Advancements in Speech-Based Emotion Recognition and PTSD Detection through Machine and Deep Learning Techniques: A Comprehensive Survey
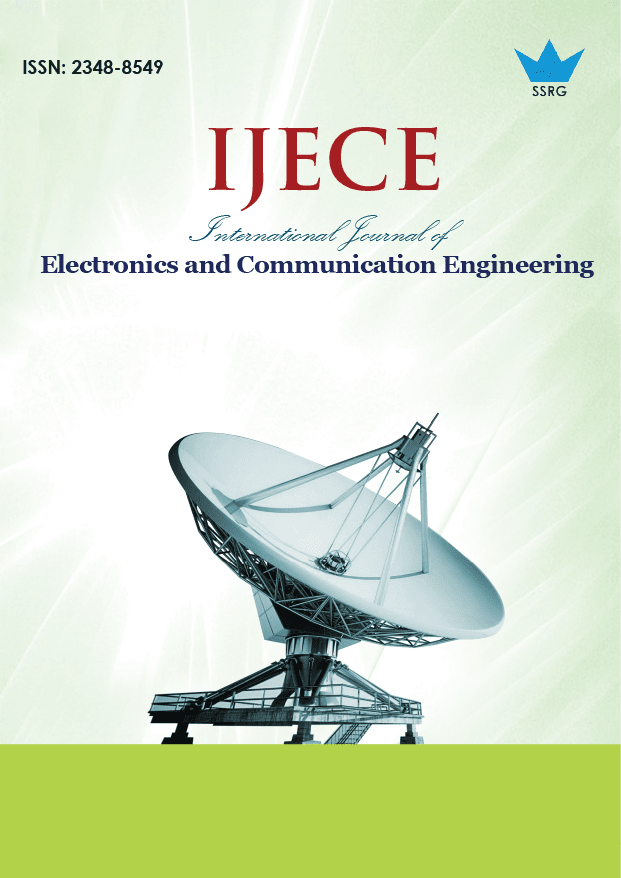
International Journal of Electronics and Communication Engineering |
© 2024 by SSRG - IJECE Journal |
Volume 11 Issue 5 |
Year of Publication : 2024 |
Authors : Chappidi Suneetha, Raju Anitha |
How to Cite?
Chappidi Suneetha, Raju Anitha, "Advancements in Speech-Based Emotion Recognition and PTSD Detection through Machine and Deep Learning Techniques: A Comprehensive Survey," SSRG International Journal of Electronics and Communication Engineering, vol. 11, no. 5, pp. 220-234, 2024. Crossref, https://doi.org/10.14445/23488549/IJECE-V11I5P121
Abstract:
This comprehensive survey delves into the intersection of Machine Learning (ML) and Deep Learning (DL) with speech analysis, showcasing significant strides in detecting and diagnosing Post-Traumatic Stress Disorder (PTSD) through speech-based emotion recognition. By leveraging advanced computational techniques, researchers can identify nuanced speech patterns indicative of PTSD, offering a non-invasive, objective, and scalable diagnostic tool. Despite promising advancements, challenges such as data variability, ethical concerns, and the need for generalizable models persist. The survey highlights the importance of interdisciplinary collaboration, ethical diligence, and the integration of multimodal data to enhance diagnostic accuracy and patient care. Looking forward, it points to a future where speech analysis could revolutionize mental health diagnostics, making it more accessible, personalized, and stigma-free. This work serves as a seminal reference in the field, urging continued innovation and research to fully harness the potential of ML and DL in transforming mental health diagnostics and treatment for PTSD.
Keywords:
Speech analysis, PTSD detection, Machine Learning, Deep Learning, Diagnostic challenges, Interdisciplinary collaboration.
References:
[1] Thole H. Hoppen, Ahlke Kip, and Nexhmedin Morina, “Are Psychological Interventions for Adult PTSD More Efficacious and Acceptable when Treatment is delivered in Higher Frequency? A Meta-Analysis of Randomized Controlled Trials,” Journal of Anxiety Disorders, vol. 95, 2023.
