Enhancing Lossless Image Compression through Smart Partitioning, Selective Encoding, and Wavelet Analysis
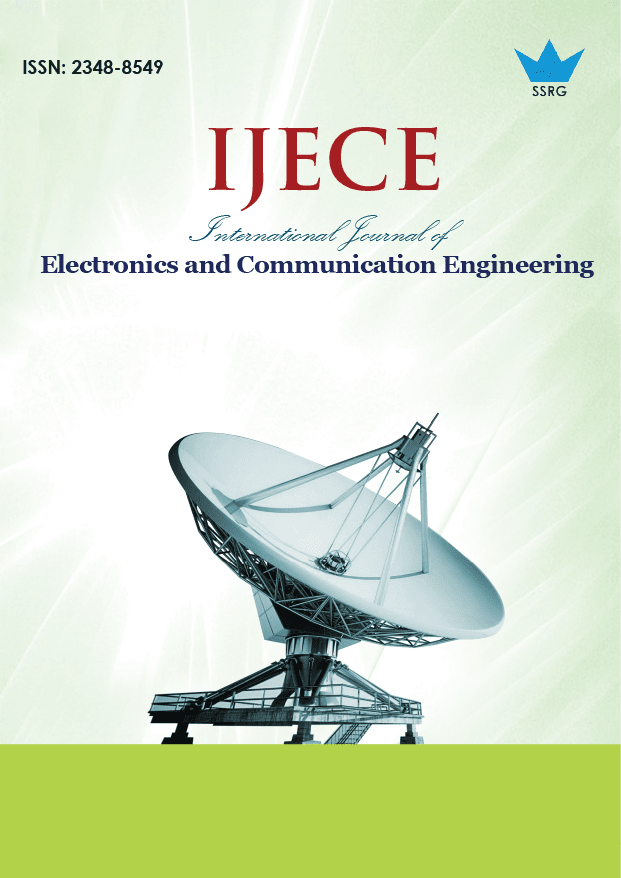
International Journal of Electronics and Communication Engineering |
© 2024 by SSRG - IJECE Journal |
Volume 11 Issue 5 |
Year of Publication : 2024 |
Authors : M. Sri Raghavendra, Pasuluri Bindu Swetha, K. Sreenivasulu, B. V. Srinivasulu, V. Jyothsna |
How to Cite?
M. Sri Raghavendra, Pasuluri Bindu Swetha, K. Sreenivasulu, B. V. Srinivasulu, V. Jyothsna, "Enhancing Lossless Image Compression through Smart Partitioning, Selective Encoding, and Wavelet Analysis," SSRG International Journal of Electronics and Communication Engineering, vol. 11, no. 5, pp. 207-219, 2024. Crossref, https://doi.org/10.14445/23488549/IJECE-V11I5P120
Abstract:
This paper presents a cutting-edge algorithmic framework for lossless image compression, directly addressing the limitations and quality compromises inherent in existing compression models. Traditional approaches often fail to effectively balance efficiency with quality retention across various image complexities, leading to degraded image fidelity. Our proposed framework distinguishes itself by adeptly integrating smart partitioning, selective encoding, and wavelet coefficient analysis, thereby achieving marked improvements in compression efficiency without sacrificing image quality. Essential to the framework's efficacy is a methodical approach to image preprocessing, which ensures images are in an optimal state for processing. Through rigorous images and evaluation against industry standards such as JPEG2000 and PNG, the proposed model demonstrated exceptional performance enhancements: achieving compression ratios up to 4.2:1, enhancing Peak Signalto-Noise Ratios (PSNR) to 49 dB for low complexity images, and maintaining Structural Similarity Index (SSIM) values as high as 0.99. These quantitative outcomes not only underline the model's superior compression capability but also its robustness in preserving the structural and perceptual quality of images across varying complexities. The significance of this research lies in its potential to redefine benchmarks within the lossless image compression domain, as evidenced by its superior performance metrics. Further exploration into machine learning for partitioning automation, real-time adaptive encoding mechanisms, and expanded framework applicability promises to optimize compression efficiency further. Ultimately, this study lays a foundational stone for future advancements in digital image management, addressing the critical need for high-efficiency, quality-conserving image compression solutions.
Keywords:
Lossless image compression, Smart partitioning, Selective encoding, Wavelet coefficient analysis, Image quality preservation, Real-time processing.
References:
[1] Sid Ahmed Elhannachi, Nacéra Benamrane, and Taleb-Ahmed Abdelmalik, “Adaptive Medical Image Compression Based on Lossy and Lossless Embedded Zerotree Methods,” Journal of Information Processing Systems, vol. 13, no. 1, pp. 40-56, 2017.
[CrossRef] [Google Scholar] [Publisher Link]
[2] Jacob Ström, and Pamela C. Cosman, “Medical Image Compression with Lossless Regions of Interest,” Signal Processing, vol. 59, no. 2, pp. 155-171, 1997.
[CrossRef] [Google Scholar] [Publisher Link]
[3] S. Zhang, F. Fdez-Riverola, and S. Kiran, “Granular Partitioning and Adaptive Encoding: A Synergistic Approach to Lossless Image Compression,” International Journal of Computer Engineering in Research Trends, vol. 11, no. 3, pp. 54-63, 2024.
[Publisher Link]
[4] M.A. Rahman et al., “Efficient Colour Image Compression Using Fusion Approach,” The Imaging Science Journal, vol. 64, no. 3, pp. 166-177, 2016.
