Emerging Trends in Data Science and Big Data Analytics: A Bibliometric Analysis
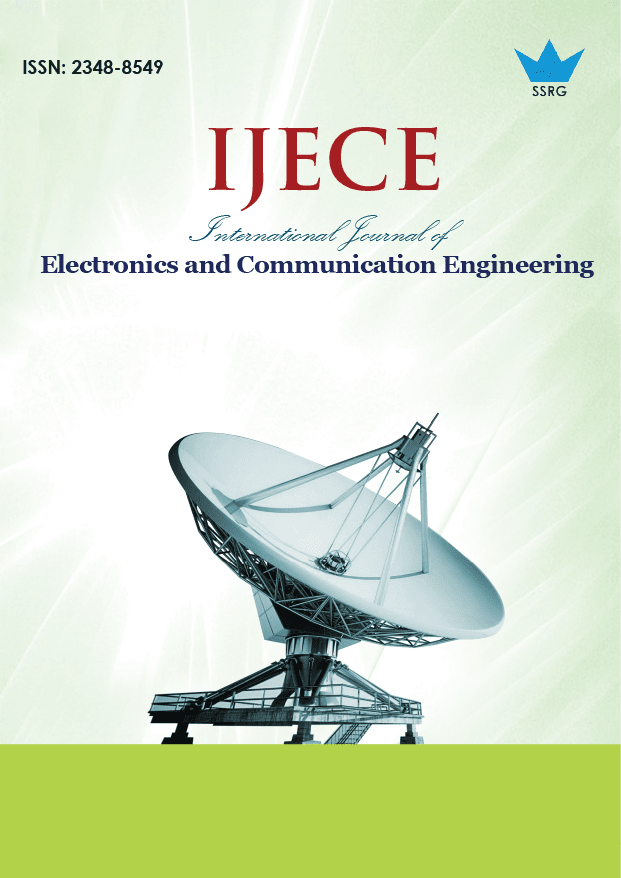
International Journal of Electronics and Communication Engineering |
© 2024 by SSRG - IJECE Journal |
Volume 11 Issue 5 |
Year of Publication : 2024 |
Authors : Abdulaziz Yasin Nageye, Abdukadir Dahir Jimale, Mohamed Omar Abdullahi, Yahye Abukar Ahmed |
How to Cite?
Abdulaziz Yasin Nageye, Abdukadir Dahir Jimale, Mohamed Omar Abdullahi, Yahye Abukar Ahmed, "Emerging Trends in Data Science and Big Data Analytics: A Bibliometric Analysis," SSRG International Journal of Electronics and Communication Engineering, vol. 11, no. 5, pp. 84-98, 2024. Crossref, https://doi.org/10.14445/23488549/IJECE-V11I5P109
Abstract:
This bibliometric analysis explores the landscape of research in Data Science and Big Data Analytics over the period from 2010 to March 2024. Leveraging advanced bibliometric techniques, including data collection from Scopus, data screening, preprocessing, and analysis using VOSviewer, Bibliometric of R package, and Microsoft Excel, this study aims to identify key trends, patterns, and dynamics within the field. The analysis encompasses document types, publication and citation trends, contributing countries, influential authors and sources, keyword co-occurrence networks, and influential affiliations. The findings provide valuable insights into the scholarly discourse, collaboration networks, and emerging research directions in Data Science and Big Data Analytics, facilitating evidence-based decision-making and fostering innovation in the field.
Keywords:
Data science, Big data analytics, Bibliometric analysis, Collaboration networks, Analytics.
References:
[1] Allison S. Theobold, “Human Centered Data Science: Ungrading in an Introductory Data Science Course,” Proceedings of the 2023 Conference on Innovation and Technology in Computer Science Education, Turku, Finland, pp. 327-333, 2023.
[CrossRef] [Google Scholar] [Publisher Link]
[2] Blend Berisha, Endrit Mëziu, and Isak Shabani, “Big Data Analytics in Cloud Computing: An Overview,” Journal of Cloud Computing, vol. 11, no. 1, pp. 1-10, 2022.
[CrossRef] [Google Scholar] [Publisher Link]
[3] Saba Ameer et al., “Comparative Analysis of Machine Learning Techniques for Predicting Air Quality in Smart Cities,” IEEE Access, vol. 7, pp. 128325-128338, 2019.
[CrossRef] [Google Scholar] [Publisher Link]
[4] Iqbal H. Sarker, “Data Science and Analytics: An Overview from Data-Driven Smart Computing, Decision-Making and Applications Perspective,” SN Computer Science, vol. 2, pp. 1-22, 2021.
[CrossRef] [Google Scholar] [Publisher Link]
[5] Ananta Charan Ojha, and Subhendu Kumar Pani, Data Science and Big Data Analytics, 1st ed., Big Data Analytics and Computing for Digital Forensic Investigations, CRC Press, pp. 1-22, 2020.
[CrossRef] [Google Scholar] [Publisher Link]
[6] Vahid Ashrafimoghari, “Big Data and Education: Using Big Data Analytics in Language Learning,” arXiv, pp. 1-12, 2022.
[CrossRef] [Google Scholar] [Publisher Link]
[7] Yasset Perez-Riverol, and Pablo Moreno, “Scalable Data Analysis in Proteomics and Metabolomics Using BioContainers and Workflows Engines,” Proteomics and Systems Biology, vol. 20, no. 9, pp. 1-12, 2020.
