BORENET: Early Detection of Brain Tumor using RegNet and Classified using a Hybrid Dilated Network
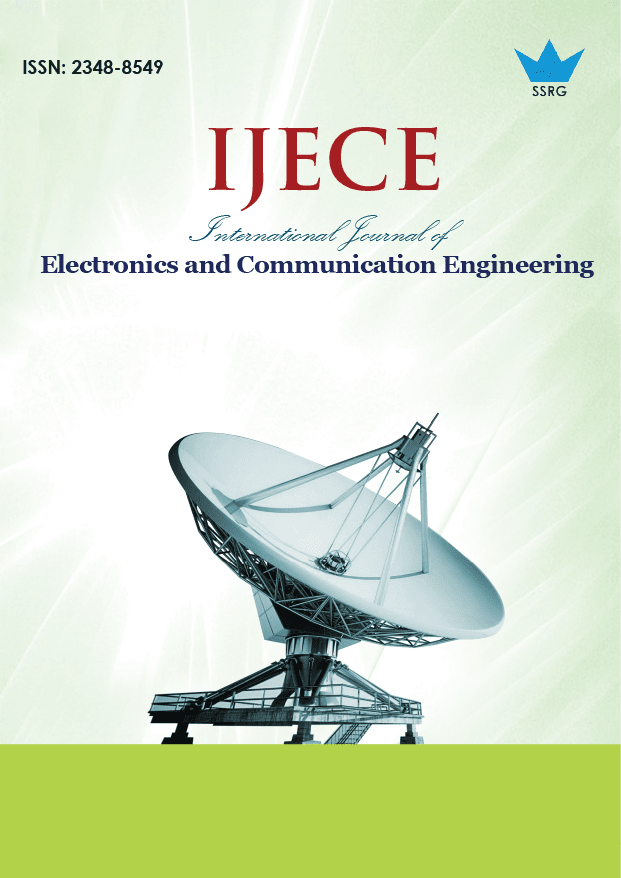
International Journal of Electronics and Communication Engineering |
© 2024 by SSRG - IJECE Journal |
Volume 11 Issue 5 |
Year of Publication : 2024 |
Authors : Gnanalakshmi. V, Shobana. G, Prema. G |
How to Cite?
Gnanalakshmi. V, Shobana. G, Prema. G, "BORENET: Early Detection of Brain Tumor using RegNet and Classified using a Hybrid Dilated Network," SSRG International Journal of Electronics and Communication Engineering, vol. 11, no. 5, pp. 244-252, 2024. Crossref, https://doi.org/10.14445/23488549/IJECE-V11I5P123
Abstract:
Brain tumor is a severe illness that affects humans. Detecting them early is vital for diagnosis and increasing the chances of survival. Brain tumors are one of the most severe forms of cancer, and they have caused the deaths of both children and adults in large numbers. Detecting brain tumors early through an MRI scan is essential for accurate diagnosis and treatment. MRI is the most widely used diagnostic technique for brain cancer, providing enhanced visibility of tumors to aid in subsequent treatment. Brain tumors must be accurately identified and predicted to ensure the best possible patient outcomes. Several issues can influence brain tumour classification, including poor image quality, insufficient training data, low-quality image characteristics, and poor tumor localization. In this work, a novel BORENET: Early Detection of Brain Tumor using RegNet and Classified using a Hybrid Dilated Network technique has been proposed to detect and categorize the types of tumors from the MRI image. Initially, the input image is pre-processed to increase its clarity, followed by feature extraction using a RegNet model to detect the presence of a tumor. Finally, a Hybrid Dilated CNN uses the collected features to categorize the tumor type as glioma, meningioma, or pituitary. Various evaluation Metrics like specificity, recall, accuracy, precision, and F1 score were used to assess the suggested BORENET model. The average classification accuracy for brain tumor detection and categorization is 99.86%. Compared to previous methods, the suggested strategy has proven to be extremely effective at detecting brain tumor. The BORENET model advances the overall accuracy by 2.72%, 0.96%, and 3.37% over the GCNN, TD-CNN-LSTM, and 3D CNN, respectively.
Keywords:
Brain tumor, RegNet, Gaussian adaptive filter, Hybrid dilated convolutional neural network, Deep learning.
References:
[1] Muthuvel Arumugam et al., “Crossover Smell Agent Optimized Multilayer Perceptron for Precise Brain Tumor Classification on MRI Images,” Expert Systems with Applications, vol. 238, 2024.
