EFSM-MLB: An Ensemble Feature Selection Model for Better Outcome Prediction in Major League Baseball Using Filter and Embedded Methods
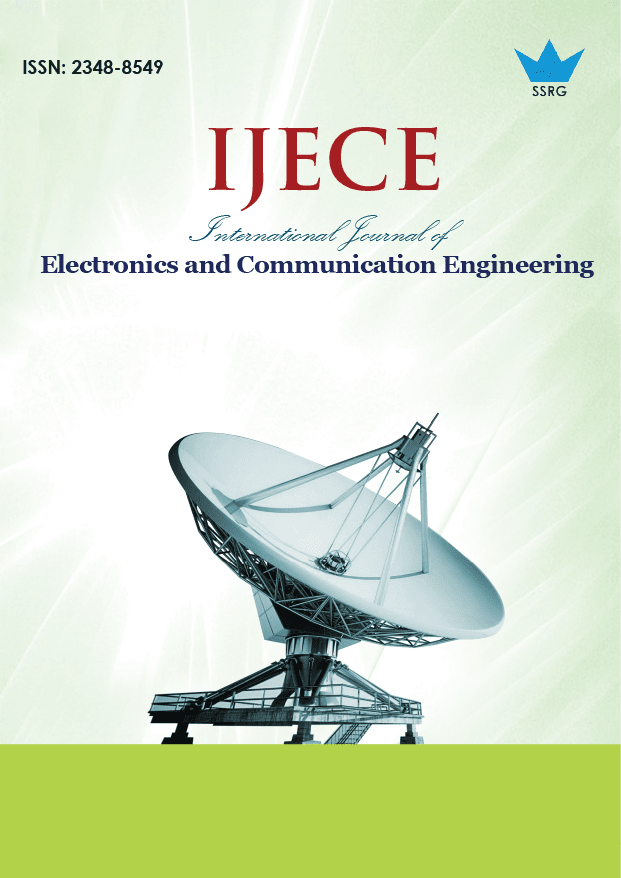
International Journal of Electronics and Communication Engineering |
© 2024 by SSRG - IJECE Journal |
Volume 11 Issue 5 |
Year of Publication : 2024 |
Authors : Deepak Pandey, Rajeev Gupta |
How to Cite?
Deepak Pandey, Rajeev Gupta, "EFSM-MLB: An Ensemble Feature Selection Model for Better Outcome Prediction in Major League Baseball Using Filter and Embedded Methods," SSRG International Journal of Electronics and Communication Engineering, vol. 11, no. 5, pp. 45-58, 2024. Crossref, https://doi.org/10.14445/23488549/IJECE-V11I5P105
Abstract:
Major League Baseball (MLB) stands as one of the most globally renowned and widely played tournaments at the international level in the realm of sports research. Predicting the key input variables of a match in MLB based tournament is very challenging. The selection process involves choosing which variables are more important for match prediction, as teams often use Sabermetrics in feature selection for an accurate selection of players. The current study aims to identify the major input variables that influence MLB team winnings. The authors of this research suggested an ensemble feature selection model for a better and more accurate outcome of a match. The proposed mechanism is tested on an open-access dataset of major leagues from 2005 to 2023, which is freely available on Baseball-Reference. The authors implement the proposed model on a set of sixty different offensive and defensive game features. Results obtained from deep analysis and implementation using linear regression and Correlation indicate a positive or negative association with win percentage. Here, the suggested model ranks all MLB variables from highly correlated to lesser correlated variables according to their association with win percentage. Pitching characteristics are found to be more important for forecasting match outcomes in favour of winners during this feature selection process. Furthermore, it has been discovered that run differential is a major factor in match prediction.
Keywords:
Correlation, Feature selection, Machine learning, Major League Baseball, Regression, Run difference.
References:
[1] Christina Gough, Major League Baseball Total League Revenue from 2001 to 2022, Statista, 2023. [Online]. Available: https://www.statista.com/statistics/193466/total-league-revenue-of-the-mlb-since-2005/
[2] N. Kwak, and Chong-Ho Choi, “Input Feature Selection for Classification Problems,” IEEE Transactions on Neural Networks, vol. 13, no. 1, pp. 143-159, 2002.
[CrossRef] [Google Scholar] [Publisher Link]
[3] Jung-Yi Lin et al., “Classifier Design with Feature Selection and Feature Extraction Using Layered Genetic Programming,” Expert Systems with Applications, vol. 34, no. 2, pp. 1384-1393, 2008.
