Energy Aware Dung Beetle Optimization-Based Clustering Scheme for Wireless Sensor Networks
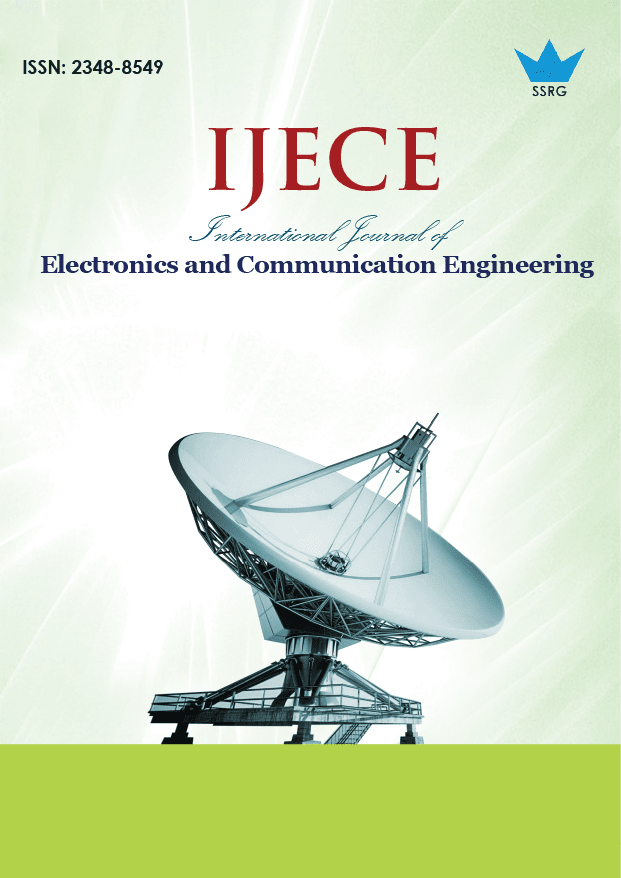
International Journal of Electronics and Communication Engineering |
© 2024 by SSRG - IJECE Journal |
Volume 11 Issue 6 |
Year of Publication : 2024 |
Authors : K. Nirmal, S. Murugan |
How to Cite?
K. Nirmal, S. Murugan, "Energy Aware Dung Beetle Optimization-Based Clustering Scheme for Wireless Sensor Networks," SSRG International Journal of Electronics and Communication Engineering, vol. 11, no. 6, pp. 53-62, 2024. Crossref, https://doi.org/10.14445/23488549/IJECE-V11I6P105
Abstract:
Wireless Sensor Networks (WSNs) have acquired considerable interest owing to their widespread applications in habitat tracking, healthcare, agriculture, disaster prevention, fire tracking, monitoring areas, etc. Extending the WSN lifespan is significant for diverse applications, and the most effective model is optimizing Cluster Heads (CHs) through clustering. The dynamic CH formation and energy-aware clustering scheme help to improve the WSN lifetime. This study introduces an EnergyAware Dung Beetle Optimization-based Clustering Scheme (EADBO-CS) for WSNs. The EADBO-CS technique intends to achieve energy efficiency by grouping the nodes into clusters and electing CHs. The EADBO-CS technique mainly follows the behavior of Dung Beetle (DB) populaces. To achieve this, the EADBO-CS technique designs a Fitness Function (FF) comprising various parameters, namely Residual Energy (RE), Distance to Neighbors (DTN), Distance to Base Station (DBS), Node Degree (ND), and Node Centrality (NC). The design of multiple parameters helps in achieving improved energy efficiency and maximum longevity in the WSN. An investigational analysis is performed to validate the improved achievement of the EADBO-CS technique. The simulation values signify the supremacy of the EADBO-CS method over other present approaches with minimal Energy Consumption (ECON) of 0.150mJ and maximum Throughput (THRO) of 98.22Mbps.
Keywords:
Energy efficiency, Cluster head selection, Metaheuristics, Wireless sensor network, Dung beetle optimization.
References:
[1] Shukun He et al., “The Optimization of Node Clustering and Multi-Hop Routing Protocol Using Hierarchical Chimp Optimization for Sustainable Energy Efficient Underwater Wireless Sensor Networks,” Wireless Networks, vol. 30, pp. 233-252, 2023.
[CrossRef] [Google Scholar] [Publisher Link]
[2] R. Anandkumar, P.V. Venkateswara Rao, and Nagendra Babu Rajaboina, “Hybrid Red Fox and Improved Whale Optimization AlgorithmBased Clustering Scheme for Improving Network Longevity in Wireless Sensor Networks,” Research Square, 2022.
[CrossRef] [Google Scholar] [Publisher Link]
[3] Venkatesh Sivaprakasam et al., “An Improved Coyote Optimization Algorithm-Based Clustering for Extending Network Lifetime in Wireless Sensor Networks,” KSII Transactions on Internet & Information Systems, vol. 17, no. 7, pp. 1873-1893, 2023.
[CrossRef] [Google Scholar] [Publisher Link]
[4] Richa Sharma, Vasudha Vashisht, and Umang Singh, “eeFFA/DE - A Fuzzy-Based Clustering Algorithm Using Hybrid Technique for Wireless Sensor Networks,” International Journal of Advanced Intelligence Paradigms, vol. 21, no. 1-2, pp. 129-157, 2022.
[CrossRef] [Google Scholar] [Publisher Link]
[5] Kavita Jaiswal, and Veena Anand, “A Grey-Wolf Based Optimized Clustering Approach to Improve QoS in Wireless Sensor Networks for IoT Applications,” Peer-to-Peer Networking and Applications, vol. 14, pp. 1943-1962, 2021.