[CrossRef] [Google Scholar] [Publisher Link]
[8] Shraddha Surana, Yogesh Wadadekar, and Divya Oberoi, “Machine Learning for Scientific Discovery,” arXiv, pp. 1-4, 2021.
[CrossRef] [Google Scholar] [Publisher Link]
[9] S. Pasari, P. Asudani, and A. Mehta, “Machine Learning Algorithms in Geostatistical Data Analysis: Formulation and Observation,” International Conference on Advances in Earth and Environmental Studies, NIT Raipur, India, pp. 1-5, 2022.
[CrossRef] [Google Scholar] [Publisher Link]
[10] Leonidas Fegaras, “Compile-Time Query Optimization for Big Data Analytics,” Open Journal of Big Data, vol. 5, no. 1, pp. 35-61, 2019.
[Google Scholar] [Publisher Link]
[11] Tewodrose Tilahun, and Solomon Tsegaye, “The Security Challenges of Big Data Analytics: A Systematic Literature Review,” Asian Journal of Research in Computer Science, vol. 14, no. 4, pp. 184-197, 2022.
[CrossRef] [Publisher Link]
[12] Chris Brunsdon, and Alexis Comber, “Big Issues for Big Data: Challenges for Critical Spatial Data Analytics,” Journal of Spatial Information Science, no. 21, pp. 89-98, 2020.
[CrossRef] [Google Scholar] [Publisher Link]
[13] Shutao Wang et al., “Hot Topics and Frontier Evolution of Science Education Research: A Bibliometric Mapping from 2001 to 2020,” Science & Education, vol. 32, no. 3, pp. 845-869, 2022.
[CrossRef] [Google Scholar] [Publisher Link]
[14] Chien-Heng Chou, Sa Ly Ngo, and Phung Phi Tran, “Renewable Energy Integration for Sustainable Economic Growth: Insights and Challenges via Bibliometric Analysis,” Sustainability, vol. 15, no. 20, pp. 1-26, 2023.
[CrossRef] [Google Scholar] [Publisher Link]
[15] Maria Drogkoula, Konstantinos Kokkinos, and Nicholas Samaras, “A Comprehensive Survey of Machine Learning Methodologies with Emphasis in Water Resources Management,” Applied Sciences, vol. 13, no. 22, pp. 1-44, 2023.
[CrossRef] [Google Scholar] [Publisher Link]
[16] Robin Chin Roemer, and Rachel Borchardt, Meaningful Metrics, A 21st Century Librarian's Guide to Bibliometrics, Altmetrics, and Research Impact, Association of College and Research Libraries, a Division of the American Library Association, pp. 1-241, 2015.
[Google Scholar] [Publisher Link]
[17] Kaihua Chen, Yi Zhang, and Xiaolan Fu, “International Research Collaboration: An Emerging Domain of Innovation Studies?,” Research Policy, vol. 48, no. 1, pp. 149-168, 2019.
[CrossRef] [Google Scholar] [Publisher Link]
[18] Ankit Verma, and Hansraj, “Big Data Analytics Using Machine Learning Techniques for Prediction on Datasets,” Computational Intelligence and Machine Learning, vol. 4, no. 1, pp. 6-10, 2023.
[CrossRef] [Publisher Link]
[19] Rahul Reddy Nadikattu, “Research on Data Science, Data Analytics and Big Data,” International Journal of Engineering, Science and Mathematics, vol. 9, no. 5, pp. 99-105, 2020.
[CrossRef] [Google Scholar] [Publisher Link]
[20] Matthew A. Waller, and Stanley E. Fawcett, “Data Science, Predictive Analytics, and Big Data: A Revolution that will Transform Supply Chain Design and Management,” Journal of Business Logistics, vol. 34, no. 2, pp. 77-84, 2013.
[CrossRef] [Google Scholar] [Publisher Link]
[21] Radwa Elshawi et al., “Big Data Systems Meet Machine Learning Challenges: Towards Big Data Science as a Service,” Big Data Research, vol. 14, pp. 1-11, 2017.
[CrossRef] [Google Scholar] [Publisher Link]
[22] Daphne R. Raban, and Avishag Gordon, “The Evolution of Data Science and Big Data Research: A Bibliometric Analysis,” Scientometrics, vol. 122, pp. 1563-1581, 2020.
[CrossRef] [Google Scholar] [Publisher Link]
[23] Hamed Jahani, Richa Jain, and Dmitry Ivanov, “Data Science and Big Data Analytics: A Systematic Review of Methodologies Used in the Supply Chain and Logistics Research,” Annals of Operations Research, pp. 1-58, 2023.
[CrossRef] [Google Scholar] [Publisher Link]
[24] Justin Zuopeng Zhang et al., “Big Data Analytics and Machine Learning: A Retrospective Overview and Bibliometric Analysis,” Expert Systems with Applications, vol. 184, 2021.
[CrossRef] [Google Scholar] [Publisher Link]
[25] Massimo Aria, and Corrado Cuccurullo, “Bibliometrix: An R-Tool for Comprehensive Science Mapping Analysis,” Journal of Informetrics, vol. 11, no. 4, pp. 959-975, 2017.
[CrossRef] [Google Scholar] [Publisher Link]