[CrossRef] [Google Scholar] [Publisher Link]
[4] Silvia Cateni, Valentina Colla, and Marco Vannucci, “Variable Selection through Genetic Algorithms for Classification Purposes,” Proceedings of the 10th IASTED International Conference on Artificial Intelligence and Applications, pp. 1-6, 2010.
[CrossRef] [Google Scholar] [Publisher Link]
[5] Kenji Kira, and Larry A. Rendell, “A Practical Approach to Feature Selection,” Machine Learning Proceedings 1992, pp. 249-256, 1992.
[CrossRef] [Google Scholar] [Publisher Link]
[6] Robert May, Graeme Dandy, and Holger Maier, Review of Input Variable Selection Methods for Artificial Neural Networks, Artificial Neural Network - Methodological Advances and Biomedical Applications, IntechOpen, pp. 1-28, 2011.
[CrossRef] [Google Scholar] [Publisher Link]
[7] Huanzhang Fu et al., “Image Categorization Using ESFS: A New Embedded Feature Selection Method Based on SFS,” Advanced Concepts for Intelligent Vision Systems, Bordeaux, France, pp. 288-299, 2009.
[CrossRef] [Google Scholar] [Publisher Link]
[8] Philip Beneventano, Paul D. Berger, and Bruce D. Weinberg, “Predicting Run Production and Run Prevention in Baseball: The Impact of Sabermetrics,” International Journal of Business, Humanities and Technology, vol. 2, no. 4, pp. 67-75, 2012.
[Google Scholar] [Publisher Link]
[9] Girish Chandrashekar, and Ferat Sahin, “A Survey on Feature Selection Methods,” Computers and Electrical Engineering, vol. 40, no. 1, pp. 16-28, 2014.
[CrossRef] [Google Scholar] [Publisher Link]
[10] Shu-Fen Li, Mei-Ling Huang, and Yun-Zhi Li, “Exploring and Selecting Features to Predict the Next Outcomes of MLB Games,” Entropy, vol. 24, no. 2, pp. 1-17, 2022.
[CrossRef] [Google Scholar] [Publisher Link]
[11] Mei-Ling Huang, and Yun-Zhi Li, “Use of Machine Learning and Deep Learning to Predict the Outcomes of Major League Baseball Matches,” Applied Sciences, vol. 11, no. 10, pp. 1-22, 2021.
[CrossRef] [Google Scholar] [Publisher Link]
[12] Tim Elfrink, and Sandjai Bhulai, “Predicting the Outcomes of MLB Games with a Machine Learning Approach,” Computer Science, 2018.
[Google Scholar]
[13] Randy Jia, Chris Wong, and David Zeng, “Predicting the Major League Baseball Season,” CS 229 Machine Learning Final Projects, Autumn, pp. 1-5, 2013.
[Google Scholar] [Publisher Link]
[14] Chi-Wen Chen, “Construction of the Winner Predictive Model in Major League Baseball Games: Use of the Artificial Neural Networks,” College Sports Journal, vol. 16, no. 2, pp. 167-181, 2014.
[Google Scholar]
[15] C. Soto Valero, “Predicting Win-Loss Outcomes in MLB Regular Season Games – A Comparative Study Using Data Mining Methods,” International Journal of Computer Science in Sport, vol. 15, no. 2, pp. 91-112, 2016.
[CrossRef] [Google Scholar] [Publisher Link]
[16] Krzysztof TrawiĆski, “A Fuzzy Classification System for Prediction of the Results of the Basketball Games,” International Conference on Fuzzy Systems, Barcelona, Spain, pp. 1-7, 2010.
[CrossRef] [Google Scholar] [Publisher Link]
[17] Blakeley B. McShane et al., “A Hierarchical Bayesian Variable Selection Approach to Major League Baseball Hitting Metrics,” Journal of Quantitative Analysis in Sports, vol. 7, no. 4, 2011.
[CrossRef] [Google Scholar] [Publisher Link]
[18] Silvia Cateni, Valentina Colla, and Marco Vannucci, “Improving the Stability of the Variable Selection with Small Datasets in Classification and Regression Tasks,” Neural Process Letter, vol. 55, no. 5, pp. 5331-5356, 2023.
[CrossRef] [Google Scholar] [Publisher Link]