A Comprehensive Survey of Machine Learning in Healthcare: Predicting Heart and Liver Disease, Tuberculosis Detection in Chest X-Ray Images
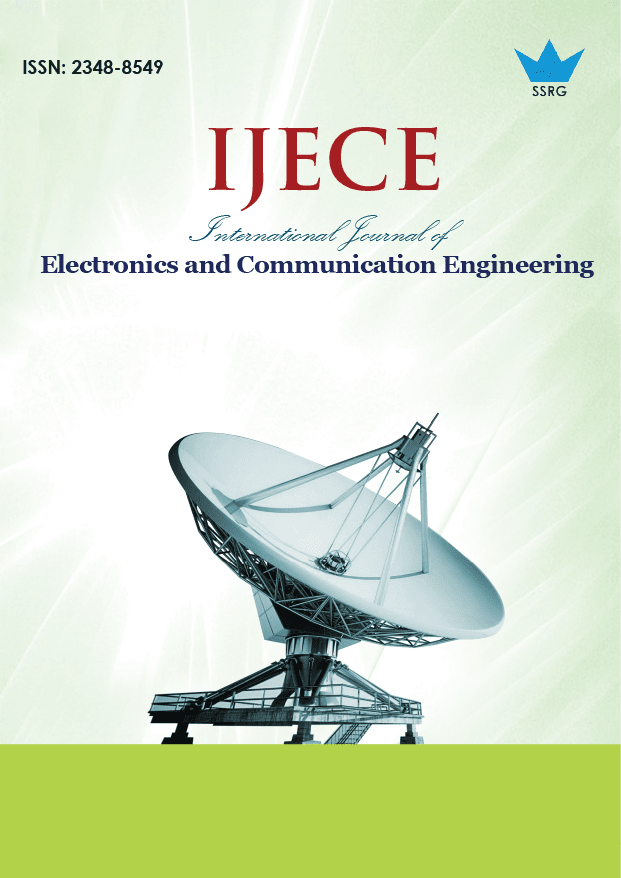
International Journal of Electronics and Communication Engineering |
© 2024 by SSRG - IJECE Journal |
Volume 11 Issue 5 |
Year of Publication : 2024 |
Authors : Chandini Banapuram, Azmera Chandu Naik, Madhu Kumar Vanteru, V Sravan Kumar, Karthik Kumar Vaigandla |
How to Cite?
Chandini Banapuram, Azmera Chandu Naik, Madhu Kumar Vanteru, V Sravan Kumar, Karthik Kumar Vaigandla, "A Comprehensive Survey of Machine Learning in Healthcare: Predicting Heart and Liver Disease, Tuberculosis Detection in Chest X-Ray Images," SSRG International Journal of Electronics and Communication Engineering, vol. 11, no. 5, pp. 155-169, 2024. Crossref, https://doi.org/10.14445/23488549/IJECE-V11I5P116
Abstract:
The utilization of Machine Learning (ML) has become widespread across several disciplines. ML is utilized as an effective support mechanism in clinical diagnostics due to the abundance of publicly accessible data. The prevalence of heart and liver disease has been seen to increase dramatically due to factors such as excessive alcohol intake, inhalation of toxic gases, narcotics usage, food contamination, and bad lifestyle choices among individuals. Both cardiovascular disease and liver disease are significant contributors to global death rates. The early detection of these disorders is of utmost importance in order to preserve individuals' lives. The integration of ML classification algorithms into healthcare institutions has demonstrated notable results, enabling medical professionals to expedite and enhance illness diagnosis with heightened precision. The use of healthcare data may be effectively employed to select the most suitable trial sample, gather more data points, evaluate continuous data from trial participants, and mitigate mistakes arising from data analysis. ML techniques play a crucial role in identifying initial signs of an epidemic or pandemic. The system analyses satellite data, news and social media reports, as well as video sources to ascertain the potential escalation of the illness. The use of ML in the healthcare sector has the potential to introduce a multitude of opportunities and advancements in this domain. The automation of information retrieval and data entry tasks allows healthcare personnel to allocate their time more effectively towards patient care rather than being occupied with such administrative duties. This paper explores the many uses of ML in the field of healthcare industry, highlighting its significance. It subsequently explores the relevant characteristics and essential components of ML that are suitable for the healthcare framework. Ultimately, the study successfully discovered and thoroughly examined the notable uses of ML in the field of healthcare. The utilization of this technology in healthcare operations can yield significant benefits for society.
