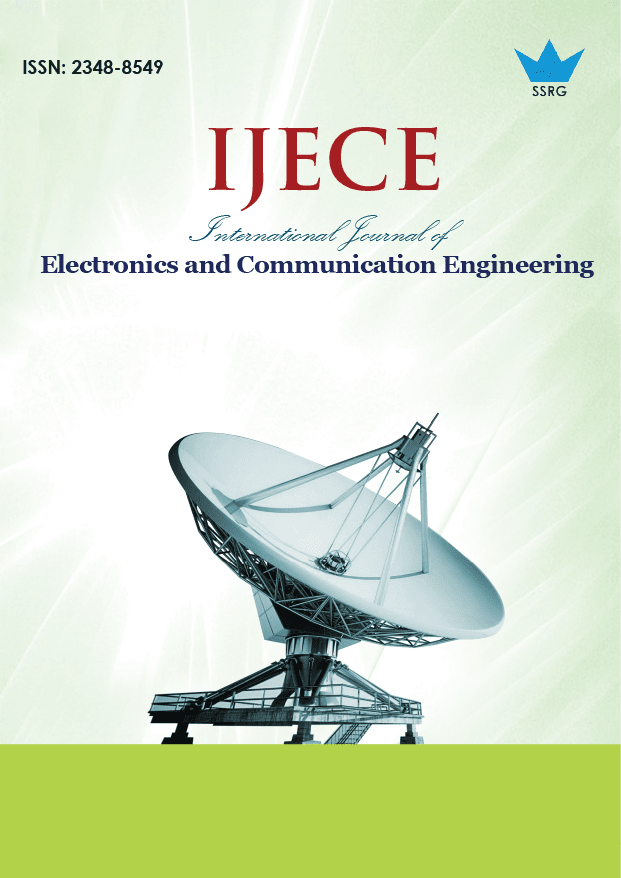
International Journal of Electronics and Communication Engineering |
© 2024 by SSRG - IJECE Journal |
Volume 11 Issue 5 |
Year of Publication : 2024 |
Authors : M. Hemalatha, Zayaraz Godandapani |
How to Cite?
M. Hemalatha, Zayaraz Godandapani, "Hybrid Whale Optimisation with Improved Pooling Based Recurrent Neural Network to Predict the Heart Murmur at Earlier Stage," SSRG International Journal of Electronics and Communication Engineering, vol. 11, no. 5, pp. 18-26, 2024. Crossref, https://doi.org/10.14445/23488549/IJECE-V11I5P102
Abstract:
Heart murmurs are abnormal sounds produced during cardiac cycles that can indicate underlying cardiovascular disorders. Early detection of heart murmurs is crucial for timely intervention and enhanced patient results. This paper proposed a hybrid method for early stage heart murmur prediction using a combination of the Whale Optimization Algorithm (WOA) and an Improved pooling-based Recurrent Neural Network (IRNN). The improved pooling mechanism enhances the RNN's ability to capture relevant features from the input data while reducing the computational complexity. Our proposed Model (WOA-IRNN) is able to learn the long-term reliance on the heart sound signals and identify the subtle patterns that are indicative of heart murmur. Experimental results demonstrate that the Whale Optimization with improved pooling-based RNN outperforms existing methods. We evaluated the WOA-IRNN method on a dataset of heart sound signals and realised a high accuracy of 98.9% in predicting heart murmur. The consequences illustrate that our system significantly progresses the prediction accuracy, demonstrating its potential for early stage heart murmur detection.
Keywords:
Heart murmurs, Recurrent Neural Network, Whale Optimization Algorithm, Early detection, and classification.
References:
[1] Yujia Xu et al., “Hierarchical Multi-Scale Convolutional Network for Murmurs Detection on PCG Signals,” 2022 Computing in Cardiology (CinC), Tampere, Finland, pp. 1-4, 2022.
[CrossRef] [Google Scholar] [Publisher Link]
[2] Matthew A. Reyna et al., “Heart Murmur Detection from Phonocardiogram Recordings: The George B. Moody PhysioNet Challenge 2022,” PLOS Digital Health, vol. 2, no. 9, pp. 1-22, 2022.
[CrossRef] [Google Scholar] [Publisher Link]
[3] Maurice Rohr et al., “Two-Stage Multitask-Learner for PCG Murmur Location Detection,” 2022 Computing in Cardiology (CinC), Tampere, Finland, pp. 1-4, 2022.
[CrossRef] [Google Scholar] [Publisher Link]
[4] Jiaming Wang et al., “Intelligent Diagnosis of Heart Murmurs in Children with Congenital Heart Disease,” Journal of Healthcare Engineering, vol. 2020, pp. 1-9, 2020.
[CrossRef] [Google Scholar] [Publisher Link]
[5] Shahnawaz Alam, Rohan Banerjee, and Soma Bandyopadhyay, “Murmur Detection Using Parallel Recurrent & Convolutional Neural Networks,” arXiv, 2018.
[CrossRef] [Google Scholar] [Publisher Link]
[6] Yunbo Wang et al., “PredRNN: A Recurrent Neural Network for Spatiotemporal Predictive Learning,” IEEE Transactions on Pattern Analysis and Machine Intelligence, vol. 45, no. 2, pp. 2208-2225, 2023.
[CrossRef] [Google Scholar] [Publisher Link]
[7] Omer Deperlioglu et al., “Diagnosis of Heart Diseases by a Secure Internet of Health Things System Based on Autoencoder Deep Neural Network,” Computer Communications, vol. 162, pp. 31-50, 2020.