[CrossRef] [Google Scholar] [Publisher Link]
[2] Nicole M. Christ et al., “Using Machine Learning to Predict Sudden Gains in Intensive Treatment for PTSD,” Journal of Anxiety Disorders, vol. 100, pp. 1-10, 2023.
[CrossRef] [Google Scholar] [Publisher Link]
[3] Narayanan Srinivasan, and S.R. Balasundaram, “Minimizing Errors in Air Traffic Speech Using Rule-Based Algorithms,” International Journal of Computer Engineering in Research Trends, vol. 10, no. 12, pp. 42-48, 2023.
[CrossRef] [Publisher Link]
[4] Alice Othmani et al., “Machine-Learning-Based Approaches for Post-Traumatic Stress Disorder Diagnosis Using Video and EEG Sensors: A Review,” IEEE Sensors Journal, vol. 23, no. 20, pp. 24135-24151, 2023.
[CrossRef] [Google Scholar] [Publisher Link]
[5] Mishaim Malik et al., “Automatic Speech Recognition: A Survey,” Multimedia Tools and Applications, vol. 80, no. 6, pp. 9411-9457, 2021.
[CrossRef] [Google Scholar] [Publisher Link]
[6] Shrikanth Narayanan, and Panayiotis G. Georgiou, “Behavioral Signal Processing: Deriving Human Behavioral Informatics from Speech and Language,” Proceedings of the IEEE, vol. 101, no. 5, pp. 1203-1233, 2013.
[CrossRef] [Google Scholar] [Publisher Link]
[7] Julna Nazer, and K. Sajeer, “Automatic Speech Recognition-A Survey,” International Journal of Computer Engineering in Research Trends, vol. 3, no. 4, pp. 190-193, 2016.
[Google Scholar] [Publisher Link]
[8] Maria Labied et al., “An Overview of Automatic Speech Recognition Preprocessing Techniques,” 2022 International Conference on Decision Aid Sciences and Applications, Chiangrai, Thailand, pp. 804-809, 2022.
[CrossRef] [Google Scholar] [Publisher Link]
[9] Uppu Jithendra, Usha Mittal, and Priyanka Chawla, “Audio Detection Using Mel-Frequency Cepstral Coefficients,” 2021 9th International Conference on Reliability, Infocom Technologies and Optimization (Trends and Future Directions), Noida, India, pp. 1-5, 2021.
[CrossRef] [Google Scholar] [Publisher Link]
[10] Yang Liu et al., “LPCSE: Neural Speech Enhancement through Linear Predictive Coding,” GLOBECOM 2022 - 2022 IEEE Global Communications Conference, Rio de Janeiro, Brazil, pp. 5335-5341, 2022.
[CrossRef] [Google Scholar] [Publisher Link]
[11] Sara Sandabad, Achraf Benba, and Hasna Nhaila, “Parkinson’s Syndrome Diagnosis Applying Perceptual Linear Prediction Cepstral Analysis on Several Speech Recordings,” International Journal of Engineering Trends and Technology, vol. 70, no. 9, pp. 214-221, 2022.
[CrossRef] [Publisher Link]
[12] Fanling Huang, and Yangdong Deng, “TCGAN: Convolutional Generative Adversarial Network for Time Series Classification and Clustering,” Neural Networks, vol. 165, pp. 868-883, 2023.
[CrossRef] [Google Scholar] [Publisher Link]
[13] Vishal Passricha, and Rajesh Kumar Aggarwal, “Convolutional Support Vector Machines for Speech Recognition,” International Journal of Speech Technology, vol. 22, no. 3, pp. 601-609, 2019.