Keywords:
Artificial Intelligence (AI), Chest X-ray, Deep Learning(DL), Healthcare, Machine Learning(ML), Heart disease, Liver disease, Particle Swarm Optimization(PSO), Tuberculosis, Support Vector Machine(SVM).
References:
[1] Pokkuluri Kiran Sree et al., “A Comprehensive Analysis on Risk Prediction of Heart Disease Using Machine Learning Models,” International Journal on Recent and Innovation Trends in Computing and Communication, vol. 11, no. 11S, pp. 605-610, 2023.
[CrossRef] [Google Scholar] [Publisher Link]
[2] Mandakini Priyadarshani Behera et al., “A Hybrid Machine Learning Algorithm for Heart and Liver Disease Prediction Using Modified Particle Swarm Optimization with Support Vector Machine,” Procedia Computer Science, vol. 218, pp. 818-827, 2023.
[CrossRef] [Google Scholar] [Publisher Link]
[3] J. Vijayashree, and H. Parveen Sultana, “A Machine Learning Framework for Feature Selection in Heart Disease Classification Using Improved Particle Swarm Optimization with Support Vector Machine Classifier,” Programming and Computer Software, vol. 44, pp. 388-397, 2018.
[CrossRef] [Google Scholar] [Publisher Link]
[4] Mohd Javaid et al., “Significance of Machine Learning in Healthcare: Features, Pillars and Applications,” International Journal of Intelligent Networks, vol. 3, pp. 58-73, 2022.
[CrossRef] [Google Scholar] [Publisher Link]
[5] Mark P. Sendak et al., “A Path for Translation of Machine Learning Products into Healthcare Delivery,” European Medical Journal Innovations, vol. 10, pp. 1-14, 2020.
[CrossRef] [Google Scholar] [Publisher Link]
[6] Aakansha Gupta, and Rahul Katarya, “Social Media Based Surveillance Systems for Healthcare Using Machine Learning: A Systematic Review,” Journal of Biomedical Informatics, vol. 108, pp. 1-13, 2020.
[CrossRef] [Google Scholar] [Publisher Link]
[7] Allan Tucker et al., “Generating High-Fidelity Synthetic Patient Data for Assessing Machine Learning Healthcare Software,” NPJ Digital Medicine, vol. 3, no. 1, pp. 1-13, 2020.
[CrossRef] [Google Scholar] [Publisher Link]
[8] Irene Y. Chen et al., “Probabilistic Machine Learning for Healthcare,” Annual Review of Biomedical Data Science, vol. 4, pp. 393-415, 2020.
[CrossRef] [Google Scholar] [Publisher Link]
[9] Sarkar Siddique, and James C.L. Chow, “Machine Learning in Healthcare Communication,” Encyclopedia, vol. 1, no. 1, pp. 220-239, 2021.
[CrossRef] [Google Scholar] [Publisher Link]
[10] Jonathan Waring, Charlotta Lindvall, and Renato Umeton, “Automated Machine Learning: Review of the State-of-the-Art and Opportunities for Healthcare,” Artificial Intelligence in Medicine, vol. 104, pp. 1-12, 2020.
[CrossRef] [Google Scholar] [Publisher Link]
[11] Muhammad Aurangzeb Ahmad et al., “Fairness in Machine Learning for Healthcare,” Proceedings of the 26th ACM SIGKDD International Conference on Knowledge Discovery & Data Mining, CA, USA, pp. 3529-3530, 2020.