[CrossRef] [Google Scholar] [Publisher Link]
[8] Mohanad Alkhodari, and Luay Fraiwan, “Convolutional and Recurrent Neural Networks for the Detection of Valvular Heart Diseases in Phonocardiogram Recordings,” Computer Methods and Programs in Biomedicine, vol. 200, 2021.
[CrossRef] [Google Scholar] [Publisher Link]
[9] Sanjoy Chakraborty et al., “A Novel Improved Whale Optimization Algorithm to Solve Numerical Optimization and Real-World Applications,” Artificial Intelligence Review, vol. 55, pp. 4605-4716, 2022.
[CrossRef] [Google Scholar] [Publisher Link]
[10] Rohit Kundu et al., “AltWOA: Altruistic Whale Optimization Algorithm for Feature Selection on Microarray Datasets,” Computers in Biology and Medicine, vol. 144, 2022.
[CrossRef] [Google Scholar] [Publisher Link]
[11] Andrew McDonald, Mark J.F. Gales, and Anurag Agarwal, “Detection of Heart Murmurs in Phonocardiograms with Parallel Hidden Semi-Markov Models,” 2022 Computing in Cardiology (CinC), Tampere, Finland, pp. 1-4, 2022.
[CrossRef] [Google Scholar] [Publisher Link]
[12] F. Mokeddem, F. Meziani, and S.M. Debbal, “Study of Murmurs and their Impact on the Heart Variability,” International Journal of Medical Engineering and Informatics, vol. 12, no. 3, pp. 291-301, 2020.
[CrossRef] [Google Scholar] [Publisher Link]
[13] Vijendra Singh, Vijayan K. Asari, and Rajkumar Rajasekaran, “A Deep Neural Network for Early Detection and Prediction of Chronic Kidney Disease,” Diagnostics, vol. 12, no. 1, pp. 1-22, 2022.
[CrossRef] [Google Scholar] [Publisher Link]
[14] Sara Khaled, “Analysis of Training Optimization Algorithms in the NARX Neural Network for Classification of Heart Sound Signals,” International Journal of Scientific and Engineering Research, vol. 13, no. 2, pp. 382-390, 2022.
[Google Scholar] [Publisher Link]
[15] Hui Wang et al., “An Automatic Approach for Heart Failure Typing Based on Heart Sounds and Convolutional Recurrent Neural Networks,” Physical and Engineering Sciences in Medicine, vol. 45, no. 2, pp. 475-485, 2022.
[CrossRef] [Google Scholar] [Publisher Link]
[16] K. Antony Kumar, and M.J. Carmel Mary Belinda, “A Multi-Layer Acoustic Neural Network-Based Intelligent Early Diagnosis System for Rheumatic Heart Disease,” International Journal of Image and Graphics, vol. 24, no. 1, 2024.
[CrossRef] [Google Scholar] [Publisher Link]
[17] Ahmed Patwa, Muhammad Mahboob Ur Rahman, and Tareq Y. Al-Naffouri, “Heart Murmur and Abnormal PCG Detection via Wavelet Scattering Transform & a 1D-CNN,” arXiv, 2023.
[CrossRef] [Google Scholar] [Publisher Link]
[18] Yasser Zeinali, and Seyed Taghi Akhavan Niaki, “Heart Sound Classification Using Signal Processing and Machine Learning Algorithms,” Machine Learning with Applications, vol. 7, 2022.
[CrossRef] [Google Scholar] [Publisher Link]
[19] Venkatesh Vakamullu et al., “Convolutional Neural Network Based Heart Sounds Recognition on Edge Computing Platform,” 2022 IEEE International Instrumentation and Measurement Technology Conference (I2MTC), Ottawa, ON, Canada, pp. 1-6, 2022.
[CrossRef] [Google Scholar] [Publisher Link]
[20] Curt G. DeGroff et al., “Artificial Neural Network–Based Method of Screening Heart Murmurs In Children,” Circulation, vol. 103, no. 22, pp. 2711-2716, 2001.
[CrossRef] [Google Scholar] [Publisher Link]
[21] Jou-Kou Wang et al., “Automatic Recognition of Murmurs of Ventricular Septal Defect Using Convolutional Recurrent Neural Networks with Temporal Attentive Pooling,” Scientific Reports, vol. 10, no. 1, 2020.
[CrossRef] [Google Scholar] [Publisher Link]
[22] Aadhav Prabu, “SmartScope: An AI-Powered Digital Auscultation Device to Detect Cardiopulmonary Diseases,” TechRxiv, Authorea Preprint, 2021. [CrossRef] [Google Scholar] [Publisher Link]
[23] Ali A. Samir et al., “Evolutionary Algorithm-Based Convolutional Neural Network for Predicting Heart Diseases,” Computers & Industrial Engineering, vol. 161, 2021.
[CrossRef] [Google Scholar] [Publisher Link]
[24] Xin Zhou et al., “A Novel 1-D Densely Connected Feature Selection Convolutional Neural Network for Heart Sounds Classification,” Annals of Translational Medicine, vol. 9, no. 24, 2021.
[CrossRef] [Google Scholar] [Publisher Link]
[25] Yi He et al., “Research on Segmentation and Classification of Heart Sound Signals Based on Deep Learning,” Applied Sciences, vol. 11, no. 2, pp. 1-15, 2021.
[CrossRef] [Google Scholar] [Publisher Link]