CSPBOT - boQIEKC: QoS Enhanced Integration OF Energy-Aware Clustering with Routing Protocol for WSN
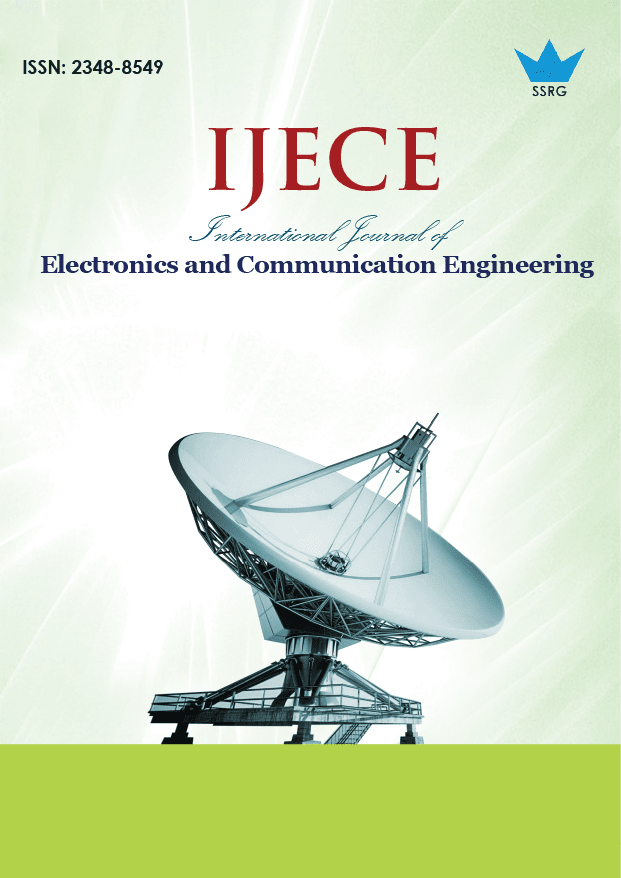
International Journal of Electronics and Communication Engineering |
© 2024 by SSRG - IJECE Journal |
Volume 11 Issue 6 |
Year of Publication : 2024 |
Authors : Venu Mangipudi, G. Aloy Anuja Mary |
How to Cite?
Venu Mangipudi, G. Aloy Anuja Mary, "CSPBOT - boQIEKC: QoS Enhanced Integration OF Energy-Aware Clustering with Routing Protocol for WSN," SSRG International Journal of Electronics and Communication Engineering, vol. 11, no. 6, pp. 40-52, 2024. Crossref, https://doi.org/10.14445/23488549/IJECE-V11I6P104
Abstract:
Nowadays, demanding ideals in Wireless Sensor Network (WSN) research are prolonging the network lifetime and lessening the energy consumption. However, the WSN with dynamic topology communication among Sensor Nodes (SNs) is liable to various attacks. SNs with low power may create some issues between security and energy consumption. In this proposed methodology, a protected energy-efficient routing approach, namely the Combinable Stochastic Patterned Bat Optimization Technique based on Quantized Indexive Energy Knowledgeable Clustering (CSPBOT -boQIEKC), is introduced. It performs SN clustering, shortest path identification, and path maintenance. Clustering and CH election are done by the Quantized Schutz Indexive Linde Buzo Gray (QSILBG) Algorithm. Meanwhile, the Cluster Head (CH) election is done by considering distance, residual energy of the SNs, and delay. Shortest path identification is performed by Combinable Stochastic Patterned Prevosti’s Bat Optimization (CSP2BO) Technique. Furthermore, the fitness of the bat is estimated based on the distance, energy, trust and link stability among SNs. In addition, the fault tolerance approach is utilized to ensure that the WSN continuously works regularly, even in the state of CH failure. The Stochastic Universal Sampling Selection (SUSS) procedure is activated to elect the best shortest path for data transmission. Finally, path maintenance is accomplished for alternative paths when link failure happens among SNs of the network. The performance of the proposed methodology is estimated on residual energy, energy consumption, packet delivery ratio, network lifetime and throughput. The proposed CSPBOT-boQIEKC technique provides an 18% improvement in energy consumption, 6% improvement in PDR, and 26% improvement in throughput than existing Taylor C-SSA, QEBSR and IoNM-BSOLyD approaches.
Keywords:
Energy efficiency, Clustering, Routing, Cluster head, Fault tolerance.
References:
[1] Syed Luqman Shah et al., “An Innovative Clustering Hierarchical Protocol for Data Collection from Remote Wireless Sensor Networks Based Internet of Things Applications,” Sensors, vol. 23, no. 12, pp. 1-26, 2023.