[CrossRef] [Google Scholar] [Publisher Link]
[12] Gunasekaran Manogaran, and Daphne Lopez, “A Survey of Big Data Architectures and Machine Learning Algorithms in Healthcare,” International Journal of Biomedical Engineering and Technology, vol. 25, no. 2-4, pp. 182-211, 2017.
[CrossRef] [Google Scholar] [Publisher Link]
[13] Jan A. Roth et al., “Introduction to Machine Learning in Digital Healthcare Epidemiology,” Infection Control & Hospital Epidemiology, vol. 39, no. 12, pp. 1457-1462, 2018.
[CrossRef] [Google Scholar] [Publisher Link]
[14] Rohan Bhardwaj, Ankita R. Nambiar, and Debojyoti Dutta, “A Study of Machine Learning in Healthcare,” 2017 IEEE 41st Annual Computer Software and Applications Conference, Turin, Italy, pp. 236-241, 2017.
[CrossRef] [Google Scholar] [Publisher Link]
[15] Amin Ul Haq et al., “A Hybrid Intelligent System Framework for the Prediction of Heart Disease Using Machine Learning Algorithms,” Mobile Information Systems, vol. 2018, pp. 1-22, 2018.
[CrossRef] [Google Scholar] [Publisher Link]
[16] Dong Zhao et al., “Epidemiology of Cardiovascular Disease in China: Current Features and Implications,” Nature Reviews Cardiology, vol. 16, no. 4, pp. 203-212, 2019.
[CrossRef] [Google Scholar] [Publisher Link]
[17] Muriel Levy et al., “Socioeconomic Differences in Health-Care Use and Outcomes for Stroke and Ischaemic Heart Disease in China During 2009–16: A Prospective Cohort Study of 0·5 Million Adults,” Lancet Global Health, vol. 8, no. 4, pp. e591-e602, 2020.
[CrossRef] [Google Scholar] [Publisher Link]
[18] Heng Zhou et al., “Activating Transcription Factor 3 in Cardiovascular Diseases: A Potential Therapeutic Target,” Basic Research in Cardiology, vol. 113, pp. 1-13, 2018.
[CrossRef] [Google Scholar] [Publisher Link]
[19] Giovanni Paragliola, and Antonio Coronato, “An Hybrid ECG-Based Deep Network for the Early Identification of High-Risk to Major Cardiovascular Events for Hypertension Patients,” Journal of Biomedical Informatics, vol. 113, pp. 1-10, 2021.
[CrossRef] [Google Scholar] [Publisher Link]
[20] Ahmed Al Ahdal et al., “Monitoring Cardiovascular Problems in Heart Patients Using Machine Learning,” Journal of Healthcare Engineering, vol. 2023, pp. 1-16, 2023.
[CrossRef] [Google Scholar] [Publisher Link]
[21] Archana Karne et al., “Convolutional Neural Networks for Object Detection and Recognition,” Journal of Artificial Intelligence, Machine Learning and Neural Network, vol. 3, no. 2, pp. 1-13, 2023.
[CrossRef] [Google Scholar] [Publisher Link]
[22] Youngga Rega Nugraha, Aji Prasetya Wibawa, and Ilham Ari Elbaith Zaeni, “Particle Swarm Optimization – Support Vector Machine (PSO-SVM) Algorithm for Journal Rank Classification,” 2019 2nd International Conference of Computer and Informatics Engineering, Banyuwangi, Indonesia, pp. 69-73, 2019.
[CrossRef] [Google Scholar] [Publisher Link]
[23] Xiaofeng Li et al., “Particle Swarm Optimization-Support Vector Machine Model for Machinery Fault Diagnoses in High-Voltage Circuit Breakers,” Chinese Journal of Mechanical Engineering, vol. 33, pp. 1-10, 2020.