[CrossRef] [Google Scholar] [Publisher Link]
[6] J. Samuel Manoharan, “A Metaheuristic Approach towards Enhancement of Network Lifetime in Wireless Sensor Networks,” KSII Transactions on Internet & Information Systems, vol. 17, no. 4, pp. 1276-1295, 2023.
[CrossRef] [Google Scholar] [Publisher Link]
[7] Zhigang Wang et al., “A Metaheuristic Algorithm for Coverage Enhancement of Wireless Sensor Networks,” Wireless Communications and Mobile Computing, pp. 1-23, 2022.
[CrossRef] [Google Scholar] [Publisher Link]
[8] Al-Ammari, and Ali Haider Mohammed, “Efficient Routing Scheme for Optimal Energy Consumptation in Wireless Sensor Network Using Metaheuristic Algorithm,” Master's Thesis, Altınbaş University/Graduate Education Institute, 2022.
[Google Scholar] [Publisher Link]
[9] Seema Swamy Gowda, and Ambika Ramalingappa, “Multi Objective Energy Based Improved Jellyfish Swarm Optimization for Effective Cluster Head Discovery in UWSN,” International Journal of Intelligent Engineering & Systems, vol. 16, no. 3, pp. 509-518, 2023.
[CrossRef] [Google Scholar] [Publisher Link]
[10] Narayanasamy Tamilarasan et al., “Hybrid Shuffled Frog Leaping and Improved Biogeography-Based Optimization Algorithm for Energy Stability and Network Lifetime Maximization in Wireless Sensor Networks,” International Journal of Communication Systems, vol. 34, no. 4, 2021.
[CrossRef] [Google Scholar] [Publisher Link]
[11] Sharmin Sharmin, Ismail Ahmedy, and Rafidah Md Noor, “An Energy-Efficient Data Aggregation Clustering Algorithm for Wireless Sensor Networks Using Hybrid PSO,” Energies, vol. 16, no. 5, pp. 1-24, 2023.
[CrossRef] [Google Scholar] [Publisher Link]
[12] Deyu Lin et al., “An Energy-Efficiency-Adaptive Clustering Formation Mechanism for the Wireless Sensor Networks,” IET Communications, vol. 16, no. 3, pp. 255-265, 2022.
[CrossRef] [Google Scholar] [Publisher Link]
[13] Baybars Karabekir, Muhammed Ali Aydın, and Abdül Halim Zaim, “Energy-Efficient Clustering-Based Mobile Routing Algorithm for Wireless Sensor Networks,” Electrica, vol. 21, no. 1, pp. 41-49, 2021.
[CrossRef] [Google Scholar] [Publisher Link]
[14] Ushus Elizebeth Zachariah, and Lakshmanan Kuppusamy, “A Hybrid Approach to Energy Efficient Clustering and Routing in Wireless Sensor Networks,” Evolutionary Intelligence, vol. 15, pp. 593-605, 2022.
[CrossRef] [Google Scholar] [Publisher Link]
[15] Nitin Kumar et al., “TEEECH: Three-Tier Extended Energy Efficient Clustering Hierarchy Protocol for Heterogeneous Wireless Sensor Network,” Expert Systems with Applications, vol. 216, 2023.
[CrossRef] [Google Scholar] [Publisher Link]
[16] Seyyit Alper Sert, and Adnan Yazici, “Increasing Energy Efficiency of Rule-Based Fuzzy Clustering Algorithms Using CLONALG-M for Wireless Sensor Networks,” Applied Soft Computing, vol. 109, 2021.
[CrossRef] [Google Scholar] [Publisher Link]
[17] G.A. Senthil, Arun Raaza, and N. Kumar, “Internet of Things Energy-Efficient Cluster-Based Routing using Hybrid Particle Swarm Optimization for Wireless Sensor Network,” Wireless Personal Communications, vol. 122, no. 3, pp. 2603-2619, 2022.
[CrossRef] [Google Scholar] [Publisher Link]
[18] Venkatasubramanian Srinivasan et al., “Detection of Data Imbalance in MANET Network Based on ADSY-AEAMBI-LSTM with DBO Feature Selection,” Journal of Autonomous Intelligence, vol. 7, no. 4, pp. 1-15, 2024.
[CrossRef] [Google Scholar] [Publisher Link]
[19] Mandli Rami Reddy et al., “Energy-Efficient Cluster Head Selection in Wireless Sensor Networks Using an Improved Grey Wolf Optimization Algorithm,” Computers, vol. 12, no. 2, pp. 1-17, 2023.
[CrossRef] [Google Scholar] [Publisher Link]
[20] Zijian Cai, Guolin Feng, and Qiguang Wang, “Based on the Improved PSO-TPA-LSTM Model Chaotic Time Series Prediction,” Atmosphere, vol. 14, no. 11, pp. 1-17, 2023.
[CrossRef] [Google Scholar] [Publisher Link]
[21] Prachi Maheshwari, Ajay K. Sharma, and Karan Verma, “Energy Efficient Cluster Based Routing Protocol for WSN Using Butterfly Optimization Algorithm and Ant Colony Optimization,” Ad Hoc Networks, vol. 110, 2021.
[CrossRef] [Google Scholar] [Publisher Link]
[22] Jeevanantham Vellaichamy et al., “Wireless Sensor Networks Based on Multi-Criteria Clustering and Optimal Bio-Inspired Algorithm for Energy-Efficient Routing,” Applied Sciences, vol. 13, no. 5, pp. 1-14, 2023.
[CrossRef] [Google Scholar] [Publisher Link]