A Deep Learning Approach for Efficient Breast Cancer Diagnosis Using Hybrid CNN-BILSTM with Soft Attention Mechanism
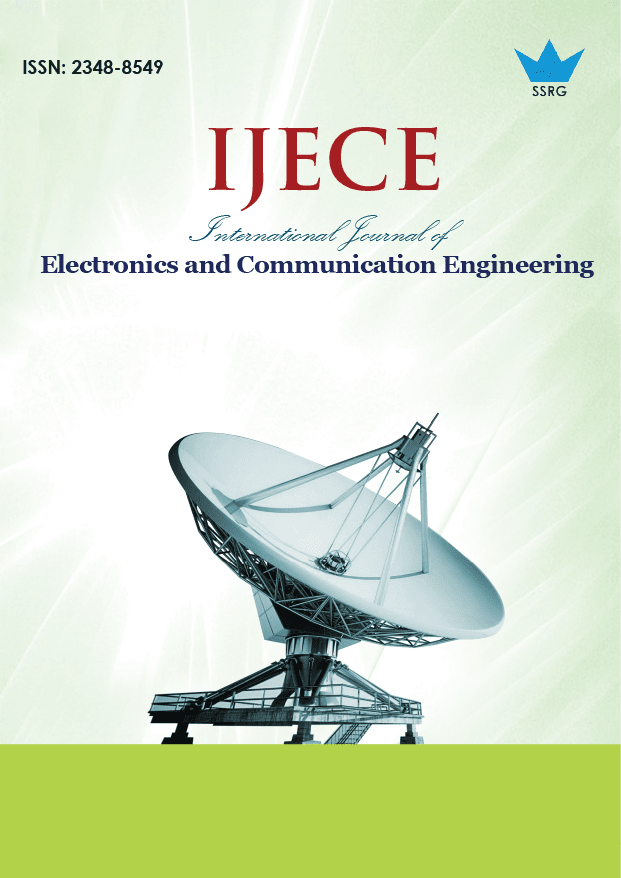
International Journal of Electronics and Communication Engineering |
© 2024 by SSRG - IJECE Journal |
Volume 11 Issue 5 |
Year of Publication : 2024 |
Authors : M. Sarathkumar, K. S. Dhanalakshmi, D. Madhivadhani, Revathi V |
How to Cite?
M. Sarathkumar, K. S. Dhanalakshmi, D. Madhivadhani, Revathi V, "A Deep Learning Approach for Efficient Breast Cancer Diagnosis Using Hybrid CNN-BILSTM with Soft Attention Mechanism," SSRG International Journal of Electronics and Communication Engineering, vol. 11, no. 5, pp. 130-138, 2024. Crossref, https://doi.org/10.14445/23488549/IJECE-V11I5P114
Abstract:
In recent years, the rising prevalence of breast cancer among women has underscored the critical importance of timely and accurate disease prediction. The ability to predict breast cancer efficiently empowers healthcare professionals with a robust decision-making system, enabling optimal treatment strategies. This study presents a sophisticated diagnostic framework, integrating various techniques from preprocessing to classification to enhance the precision and effectiveness of breast cancer detection. The proposed methodology begins with preprocessing using a Butterworth filter to enhance the quality of input data. The preprocessed output is segmented utilizing Cascaded Fuzzy C-Means segmentation. Subsequently, extracting features through the (GLCM), capturing intricate patterns and texture information crucial for discriminating between benign and malignant tissues. The classification step employs a Hybrid CNN- BiLSTM architecture augmented by a Soft Attention Mechanism. This hybrid model is designed to effectively leverage spatial hierarchies and sequential dependencies in medical images, allowing for a more comprehensive analysis of complex forms related to breast cancer. Soft Attention Mechanism enhances the interpretability of the model by assigning varying weights to different regions of the input data, emphasizing salient features crucial for exact analysis. The attention mechanism donates to the model’s ability to focus on relevant information, improving both sensitivity and specificity in breast cancer classification. The proposed methodology is validated through extensive experiments, demonstrating its superior performance compared to traditional approaches. The results highlight the possibility of this deep learning-based strategy valuable enabling healthcare professionals, facilitating precise and timely decision-making in breast cancer treatment optimization.
Keywords:
Breast cancer, Butterworth filter, Cascaded Fuzzy C-Means, Gray-Level Co-occurrence Matrix (GLCM), Hybrid CNN-BiLSTM.
References:
[1] Yi Wang et al., “Deeply-Supervised Networks with Threshold Loss for Cancer Detection in Automated Breast Ultrasound,” IEEE Transactions on Medical Imaging, vol. 39, no. 4, pp. 866-876, 2019.
[CrossRef] [Google Scholar] [Publisher Link]
[2] Usman Naseem et al., “An Automatic Detection of Breast Cancer Diagnosis and Prognosis Based on Machine Learning Using Ensemble of Classifiers,” IEEE Access, vol. 10, pp. 78242-78252, 2022.
[CrossRef] [Google Scholar] [Publisher Link]
[3] Abeer Saber et al., “A Novel Deep-Learning Model for Automatic Detection and Classification of Breast Cancer Using The TransferLearning Technique,” IEEE Access, vol. 9, pp. 71194-71209, 2021.
[CrossRef] [Google Scholar] [Publisher Link]
[4] Zexian Huang, and Daqi Chen, “A Breast Cancer Diagnosis Method Based On Vim Feature Selection and Hierarchical Clustering Random Forest Algorithm,” IEEE Access, vol. 10, pp. 3284-3293, 2021.