[CrossRef] [Google Scholar] [Publisher Link]
[24] S. Vijayarani, and S. Dhayanand, “Liver Disease Prediction Using SVM and Naïve Bayes Algorithms,” International Journal of Science, Engineering and Technology Research, vol. 4, no. 4, pp. 816-820, 2015.
[Google Scholar]
[25] K. Thirunavukkarasu et al., “Prediction of Liver Disease using Classification Algorithms,” 2018 4th International Conference on Computing Communication and Automation, Greater Noida, India, pp. 1-3, 2018.
[CrossRef] [Google Scholar] [Publisher Link]
[26] Archana Singh, and Rakesh Kumar, “Heart Disease Prediction Using Machine Learning Algorithms,” 2020 International Conference on Electrical and Electronics Engineering, Gorakhpur, India, pp. 452-457, 2020.
[CrossRef] [Google Scholar] [Publisher Link]
[27] M. Senbagavalli, and Arasu G. Tholkappia, “Opinion Mining for Cardiovascular Disease Using Decision Tree Based Feature Selection,” Asian Journal of Research in Social Sciences and Humanities, vol. 6, no. 8, pp. 891-897, 2016.
[CrossRef] [Google Scholar] [Publisher Link]
[28] M.S. Valli, and G.T. Arasu, “An Efficient Feature Selection Technique of Unsupervised Learning Approach for Analyzing Web Opinions,” Journal of Scientific & Industrial Research, vol. 75, pp. 221-224, 2016.
[Google Scholar] [Publisher Link]
[29] Mien Van, Duy Tang Hoang, and Hee Jun Kang, “Bearing Fault Diagnosis Using a Particle Swarm Optimization-Least Squares Wavelet Support Vector Machine Classifier,” Sensors, vol. 20, no. 12, pp. 1-19, 2020.
[CrossRef] [Google Scholar] [Publisher Link]
[30] Qingyun Yu et al., “PSO-SVM Based Performance-Driving Scheduling Method for Semiconductor Manufacturing Systems,” Applied Sciences, vol. 13, no. 20, 2023.
[CrossRef] [Google Scholar] [Publisher Link]
[31] Emrana Kabir Hashi, and Md. Shahid Uz Zaman, “Developing a Hyperparameter Tuning Based Machine Learning Approach of Heart Disease Prediction,” Journal of Applied Science & Process Engineering, vol. 7, no. 2, pp. 631-647, 2020.
[CrossRef] [Google Scholar] [Publisher Link]
[32] Ashir Javeed et al., “An Intelligent Learning System Based on Random Search Algorithm and Optimized Random Forest Model for Improved Heart Disease Detection,” IEEE Access, vol. 7, pp. 180235-180243, 2019.
[CrossRef] [Google Scholar] [Publisher Link]
[33] Senthilkumar Mohan, Chandrasegar Thirumalai, and Gautam Srivastava, “Effective Heart Disease Prediction Using Hybrid Machine Learning Techniques,” IEEE Access, vol. 7, pp. 81542-81554, 2019.
[CrossRef] [Google Scholar] [Publisher Link]
[34] Liaqat Ali et al., “An Automated Diagnostic System for Heart Disease Prediction Based on χ2 Statistical Model and Optimally Configured Deep Neural Network,” IEEE Access, vol. 7, pp. 34938-34945, 2019.
[CrossRef] [Google Scholar] [Publisher Link]
[35] David Selasi Koblah et al., “A Survey and Perspective on Artificial Intelligence for Security- Aware Electronic Design Automation,” arXiv, pp. 1-55, 2022.
[CrossRef] [Google Scholar] [Publisher Link]
[36] Ahmad Khajenezhad, Mohammad Ali Bashiri, and Hamid Beigy, “A Distributed Density Estimation Algorithm and its Application to Naive Bayes Classification,” Applied Soft Computing, vol. 98, 2021.
