Detection of Fake and Real Violence Using Hierarchical CNN Model
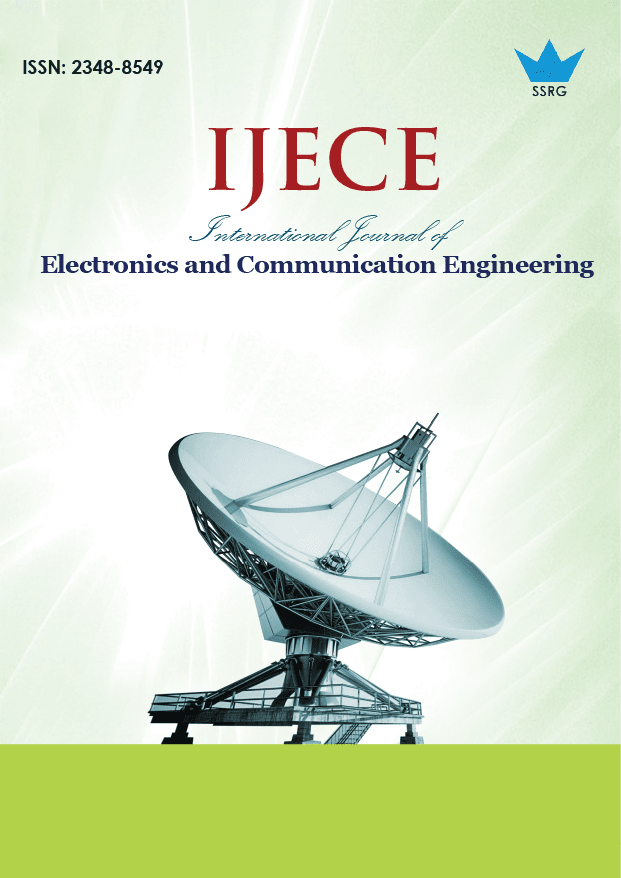
International Journal of Electronics and Communication Engineering |
© 2024 by SSRG - IJECE Journal |
Volume 11 Issue 6 |
Year of Publication : 2024 |
Authors : Lucky Rajpoot, Rosy Madaan |
How to Cite?
Lucky Rajpoot, Rosy Madaan, "Detection of Fake and Real Violence Using Hierarchical CNN Model," SSRG International Journal of Electronics and Communication Engineering, vol. 11, no. 6, pp. 114-121, 2024. Crossref, https://doi.org/10.14445/23488549/IJECE-V11I6P110
Abstract:
This investigation delves into the intersection of deep learning and image processing for early detection and classification of violence, with a primary focus on differentiating between movie fights (staged or fake) and true violence. Leveraging the "Violence and Non-violence Images Dataset," along with the collected movie fight images dataset, the proposed methodology involves Training Model3 (Hierarchal combination of Model1 and Model2). The hierarchy enhances performance and significantly improves specificity scores, even in a dataset biased toward nonviolence cases. The proposed model achieves an impressive accuracy of 98.33%, showcasing its potential for crime detection.
Keywords:
CNN, Domain Transfer Learning (DTL), Domain Data Augmentation (DDA), Deep learning, LSTM.
References:
[1] Marcella Papini et al., “The Role of Deep Learning Models in the Detection of Anti-Social Behaviours towards Women in Public Transport from Surveillance Videos: A Scoping Review,” Safety, vol. 9, no. 4, pp. 1-26, 2023.
[CrossRef] [Google Scholar] [Publisher Link]
[2] Viktor Huszar, “Possibilities and Challenges of the Defensive Use of Artificial Intelligence and Computer Vision Based Technologies and Applications in the Defence Sector,” Doctoral PhD Dissertation, pp. 1-21, 2023.
[Google Scholar]
[3] Md Golam Morshed et al., “Human Action Recognition: A Taxonomy-Based Survey, Updates, and Opportunities,” Sensors, vol. 23, no. 4, pp. 1-40, 2023.