[CrossRef] [Google Scholar] [Publisher Link]
[14] K.A. Senthil Devi, and B. Srinivasan, “Spoken Keyword Spotting System Design Using Various Wavelet Transformation Techniques with BPNN Classifier,” International Journal of Computer Engineering in Research Trends, vol. 4, no. 3, pp. 111-118, 2017.
[Publisher Link]
[15] Kai Yu et al., “Context Adaptive Training with Factorized Decision Trees for HMM-Based Statistical Parametric Speech Synthesis,” Speech Communication, vol. 53, no. 6, pp. 914-923, 2011.
[CrossRef] [Google Scholar] [Publisher Link]
[16] Fatemeh Noroozi et al., “Vocal-Based Emotion Recognition Using Random Forests and Decision Tree,” International Journal of Speech Technology, vol. 20, no. 2, pp. 239-246, 2017.
[CrossRef] [Google Scholar] [Publisher Link]
[17] Miao Liu et al., “Non-Intrusive Speech Quality Assessment Based on Deep Neural Networks for Speech Communication,” IEEE Transactions on Neural Networks and Learning Systems, pp. 1-14, 2023.
[CrossRef] [Google Scholar] [Publisher Link]
[18] Hyeong II Koh, Sungdae Na, and Myoung Nam Kim, “Speech Perception Improvement Algorithm Based on a Dual-Path Long ShortTerm Memory Network,” Bioengineering, vol. 10, no. 11, pp. 1-12, 2023.
[CrossRef] [Google Scholar] [Publisher Link]
[19] K. Islam, and Z. ElSayed, “Speech-Based Emotion Recognition and PTSD Detection through Machine and Deep Learning,” International Journal of Computer Engineering in Research Trends, vol. 11, no. 3, pp. 46-53, 2024.
[CrossRef] [Publisher Link]
[20] Maya O'Neil et al., “Development of a Publicly Available Database of Randomized Controlled Trials for Posttraumatic Stress Disorder: The PTSD-Repository,” Archives of Physical Medicine and Rehabilitation, vol. 101, no. 11, 2020.
[CrossRef] [Google Scholar] [Publisher Link]
[21] Parimal A. Itankar, and Hirendra R. Hajare, “Indoor Environment Navigation for Blind with Voice Feedback,” International Journal of Computer Engineering in Research Trends, vol. 3, no. 12, pp. 609-612, 2016.
[Google Scholar] [Publisher Link]
[22] M. Muzammil Parvez, H. Salam, and Y. Hoffmann, “Next-Generation Speech Analysis for Emotion Recognition and PTSD Detection with Advanced Machine and Deep Learning Models,” Synthesis: A Multidisciplinary Research Journal, vol. 1, no. 1, pp. 11-21, 2023.
[Publisher Link]
[23] Hussain Basha Pathan, Shyam Preeth, and M. Bhavsingh, “Revolutionizing PTSD Detection and Emotion Recognition through Novel Speech-Based Machine and Deep Learning Algorithms,” Frontiers in Collaborative Research, vol. 1, no. 1, pp. 35-44, 2023.
[Publisher Link]
[24] Vandana Singh, and Swati Prasad, “Speech Emotion Recognition Using Fully Convolutional Network and Augmented RAVDESS Dataset,” 2023 International Conference on Advanced Computing Technologies and Applications, Mumbai, India, pp. 1-7, 2023.
[CrossRef] [Google Scholar] [Publisher Link]
[25] Zhen-Tao Liu et al., “Speech Emotion Recognition Based on an Improved Brain Emotion Learning Model,” Neurocomputing, vol. 309, pp. 145-156, 2018.
[CrossRef] [Google Scholar] [Publisher Link]
[26] Samarth Tripathi, Sarthak Tripathi, and Homayoon Beigi, “Multi-Modal Emotion Recognition on IEMOCAP Dataset Using Deep Learning,” arXiv, pp. 1-5, 2018.
[CrossRef] [Google Scholar] [Publisher Link]