[CrossRef] [Google Scholar] [Publisher Link]
[37] K. Shankar et al., “Retracted Article: Optimal Feature-Based Multi-Kernel SVM Approach for Thyroid Disease Classification,” The Journal of Supercomputing, vol. 76, pp. 1128-1143, 2020.
[CrossRef] [Google Scholar] [Publisher Link]
[38] Harikumar Pallathadka et al., “Impact of Machine Learning on Management, Healthcare and Agriculture,” Materials Today: Proceedings, vol. 80, pp. 2803-2806, 2023.
[CrossRef] [Google Scholar] [Publisher Link]
[39] B. Kalaiselvi, and M. Thangamani, “An Efficient Pearson Correlation Based Improved Random Forest Classification for Protein Structure Prediction Techniques,” Measurement, vol. 162, 2020.
[CrossRef] [Google Scholar] [Publisher Link]
[40] J. Saleem Tausifa, and Chishti Mohammad Ahsan, “Exploring the Applications of Machine Learning in Healthcare,” International Journal of Sensors Wireless Communications and Control, vol. 10, no. 4, pp. 458-472, 2020.
[CrossRef] [Google Scholar] [Publisher Link]
[41] Flávio H.D. Araújo, André M. Santana, and Pedro de A. Santos Neto, “Using Machine Learning to Support Healthcare Professionals in Making Preauthorisation Decisions,” International Journal of Medical Informatics, vol. 94, pp. 1-7, 2016.
[CrossRef] [Google Scholar] [Publisher Link]
[42] Sonali Vyas, Mahima Gupta, and Rakesh Yadav, “Converging Blockchain and Machine Learning for Healthcare,” 2019 Amity International Conference on Artificial Intelligence, Dubai, United Arab Emirates, pp. 709-711, 2019.
[CrossRef] [Google Scholar] [Publisher Link]
[43] Jian Ping Li et al., “Heart Disease Identification Method Using Machine Learning Classification in E-Healthcare,” IEEE Access, vol. 8, pp. 107562-107582, 2020.
[CrossRef] [Google Scholar] [Publisher Link]
[44] Wasudeo Rahane et al., “Lung Cancer Detection Using Image Processing and Machine Learning HealthCare,” 2018 International Conference on Current Trends towards Converging Technologies, Coimbatore, India, pp. 1-5, 2018.
[CrossRef] [Google Scholar] [Publisher Link]
[45] Zeeshan Ahmed et al., “Artificial Intelligence with Multi-Functional Machine Learning Platform Development for Better Healthcare and Precision Medicine,” Database, vol. 2020, pp. 1-35, 2020.
[CrossRef] [Google Scholar] [Publisher Link]
[46] J. Kennedy, and R. Eberhart, “Particle Swarm Optimization,” Proceedings of ICNN'95 - International Conference on Neural Networks, Perth, WA, Australia, vol. 4, pp. 1942-1948, 1995.
[CrossRef] [Google Scholar] [Publisher Link]
[47] Arun Kumar, Alaknanda Ashok, and M.A. Ansari, “Brain Tumor Classification Using Hybrid Model of PSO And SVM Classifier,” 2018 International Conference on Advances in Computing, Communication Control and Networking, Greater Noida, India, pp. 1022-1026, 2018.
[CrossRef] [Google Scholar] [Publisher Link]
[48] Hui Wang et al., “A Hybrid Particle Swarm Algorithm with Cauchy Mutation,” 2007 IEEE Swarm Intelligence Symposium, Honolulu, HI, USA, pp. 356-360, 2007.
[CrossRef] [Google Scholar] [Publisher Link]
[49] G.S. Monisha et al., “A Enhanced Approach for Identification of Tuberculosis for Chest X-Ray Image Using Machine Learning,” International Journal on Recent and Innovation Trends in Computing and Communication, vol. 11, no. 11S, pp. 443-453, 2023.
[CrossRef] [Publisher Link]