[CrossRef] [Google Scholar] [Publisher Link]
[4] Ryo Hachiuma, Fumiaki Sato, and Taiki Sekii, “Unified Keypoint-Based Action Recognition Framework via Structured Keypoint Pooling,” 2023 IEEE/CVF Conference on Computer Vision and Pattern Recognition, Vancouver, BC, Canada, pp. 22962-22971, 2023.
[CrossRef] [Google Scholar] [Publisher Link]
[5] Elarbi Badidi, Karima Moumane, and Firdaous El Ghazi, “Opportunities, Applications, and Challenges of Edge-AI Enabled Video Analytics in Smart Cities: A Systematic Review,” IEEE Access, vol. 11, pp. 80543-80572, 2023.
[CrossRef] [Google Scholar] [Publisher Link]
[6] Nadia Mumtaz et al., “An Overview of Violence Detection Techniques: Current Challenges and Future Directions,” Artificial Intelligence Review, vol. 56, p. 4641-4666, 2023.
[CrossRef] [Google Scholar] [Publisher Link]
[7] Muhammad Awais, and Sara Durrani, “Violence Activity Detection Classification-A Review,” International Conference on Scientific and Academic Research, vol. 1, pp. 139-144, 2023.
[Publisher Link]
[8] Joelton Cezar Vieira et al., “Low-Cost CNN for Automatic Violence Recognition on Embedded System,” IEEE Access, vol. 10, p. 2519025202, 2022.
[CrossRef] [Google Scholar] [Publisher Link]
[9] Kristina Host, and Marina Ivasic-Kos, “An Overview of Human Action Recognition in Sports Based on Computer Vision,” Heliyon, vol. 8, no. 6, pp. 1-25, 2022.
[CrossRef] [Google Scholar] [Publisher Link]
[10] Fath U. Min Ullah et al., “AI-Assisted Edge Vision for Violence Detection in IoT-Based Industrial Surveillance Networks,” IEEE Transactions on Industrial Informatics, vol. 18, no. 8, pp. 5359-5370, 2022.
[CrossRef] [Google Scholar] [Publisher Link]
[11] Abbas Z. Kouzani, “Technological Innovations for Tackling Domestic Violence,” IEEE Access, vol. 11, pp. 91293-91311, 2023.
[CrossRef] [Google Scholar] [Publisher Link]
[12] Fei Wu et al., “A Survey on Video Action Recognition in Sports: Datasets, Methods and Applications,” IEEE Transactions on Multimedia, vol. 25, pp. 7943-7966, 2023.
[CrossRef] [Google Scholar] [Publisher Link]
[13] Yassine Himeur et al., “Video Surveillance Using Deep Transfer Learning and Deep Domain Adaptation: Towards Better Generalization,” Engineering Applications of Artificial Intelligence, vol. 119, pp. 1-34, 2023.
[CrossRef] [Google Scholar] [Publisher Link]
[14] Mujtaba Asad et al., “Multi-Frame Feature-Fusion-Based Model for Violence Detection,” The Visual Computer, vol. 37, pp. 1415-1431, 2021.
[CrossRef] [Google Scholar] [Publisher Link]
[15] Fernando J. Rendón-Segador et al., “Crimenet: Neural structured Learning Using Vision Transformer for Violence Detection,” Neural Networks, vol. 161, pp. 318-329, 2023.
[CrossRef] [Google Scholar] [Publisher Link]
[16] Fath U. Min Ullah et al., “An Intelligent System for Complex Violence Pattern Analysis and Detection,” International Journal of Intelligent Systems, vol. 37, pp. 10400-10422, 2022.
[CrossRef] [Google Scholar] [Publisher Link]
[17] Dalia Andrea Rodríguez et al., “A Systematic Review of Computer Science Solutions for Addressing Violence Against Women and Children,” IEEE Access, vol. 9, pp. 114622-114639, 2021.
[CrossRef] [Google Scholar] [Publisher Link]