[CrossRef] [Google Scholar] [Publisher Link]
[2] Khushboo Jain, Anoop Kumar, and Akansha Singh, “Data Transmission Reduction Techniques for Improving Network Lifetime in Wireless Sensor Networks: An Upto Date Survey from 2017 to 2022,” Transactions on Emerging Telecommunications Technologies, vol. 34, no. 1, 2023.
[CrossRef] [Google Scholar] [Publisher Link]
[3] Satish R. Jondhale, R. Maheswar, and Jaime Lloret, Fundamentals of Wireless Sensor Networks, Received Signal Strength Based Target Localization and Tracking Using Wireless Sensor Networks, pp. 1-19, 2022.
[CrossRef] [Google Scholar] [Publisher Link]
[4] P. Paruthi Ilam Vazhuthi et al., “A Hybrid ANFIS Reptile Optimization Algorithm for Energy-Efficient Inter-Cluster Routing in Internet of Things-Enabled Wireless Sensor Networks,” Peer-to-Peer Networking and Applications, vol. 16, no. 2, pp. 1049-1068, 2023.
[CrossRef] [Google Scholar] [Publisher Link]
[5] Muhammad Amir Khan, and Adnan Anwar Awan, “Intelligent on Demand Clustering Routing Protocol for Wireless Sensor Networks,” Wireless Communications and Mobile Computing, vol. 2022, pp. 1-10, 2022.
[CrossRef] [Google Scholar] [Publisher Link]
[6] Divya Pandey, and Vandana Kushwaha, “A Trust-Based Security Aware Congestion Control Algorithm for Wireless Sensor Networks,” Adhoc & Sensor Wireless Networks, vol. 54, no. 3-4, pp. 249-290, 2022.
[CrossRef] [Google Scholar] [Publisher Link]
[7] Walid Osamy et al., “TACTIRSO: Trust Aware Clustering Technique Based on Improved Rat Swarm Optimizer for WSN-Enabled Intelligent Transportation System,” The Journal of Supercomputing, vol. 79, no. 6, pp. 5962-6016, 2023.
[CrossRef] [Google Scholar] [Publisher Link]
[8] Maha Ebrahim Al-Sadoon, Ahmed Jedidi, and Hamed Al-Raweshidy, “Dual-Tier Cluster-Based Routing in Mobile Wireless Sensor Network for IoT Application,” IEEE Access, vol. 11, pp. 4079-4094, 2023.
[CrossRef] [Google Scholar] [Publisher Link]
[9] A. Gautami, J. Shanthini, and S. Karthik, “A Quasi-Newton Neural Network Based Efficient Intrusion Detection System for Wireless Sensor Network,” Computer Systems Science & Engineering, vol. 45, no. 1, pp. 427-443, 2023.
[CrossRef] [Google Scholar] [Publisher Link]
[10] Chun Liu, and Liping Guo, “MMSCM: A Multiple Mobile Sinks Coverage Maximization Based Hierarchical Routing Protocol for Mobile Wireless Sensor Networks,” IET Communications, vol. 17, no. 10, pp. 1228-1242, 2023.
[CrossRef] [Google Scholar] [Publisher Link]
[11] Kamanashis Biswas et al., “A Multipath Routing Protocol for Secure Energy Efficient Communication in Wireless Sensor Networks,” Computer Networks, vol. 232, 2023.
[CrossRef] [Google Scholar] [Publisher Link]
[12] Changsun Shin, and Meonghun Lee, “Swarm-Intelligence-Centric Routing Algorithm for Wireless Sensor Networks,” Sensors, vol. 20, no. 18, pp. 1-13, 2020. [CrossRef] [Google Scholar] [Publisher Link]
[13] Chunlei Chen et al., “Deep Learning on Computational-Resource-Limited Platforms: A Survey,” Mobile Information Systems, pp. 1-19, 2020.
[CrossRef] [Google Scholar] [Publisher Link]
[14] Tianyi Zhang et al., “Seamless Clustering Multi-Hop Routing Protocol Based on Improved Artificial Bee Colony Algorithm,” EURASIP Journal on Wireless Communications and Networking, vol. 2020, no. 1, pp. 1-20, 2020.
[CrossRef] [Google Scholar] [Publisher Link]
[15] Vishal Kumar Arora, Vishal Sharma, and Monika Sachdeva, “A Multiple Pheromone Ant Colony Optimization Scheme for EnergyEfficient Wireless Sensor Networks,” Soft Computing, vol. 24, no. 1, pp. 543-553, 2020.
