Innovative IoT-Enabled Food Nutrient Profiling with Deep Learning Techniques
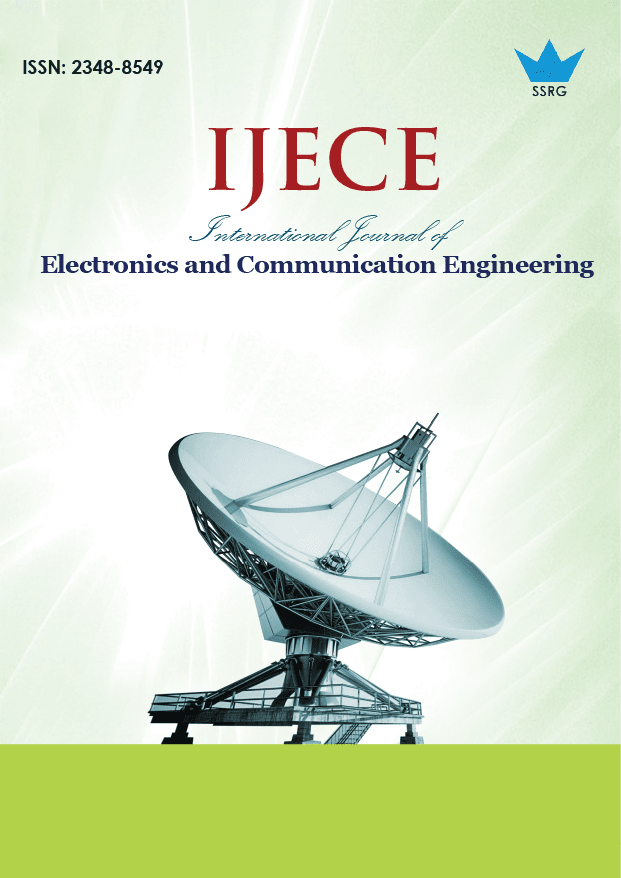
International Journal of Electronics and Communication Engineering |
© 2024 by SSRG - IJECE Journal |
Volume 11 Issue 5 |
Year of Publication : 2024 |
Authors : Sharanagouda N Patil, Ramesh M. Kagalkar |
How to Cite?
Sharanagouda N Patil, Ramesh M. Kagalkar, "Innovative IoT-Enabled Food Nutrient Profiling with Deep Learning Techniques," SSRG International Journal of Electronics and Communication Engineering, vol. 11, no. 5, pp. 179-194, 2024. Crossref, https://doi.org/10.14445/23488549/IJECE-V11I5P118
Abstract:
As living costs in large cities continue to rise, and the health and safety concerns associated with eating fast food become more apparent, an increasing number of office workers are opting to bring their lunches from home. Traditionally, these lunches are reheated using microwaves, thermal lunch boxes, or electric heating containers. This device introduces a novel solution by enabling users to control the temperature of their lunchbox remotely via a smartphone application. This innovative device automates several conventional functions, such as setting timers for food heating, thus providing a more convenient alternative to traditional microwaves. Not only does it eliminate the need for an external power source during heating, but it also promotes healthier eating habits by tracking the nutritional content of the meals. The device is ideal for users seeking the convenience of enjoying hot meals on the go, making it a significant advancement in personal meal management.
Keywords:
Dietary management technology, Food detection, Machine learning in food analysis, Nutritional analysis, Remote temperature control, Smart tiffin box.
References:
[1] Chairote Yaiprasert, and Achmad Nizar Hidayanto, “AI-Powered in the Digital Age: Ensemble Innovation Personalizes the Food Recommendations,” Journal of Open Innovation: Technology, Market, and Complexity, vol. 10, no. 2, pp. 1-14, 2024.
[CrossRef] [Google Scholar] [Publisher Link]
[2] Saloni Joshi et al., “Artificial Intelligence Assisted Food Science and Nutrition Perspective for Smart Nutrition Research and Healthcare,” Systems Microbiology and Biomanufacturing, vol. 4, pp. 86-101, 2024.
[CrossRef] [Google Scholar] [Publisher Link]
[3] Ahmad Qarajeh et al., “AI-Powered Renal Diet Support: Performance of ChatGPT, Bard AI, and Bing Chat,” Clinics and Practice, vol. 13, no. 5, pp. 1160-1172, 2023.
[CrossRef] [Google Scholar] [Publisher Link]
[4] Zahra Namkhah et al., “Advancing Sustainability in the Food and Nutrition System: A Review of Artificial Intelligence Applications,” Frontiers in Nutrition, vol. 10, pp. 1-8, 2023.