[CrossRef] [Google Scholar] [Publisher Link]
[2] Tapas Kumar Dutta, Deepak Ranjan Nayak, and Yu-Dong Zhang, “ARM-Net: Attention-Guided Residual Multiscale CNN for Multiclass Brain Tumor Classification Using MRI Images,” Biomedical Signal Processing and Control, vol. 87, 2024.
[CrossRef] [Google Scholar] [Publisher Link]
[3] N. Nagarani et al., “Self-Attention Based Progressive Generative Adversarial Network Optimized with Momentum Search Optimization Algorithm for Classification of Brain Tumor on MRI Image,” Biomedical Signal Processing and Control, vol. 88, 2024.
[CrossRef] [Google Scholar] [Publisher Link]
[4] Gopal S. Tandel et al., “A Review on a Deep Learning Perspective in Brain Cancer Classification,” Cancers, vol. 11, no. 1, pp. 1-32, 2019.
[CrossRef] [Google Scholar] [Publisher Link]
[5] D. Anand et al., “Identification of Meningioma Tumor using Recurrent Neural Networks,” Journal of Autonomous Intelligence, vol. 7, no. 2, pp. 1-7, 2024.
[CrossRef] [Google Scholar] [Publisher Link]
[6] Jaeyong Kang, Zahid Ullah, and Jeonghwan Gwak, “MRI-Based Brain Tumor Classification Using Ensemble of Deep Features and Machine Learning Classifiers,” Sensors, vol. 21, no. 6, pp. 1-21, 2021.
[CrossRef] [Google Scholar] [Publisher Link]
[7] Hassan Ali Khan et al., “Brain Tumor Classification in MRI Image Using Convolutional Neural Network,” Mathematical Biosciences and Engineering, vol. 17, no. 5, pp. 6203-6216, 2021.
[CrossRef] [Google Scholar] [Publisher Link]
[8] Arkapravo Chattopadhyay, and Mausumi Maitra, “MRI-Based Brain Tumour Image Detection Using CNN Based Deep Learning Method,” Neuroscience Informatics, vol. 2, no. 4, pp. 1-6, 2022.
[CrossRef] [Google Scholar] [Publisher Link]
[9] Emrah Irmak, “Multi-Classification of Brain Tumor MRI Images Using a Deep Convolutional Neural Network with a Fully Optimized Framework,” Iranian Journal of Science and Technology, Transactions of Electrical Engineering, vol. 45, no. 3, pp. 1015-1036, 2021.
[CrossRef] [Google Scholar] [Publisher Link]
[10] Md. Saikat Islam Khan et al., “Accurate Brain Tumor Detection Using Deep Convolutional Neural Network,” Computational and Structural Biotechnology Journal, vol. 20, pp. 4733-4745, 2022.
[CrossRef] [Google Scholar] [Publisher Link]
[11] Muhammad Aamir et al., “A Deep Learning Approach for Brain Tumor Classification Using MRI Images,” Computers and Electrical Engineering, vol. 101, 2022.
[CrossRef] [Google Scholar] [Publisher Link]
[12] Muhammad Rizwan et al., “Brain Tumor and Glioma Grade Classification Using Gaussian Convolutional Neural Network,” IEEE Access, vol. 10, pp. 29731-29740, 2022.
[CrossRef] [Google Scholar] [Publisher Link]
[13] Sidratul Montaha et al., “Time Distributed-CNN-LSTM: A Hybrid Approach Combining CNN and LSTM to Classify Brain Tumor on 3D MRI Scans Performing Ablation Study,” IEEE Access, vol. 10, pp. 60039-60059, 2022.
[CrossRef] [Google Scholar] [Publisher Link]
[14] Hiba Mzoughi et al., “Deep Multi-Scale 3D Convolutional Neural Network (CNN) for MRI Gliomas Brain Tumor Classification,” Journal of Digital Imaging, vol. 33, pp. 903-915, 2020.
[CrossRef] [Google Scholar] [Publisher Link]
[15] Hanaa M. Al Abboodi et al., “High-Resolution Model for Segmenting and Predicting Brain Tumor Based on Deep UNet with Multi Attention Mechanism,” International Journal of Intelligent Engineering & Systems, vol. 17, no. 2, pp. 294-310, 2024.
[CrossRef] [Google Scholar] [Publisher Link]