[CrossRef] [Google Scholar] [Publisher Link]
[5] Neha N. Ganvir, and D.M. Yadav, “Filtering Method for Pre-Processing Mammogram Images for Breast Cancer Detection,” International Journal of Engineering and Advanced Technology, vol. 9, no. 1, pp. 4222-4229, 2019.
[CrossRef] [Google Scholar] [Publisher Link]
[6] R. Ramani et al., “The Pre-Processing Techniques for Breast Cancer Detection in Mammography Images,” International Journal of Image, Graphics and Signal Processing, vol. 5, no. 5, pp. 47-54, 2013.
[CrossRef] [Google Scholar] [Publisher Link]
[7] Nijad Al-Najdawi, Mariam Biltawi, and Sara Tedmori, “Mammogram Image Visual Enhancement, Mass Segmentation and Classification,” Applied Soft Computing, vol. 35, pp. 175-185, 2015.
[CrossRef] [Google Scholar] [Publisher Link]
[8] Milos Radovic et al., “Parameter Optimization of a Computer-Aided Diagnosis System for Detection of Masses on Digitized Mammograms,” Technology and Health Care, vol. 23, no. 6, pp. 757-774, 2015.
[CrossRef] [Google Scholar] [Publisher Link]
[9] Hanife Avcı, and Jale Karakaya, “A Novel Medical Image Enhancement Algorithm for Breast Cancer Detection on Mammography Images Using Machine Learning,” Diagnostics, vol. 13, no. 3, pp. 1-14, 2023.
[CrossRef] [Google Scholar] [Publisher Link]
[10] P. Esther Jebarani et al., “A Novel Hybrid K-Means and GMM Machine Learning Model for Breast Cancer Detection,” IEEE Access, vol. 9, pp. 146153-146162, 2021.
[CrossRef] [Google Scholar] [Publisher Link]
[11] Rajeshwari S. Patil, and Nagashettappa Biradar, “Improved Region Growing Segmentation for Breast Cancer Detection: Progression of Optimized Fuzzy Classifier,” International Journal of Intelligent Computing and Cybernetics, vol. 13, no. 2, pp. 181-205, 2020.
[CrossRef] [Google Scholar] [Publisher Link]
[12] Naglaa F. Soliman et al., “An Efficient Breast Cancer Detection Framework for Medical Diagnosis Applications,” Computers, Materials & Continua, vol. 70, no. 1, pp. 1-20, 2022.
[CrossRef] [Google Scholar] [Publisher Link]
[13] Milosevic, Marina et al., “Early Diagnosis and Detection of Breast Cancer,” Technology and Health Care, vol. 26, no. 4, pp. 729-759, 2018.
[CrossRef] [Google Scholar] [Publisher Link]
[14] Selina Sharmin et al., “A Hybrid Dependable Deep Feature Extraction and Ensemble-Based Machine Learning Approach for Breast Cancer Detection,” IEEE Access, vol. 11, pp. 87694-87708, 2023.
[CrossRef] [Google Scholar] [Publisher Link]
[15] Amita Das et al., “Deep Learning Based Liver Cancer Detection Using Watershed Transform and Gaussian Mixture Model Techniques,” Cognitive Systems Research, vol. 54, pp. 165-175, 2019.
[CrossRef] [Google Scholar] [Publisher Link]
[16] Kumari Nibha Priyadarshani, and Sangeeta Singh, “Ultra-Sensitive Breast Cancer Cell Lines Detection Using Dual Nanocavities Engraved Junctionless FET,” IEEE Transactions on NanoBioscience, vol. 22, no. 4, pp. 889-896, 2023.
[CrossRef] [Google Scholar] [Publisher Link]
[17] Irum Hirra et al., “Breast Cancer Classification from Histopathological Images Using Patch-Based Deep Learning Modeling,” IEEE Access, vol. 9, pp. 24273-24287, 2021.
[CrossRef] [Google Scholar] [Publisher Link]
[18] Jiande Wu, and Chindo Hicks, “Breast Cancer Type Classification Using Machine Learning,” Journal of Personalized Medicine, vol. 11, no. 2, pp. 1-12, 2021.
[CrossRef] [Google Scholar] [Publisher Link]
[19] Kapil Juneja, and Chhavi Rana, “An Improved Weighted Decision Tree Approach For Breast Cancer Prediction,” International Journal of Information Technology, vol. 12, no. 3, pp.797-804, 2020.
[CrossRef] [Google Scholar] [Publisher Link]
[20] Muhammad Danish Ali et al., “Breast Cancer Classification through Meta-Learning Ensemble Technique Using Convolution Neural Networks,” Diagnostics, vol. 13, no. 13, pp. 1-19, 2023.
[CrossRef] [Google Scholar] [Publisher Link]
[21] Mona Alfifi et al., “Enhanced Artificial Intelligence System for Diagnosing and Predicting Breast Cancer Using Deep Learning,” International Journal of Advanced Computer Science and Applications, vol. 11, no. 7, pp. 1-16, 2020.
[CrossRef] [Google Scholar] [Publisher Link]
[22] Mehedi Masud, Amr E. Eldin Rashed, and M. Shamim Hossain, “Convolutional Neural Network-Based Models for Diagnosis Of Breast Cancer,” Neural Computing and Applications, vol. 34, no. 14, pp. 11383-11394, 2022.
[CrossRef] [Google Scholar] [Publisher Link]