[CrossRef] [Google Scholar] [Publisher Link]
[5] R. Dinesh Kumar et al., “Recognition of Food Type and Calorie Estimation Using Neural Network,” The Journal of Supercomputing, vol. 77, pp. 8172-8193, 2021.
[CrossRef] [Google Scholar] [Publisher Link]
[6] Landu Jiang et al., “DeepFood: Food Image Analysis and Dietary Assessment via Deep Model,” IEEE Access, vol. 8, pp. 47477-47489, 2020.
[CrossRef] [Google Scholar] [Publisher Link]
[7] Shuqiang Jiangn et al., “Multi-Scale Multi-View Deep Feature Aggregation for Food Recognition,” IEEE Transactions on Image Processing, vol. 29, pp. 265-276, 2020.
[CrossRef] [Google Scholar] [Publisher Link]
[8] Erdal Tasci, “Voting Combinations-Based Ensemble of Fine-Tuned Convolutional Neural Networks for Food Image Recognition,” Multimedia Tools and Applications, vol. 79, pp. 30397-30418, 2020.
[CrossRef] [Google Scholar] [Publisher Link]
[9] Dang Thi Phuong Chung, and Dinh Van Tai, “A Fruits Recognition System Based on a Modern Deep Learning Technique,” Journal of Physics: Conference Series, International Conference on Innovations in Non-Destructive Testing SibTest, Yekaterinburg, Russia, vol. 1327, pp. 1-5, 2019.
[CrossRef] [Google Scholar] [Publisher Link]
[10] Mohammed Ahmed Subhi, Sawal Hamid Ali, and Mohammed Abulameer Mohammed, “Vision-Based Approaches for Automatic Food Recognition and Dietary Assessment: A Survey,” IEEE Access, vol. 7, pp. 35370-35381, 2019.
[CrossRef] [Google Scholar] [Publisher Link]
[11] Chang Liu et al., “A New Deep Learning-Based Food Recognition System for Dietary Assessment on an Edge Computing Service Infrastructure,” IEEE Transactions on Services Computing, vol. 11, no. 2, pp. 249-261, 2018.
[CrossRef] [Google Scholar] [Publisher Link]
[12] Sirichai Turmchokkasam, and Kosin Chamnongthai, “The Design and Implementation of an Ingredient-Based Food Calorie Estimation System Using Nutrition Knowledge and Fusion of Brightness and Heat Information,” IEEE Access, vol. 6, pp. 46863-46876, 2018.
[CrossRef] [Google Scholar] [Publisher Link]
[13] Gianluigi Ciocca, Paolo Napoletano, and Raimondo Schettini, “Food Recognition: A New Dataset, Experiments, and Results,” IEEE Journal of Biomedical and Health Informatics, vol. 21, no. 3, pp. 588-598, 2017.
[CrossRef] [Google Scholar] [Publisher Link]
[14] Eduardo Aguilar, Marc Bolaños, and Petia Radeva, “Food Recognition Using Fusion of Classifiers Based on CNNs,” Image Analysis and Processing: 19th International Conference, Catania, Italy, pp. 213-224, 2017.
[CrossRef] [Google Scholar] [Publisher Link]
[15] Hongsheng He, Fanyu Kong, and Jindong Tan, “DietCam: Multiview Food Recognition Using a Multikernel SVM,” IEEE Journal of Biomedical and Health Informatics, vol. 20, no. 3, pp. 848-855, 2016.
[CrossRef] [Google Scholar] [Publisher Link]
[16] Shuang Ao, and Charles X. Ling, “Adapting New Categories for Food Recognition with Deep Representation,” 2015 IEEE International Conference on Data Mining Workshop, Atlantic City, NJ, USA, pp. 1196-1203, 2015.
[CrossRef] [Google Scholar] [Publisher Link]
[17] Ping Kuang, Wei-Na Cao, and Qiao Wu, “Preview on Structures and Algorithms of Deep Learning,” 2014 11th International Computer Conference on Wavelet Actiev Media Technology and Information Processing, Chengdu, China, pp. 176-179, 2014.
[CrossRef] [Google Scholar] [Publisher Link]
[18] Parisa Pouladzadeh, Shervin Shirmohammadi, and Rana Al-Maghrabi, “Measuring Calorie and Nutrition From Food Image,” IEEE Transactions on Instrumentation and Measurement, vol. 63, no. 8, pp. 1947-1956, 2014.
[CrossRef] [Google Scholar] [Publisher Link]
[19] Mohd Amran Mohd Daril et al., “Unveiling the Landscape of Big Data Analytics in Healthcare: A Comprehensive Bibliometric Analysis,” International Journal of Online and Biomedical Engineering, vol. 20, no. 6, pp. 4-24, 2024.
[CrossRef] [Google Scholar] [Publisher Link]