Innovative Web-Based Tool Enhances Teen Mental Health Screening through Biomedical Circuit Analysis and Personalized Recommendations
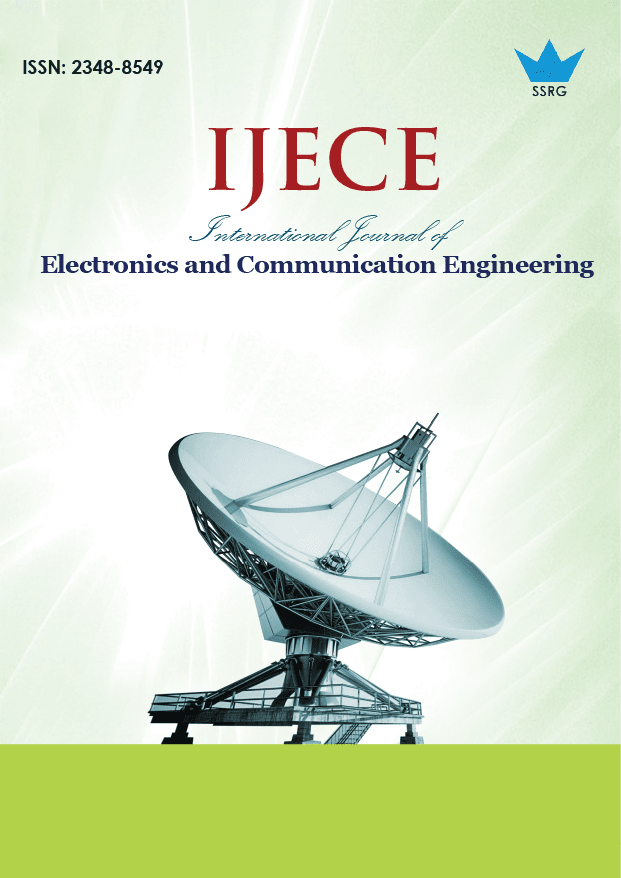
International Journal of Electronics and Communication Engineering |
© 2024 by SSRG - IJECE Journal |
Volume 11 Issue 5 |
Year of Publication : 2024 |
Authors : P. Sowmiya, S. Gunasundari, K. Sudharson, R. Vanitha, C.S. Anita |
How to Cite?
P. Sowmiya, S. Gunasundari, K. Sudharson, R. Vanitha, C.S. Anita, "Innovative Web-Based Tool Enhances Teen Mental Health Screening through Biomedical Circuit Analysis and Personalized Recommendations," SSRG International Journal of Electronics and Communication Engineering, vol. 11, no. 5, pp. 123-129, 2024. Crossref, https://doi.org/10.14445/23488549/IJECE-V11I5P113
Abstract:
This study introduces a groundbreaking web-based tool designed to improve the screening of mental health issues in teenagers. Leveraging innovative biomedical circuit analysis techniques and personalized recommendation algorithms, the system provides an advanced approach to early detection and intervention. Through intricate questionnaire modules and specialized Natural Language Processing (NLP) models, user responses are carefully analyzed to offer tailored recommendations. Built with user privacy and accessibility in mind, the application utilizes Flask technology. Calibration of the analysis ensures precise results, marking a significant leap forward in mental health diagnosis. With an impressive accuracy rate of 97%, this tool promises to make a substantial impact on addressing adolescent mental health challenges. Its innovative integration of biomedical circuit analysis and personalized recommendations represents a novel and effective approach to proactive mental health care.
Keywords:
Web-based tool, Teen mental health, Biomedical circuit analysis, Personalized recommendations, Innovative solution.
References:
[1] Aziliz Le Glaz et al., “Machine Learning and Natural Language Processing in Mental Health: Systematic Review,” Journal of Medical Internet Research, vol. 23, no. 5, pp. 1-19, 2021.
[CrossRef] [Google Scholar] [Publisher Link]
[2] S. Chancellor, and M. De Choudhury, “Methods in Predictive Techniques for Mental Health Status on Social Media: A Critical Review,” NPJ Digital Medicine, vol. 3, no. 43, pp. 1-11, 2020.
[CrossRef] [Google Scholar] [Publisher Link]
[3] Akkapon Wongkoblap, Miguel A. Vadillo, and Vasa Curcin, “Researching Mental Health Disorders in the Era of Social Media: Systematic Review,” Journal of Medical Internet Research, vol. 19, no. 6, pp. 1-36, 2017.
[CrossRef] [Google Scholar] [Publisher Link]
[4] Lin Lin et al., “Towards Automatic Depression Detection: A BiLSTM/1D CNN-Based Model,” Applied Science, vol. 10, no. 23, pp. 120, 2020.
[CrossRef] [Google Scholar] [Publisher Link]
[5] Dai Su et al., “Use of Machine Learning Approach to Predict Depression in the Elderly in China: A Longitudinal Study,” Journal of Affective Disorders, vol. 282, pp. 289-298, 2021.
[CrossRef] [Google Scholar] [Publisher Link]
[6] Sairam Balani, and Munmun De Choudhury, “Detecting and Characterizing Mental Health Related Self-Disclosure in Social Media,” Proceedings of the 33rd Annual ACM Conference Extended Abstracts on Human Factors in Computing Systems, pp. 1373-1378, 2015.
[CrossRef] [Google Scholar] [Publisher Link]
[7] Sharath Chandra Guntuku et al., “Detecting Depression and Mental Illness on Social Media: An Integrative Review,” Current Opinion in Behavioral Sciences, vol. 18, pp. 43-49, 2017.
[CrossRef] [Google Scholar] [Publisher Link]
[8] Yaakov Ophir, Christa S.C. Asterhan, and Baruch B. Schwarz, “Unfolding the Notes from the Walls: Adolescents’ Depression Manifestations on Facebook,” Computers in Human Behavior, vol. 72, pp. 96-107, 2017.
[CrossRef] [Google Scholar] [Publisher Link]
[9] Munmun De Choudhury et al., “Predicting Depression via Social Media,” Proceedings of the Seventh International AAAI Conference on Weblogs and Social Media, viol. 7, no. 1, pp. 128-137, 2013.
[CrossRef] [Google Scholar] [Publisher Link]