[CrossRef] [Google Scholar] [Publisher Link]
[5] K. Jeyakumar, “Image Compression and Fusion Based Technology Using Wavelet Transform,” Indian Journal of Science and Technology, vol. 8, no. 32, pp. 1-8, 2015.
[CrossRef] [Google Scholar] [Publisher Link]
[6] Ankita Vaish, and Saumya Patel, “A Sparse Representation-Based Compression of Fused Images Using WDR Coding,” Journal of King Saud University - Computer and Information Sciences, vol. 34, no. 8, pp. 6165-6178, 2022.
[CrossRef] [Google Scholar] [Publisher Link]
[7] Fulong Yang et al., “Double JPEG Compression Detection Based On Fusion Features,” Machine Learning and Intelligent Communications: Second International Conference, Weihai, China, pp. 158-167, 2018.
[CrossRef] [Google Scholar] [Publisher Link]
[8] K. Venkata Ramana et al., “Secure and Efficient Energy Trading using Homomorphic Encryption on the Green Trade Platform,” International Journal of Intelligent Systems and Applications in Engineering, vol. 12, no. 1s, pp. 345-360, 2024.
[Google Scholar] [Publisher Link]
[9] Ashish Khare, Manish Khare, and Richa Srivastava, “Shearlet Transform Based Technique for Image Fusion Using Median Fusion Rule,” Multimedia Tools and Applications, vol. 80, pp. 11491-11522, 2021.
[CrossRef] [Google Scholar] [Publisher Link]
[10] D.J. Ashpin Pabi, N. Puviarasan, and P. Aruna, “Fast Singular Value Decomposition-Based Image Compression Using Butterfly Particle Swarm Optimization Technique (SVD-BPSO),” International Journal of Computer Engineering in Research Trends, vol. 4, no. 4, pp. 128-135, 2017.
[Google Scholar] [Publisher Link]
[11] Roman Starosolski, “Hybrid Adaptive Lossless Image Compression Based On Discrete Wavelet Transform,” Entropy, vol. 22, no. 7, pp. 1-20, 2020.
[CrossRef] [Google Scholar] [Publisher Link]
[12] Gergely Flamich, Marton Havasi, and José Miguel Hernández-Lobato, “Compressing Images by Encoding Their Latent Representations With Relative Entropy Coding,” arXiv, 2020.
[CrossRef] [Google Scholar] [Publisher Link]
[13] Xiaoxiao Liu et al., “An Improved Lossless Image Compression Algorithm Based On Huffman Coding,” Multimedia Tools and Applications, vol. 81, pp. 4781-4795, 2022.
[CrossRef] [Google Scholar] [Publisher Link]
[14] Rafael Rojas-Hernández et al., “Lossless Medical Image Compression by Using Difference Transform,” Entropy, vol. 24, no. 7, pp. 1-27, 2022.
[CrossRef] [Google Scholar] [Publisher Link]
[15] Mahesh Boddu, and Soumitra Kumar Mandal, “Quantum-Dot Cellular Automata Based Lossless CFA Image Compression Using Improved And Extended Golomb-Rice Entropy Coder,” International Journal of Intelligent Engineering & Systems, vol. 15, no. 2, pp. 12-25, 2022.
[CrossRef] [Google Scholar] [Publisher Link]
[16] Vijaykrishnan Narayanan, and Kevin W. Eliceiri, “Deep Wavelet Packet Decomposition with Adaptive Entropy Modeling for Selective Lossless Image Compression,” Synthesis: A Multidisciplinary Research Journal, vol. 1, no. 1, pp. 1-10, 2023.
[Publisher Link]
[17] Kayithi Varshitha et al., “Optimizing Digital Image Quality Assessment: Format Selection and Methods,” International Journal of Computer Engineering in Research Trends, vol. 10, no. 8, pp. 11-19, 2023.
[CrossRef] [Publisher Link]
[18] Sneha Rahul Mhatre, Arun Kulkarni, and Madhuri Gedam, “Image Compression Using Vector Quantization Based on MSE Approach,” International Journal of Computer Engineering in Research Trends, vol. 3, no. 7, pp. 366-370, 2016.
[Publisher Link]
[19] Mohamed Hamada, and A. Al-Fayadh, “Wavelet-Aided Selective Encoding for Enhanced Lossless Image Compression,” Frontiers in Collaborative Research, vol. 1, no. 2, pp. 1-9, 2023.
[Publisher Link]
[20] Lingineni Pavan Kalyan et al., “Identification of Face Mask Detection Using Convolutional Neural Networks,” International Conference on Artificial Intelligence and Sustainable Engineering, pp. 303-313, 2022.
[CrossRef] [Google Scholar] [Publisher Link]
[21] E.V.N. Jyothi et al., “A Graph Neural Network-based Traffic Flow Prediction System with Enhanced Accuracy and Urban Efficiency,” Journal of Electrical Systems, vol. 19, no. 4, pp. 336-349, 2023.
[CrossRef] [Google Scholar] [Publisher Link]
[22] Bhavsingh Maloth, Ashok Sarabu, and B. Vinod Kumar, “An Adaptive Cross-Layer Mapping Algorithm for Multiview Video Coding over IEEE 802.11 e WLANs,” International Journal of Computer Science Engineering and Technology, vol. 2, no. 2, pp. 858-864, 2012.
[Google Scholar] [Publisher Link]