Design and Development of Web Application Using AI Based Sensors for Improving Air Quality Index
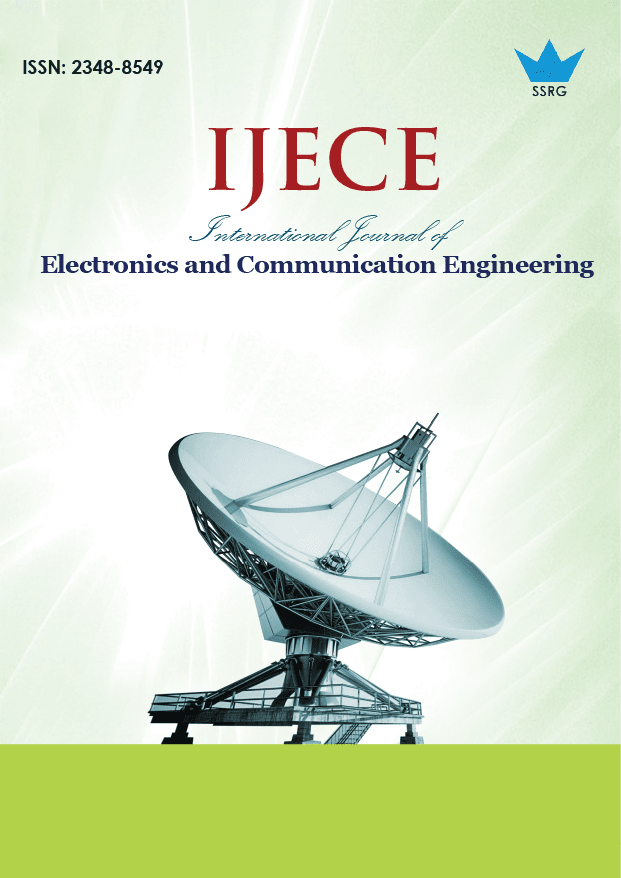
International Journal of Electronics and Communication Engineering |
© 2024 by SSRG - IJECE Journal |
Volume 11 Issue 5 |
Year of Publication : 2024 |
Authors : M. Suresh Babu, D. Asha Devi, A.Pranayanath Reddy, Sudeepthi Govathoti |
How to Cite?
M. Suresh Babu, D. Asha Devi, A.Pranayanath Reddy, Sudeepthi Govathoti, "Design and Development of Web Application Using AI Based Sensors for Improving Air Quality Index," SSRG International Journal of Electronics and Communication Engineering, vol. 11, no. 5, pp. 69-77, 2024. Crossref, https://doi.org/10.14445/23488549/IJECE-V11I5P107
Abstract:
This paper presents a Web-based platform for monitoring air quality that consists of a web server and the "SmartAir" Air quality sensor. This platform uses cloud computing and the Internet of Things to track air quality everywhere, at any time, through seamless connectivity and powered AI through an LTE modem. Based on IoT technology, Smart-Air was created to effectively monitor air quality and send data to a web server through LTE in real time. A microprocessor, sensors for pollutant detection, and an LTE modem make up the device. In order to monitor the quality of the air, the research equipment was created to detect the concentration of aerosol, VOC, CO, CO2, Particulate Matter, SO2, NO2, and temperature-humidity. The device was then successfully reliability tested by adhering to the Pollution Control Board's recommended protocol. In order to identify and visualise air quality in accordance with the Pollution Control Board and regulatory standards, Ubiquitous computing has also been integrated into a web server for data analysis from the device. To aid in tracking the air quality, an app was created. Consequently, authorised staff can use the web server or the application to check the air quality at any time and from anywhere. Artificial Intelligence (AI) plays a significant role in improving the Air Quality Index (AQI) through various applications and processes. The backend server stores all data which is accumulated from different sources and provides resources for further analysis of indoor air quality.
Keywords:
Web server, LTE Modem, Artificial Intelligence, Air Quality Index, Sensor.
References:
[1] Jose Antonio Moscoso-López et al., “Hourly Air Quality Index (AQI) Forecasting Using Machine Learning Methods,” 15th International Conference on Soft Computing Models in Industrial and Environmental Applications, Advances in Intelligent Systems and Computing, vol. 1268, pp. 123-132, 2020.
[CrossRef] [Google Scholar] [Publisher Link]
[2] Ma Limei, “Neural Network Stock Forecasting System Based on, Beijing University of Posts and Telecommunications,” 2005.
[3] Ai Hongfu et al., “Forecast of Fog and Haze Based on Bp Artificial Neural Network,” Computer Simulation, vol. 1, pp. 402-406, 2015.
[4] Li Fenfen, “The Model of Environmental Air Quality Forecasting and Early Warning System and the Causes of Uncertainty and Improvement,” Energy and Energy Conservation, vol. 12, pp. 103-105, 2018.
[5] Zuo Jinxin, “Design and Implementation of Atmospheric Quality Monitoring and Prediction and Early Warning Software,” vol. 8, 2018.
[6] Mauro Castelli et al., “A Machine Learning Approach to Predict Air Quality in California,” Complexity, vol. 2020, pp. 1-23, 2020.
[CrossRef] [Google Scholar] [Publisher Link]
[7] Chavi Srivastava, Shyamli Singh, and Amit Prakash Singh, “Estimation of Air Pollution in Delhi Using Machine Learning Techniques,” 2018 International Conference on Computing, Power and Communication Technologies, Greater Noida, India, pp. 304-309, 2018.
[CrossRef] [Google Scholar] [Publisher Link]
[8] Xiang Li et al., “Deep Learning Architecture for Air Quality Predictions,” Environmental Science and Pollution Research, vol. 23, pp. 22408-22417, 2016.
[CrossRef] [Google Scholar] [Publisher Link]
[9] Congcong Wen et al., “A Novel Spatiotemporal Convolutional Long Short-Term Neural Network for Air Pollution Prediction,” Science of the Total Environment, vol. 654, pp. 1091-1099, 2019.
[CrossRef] [Google Scholar] [Publisher Link]
[10] Sumanth Reddy Enigella, and Hamid Shahnasser, “Real Time Air Quality Monitoring,” 2018 10th International Conference on Knowledge and Smart Technology, Chiang Mai, Thailand, pp. 182-185, 2018.
[CrossRef] [Google Scholar] [Publisher Link]
[11] Hammad Aamer et al., “A Very Low Cost, Open, Wireless, Internet of Things (IoT) Air Quality Monitoring Platform,” 2018 15th International Conference on Smart Cities: Improving Quality of Life Using ICT & IoT, Islamabad, Pakistan, pp. 102-106, 2018.
[CrossRef] [Google Scholar] [Publisher Link]
[12] You are What You Breathe, Air Quality World. [Online]. Available: https://www.airquality.world/?utm_campaign=oxfordshireairquality&utm_medium=referral&utm_source=old-website
[13] Somansh Kumar, and Ashish Jasuja, “Air Quality Monitoring System Based on IoT Using Raspberry Pi,” 2017 International Conference on Computing, Communication and Automation, Greater Noida, India, pp. 1341-1346, 2017.
[CrossRef] [Google Scholar] [Publisher Link]