[CrossRef] [Google Scholar] [Publisher Link]
[16] Vishal Kumar Arora, Vishal Sharma, and Monika Sachdeva, “ACO Optimized Self-Organized Tree-Based Energy Balance Algorithm for Wireless Sensor Network: AOSTEB,” Journal of Ambient Intelligence and Humanized Computing, vol. 10, pp. 4963-4975, 2019.
[CrossRef] [Google Scholar] [Publisher Link]
[17] W.S. Kiran, S. Smys, and V. Bindhu, “Enhancement of Network Lifetime Using Fuzzy Clustering and Multidirectional Routing for Wireless Sensor Networks,” Soft Computing, vol. 24, no. 15, pp. 11805-11818, 2020.
[CrossRef] [Google Scholar] [Publisher Link]
[18] Akshay Verma et al., “Fuzzy Logic Based Effective Clustering of Homogeneous Wireless Sensor Networks for Mobile Sink,” IEEE Sensors Journal, vol. 20, no. 10, pp. 5615-5623, 2020.
[CrossRef] [Google Scholar] [Publisher Link]
[19] Indu Dohare, and Karan Singh, “Green Communication in Sensor Enabled IoT: Integrated Physics Inspired Meta-Heuristic Optimization Based Approach,” Wireless Networks, vol. 26, pp. 3331-3348, 2020.
[CrossRef] [Google Scholar] [Publisher Link]
[20] Xingjuan Cai et al., “A Unified Heuristic Bat Algorithm to Optimize the LEACH Protocol,” Concurrency and Computation: Practice and Experience, vol. 32, no. 9, 2020.
[CrossRef] [Google Scholar] [Publisher Link]
[21] Mohamed Elhoseny et al., “Swarm Intelligence–Based Energy Efficient Clustering with Multihop Routing Protocol for Sustainable Wireless Sensor Networks,” International Journal of Distributed Sensor Networks, vol. 16, no. 9, 2020.
[CrossRef] [Google Scholar] [Publisher Link]
[22] Prachi Maheshwari, Ajay K. Sharma, and Karan Verma, “Energy Efficient Cluster Based Routing Protocol for WSN Using Butterfly Optimization Algorithm and Ant Colony Optimization,” Ad Hoc Networks, vol. 110, 2021.
[CrossRef] [Google Scholar] [Publisher Link]
[23] G.A. Senthil, Arun Raaza, and N. Kumar, “Internet of Things Energy Efficient Cluster-Based Routing Using Hybrid Particle Swarm Optimization for Wireless Sensor Network,” Wireless Personal Communications, vol. 122, no. 3, pp. 2603-2619, 2022.
[CrossRef] [Google Scholar] [Publisher Link]
[24] Zongshan Wang et al., “Energy Efficient Cluster Based Routing Protocol for WSN Using Firefly Algorithm and Ant Colony Optimization,” Wireless Personal Communications, vol. 125, no. 3, pp. 2167-2200, 2022.
[CrossRef] [Google Scholar] [Publisher Link]
[25] K. Suresh et al., “F2SO: An Energy Efficient Cluster Based Routing Protocol Using Fuzzy Firebug Swarm Optimization Algorithm in WSN,” The Computer Journal, vol. 66, no. 5, pp. 1126-1138, 2023.
[CrossRef] [Google Scholar] [Publisher Link]
[26] Jaiswal, Kavita, and Veena Anand, “FAGWO-H: A Hybrid Method Towards Fault-Tolerant Cluster-Based Routing in Wireless Sensor Network for IoT Applications,” The Journal of Supercomputing, vol. 78, no. 8, pp. 11195-11227, 2022.
[CrossRef] [Google Scholar] [Publisher Link]
[27] Ishita Banerjee, and P. Madhumathy, “Qos Enhanced Energy Efficient Cluster Based Routing Protocol Realized Using Stochastic Modeling to Increase Lifetime of Green Wireless Sensor Network,” Wireless Networks, vol. 29, no. 2, pp. 489-507, 2022.
[CrossRef] [Google Scholar] [Publisher Link]
[28] A. Vinitha, M.S.S. Rukmini, and Dhirajsunehra, “Secure and Energy Aware Multi-Hop Routing Protocol in WSN Using Taylor-Based Hybrid Optimization Algorithm,” Journal of King Saud University-Computer and Information Sciences, vol. 34, no. 5, pp. 1857-1868, 2022.
[CrossRef] [Google Scholar] [Publisher Link]
[29] Manisha Rathee et al., “Ant Colony Optimization based Quality of Service Aware Energy Balancing Secure Routing Algorithm for Wireless Sensor Networks,” IEEE Transactions on Engineering Management, vol. 68, no. 1, pp. 170-182, 2019.
[CrossRef] [Google Scholar] [Publisher Link]