Lung Cancer Detection Using Integration of Hybrid Segmentation Approach and DL Techniques
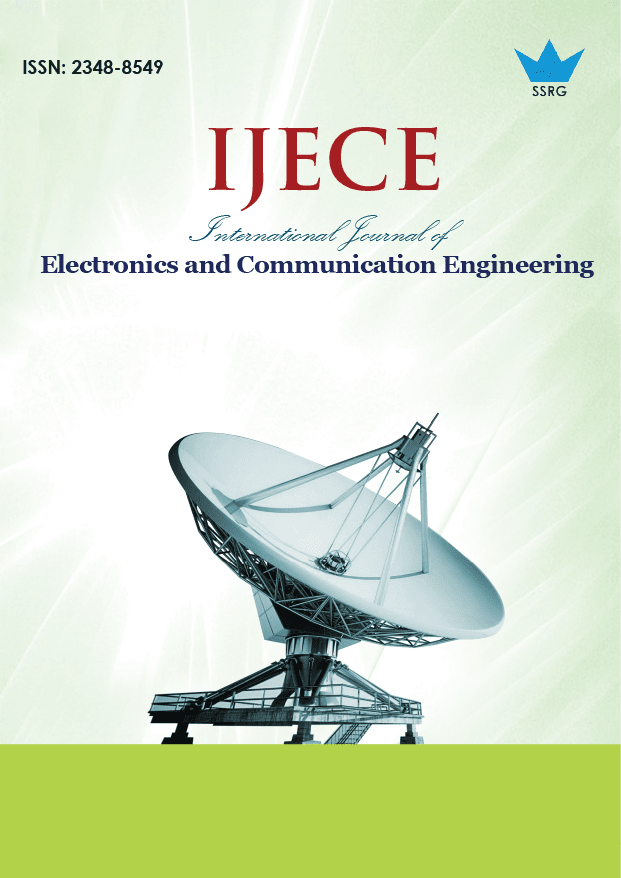
International Journal of Electronics and Communication Engineering |
© 2024 by SSRG - IJECE Journal |
Volume 11 Issue 6 |
Year of Publication : 2024 |
Authors : N. Raghapriya, Y. Kalpana |
How to Cite?
N. Raghapriya, Y. Kalpana, "Lung Cancer Detection Using Integration of Hybrid Segmentation Approach and DL Techniques," SSRG International Journal of Electronics and Communication Engineering, vol. 11, no. 6, pp. 148-157, 2024. Crossref, https://doi.org/10.14445/23488549/IJECE-V11I6P113
Abstract:
Cancer is a frequent illness with a rising death rate in recent years. Lung Cancer (LC) is a deadly illness that has a high patient death rate. Patients' lives can be saved by correctly determining the LC stage and receiving an early diagnosis of this illness. LC can be identified using a variety of image processing, biomarker-based, and machine automation techniques, however, medical professionals have difficulties in accurately and promptly diagnosing the disease. These automated detection systems currently use a diversity of Machine Learning (ML) methods to identify LC in its early stages. However, the processing of LC detection is time-consuming, and these systems do not offer reliable detection. This work proposes a hybrid segmentation approach that combines the Enhanced Kernal Fuzzy Clustering (EKFC) algorithm with the Global Particle Swarm Optimizer (GPSO) to carry out segmentation. CNN architecture is used to classify and extract features. The supplied image's categorization layer is responsible for identifying whether the tumor is abnormal or normal. In this work, the CT scan pictures are extracted using the lung imaging data that were acquired from the Kaggle website. The suggested segmentation methodology outperforms the other two segmentation approaches in the market with a Dice Index of 0.93. Furthermore, the Convolutional Neural Network (CNN) from the suggested segmented technique obtains 97.8% classification accuracy compared to the LSTM model.
Keywords:
CNN, CT images, Detection, EKFC, GPSO, Lung cancer.
References:
[1] Yu Gu et al., “ A Survey of Computer-Aided Diagnosis of Lung Nodules from CT Scans using DL, Computers in Biology and Medicine,” vol. 137, 2021.
[CrossRef] [Google Scholar] [Publisher Link]
[2] Muhammad Irfan Sharif et al., “ A Comprehensive Review on Multi-Organs Tumor Detection Based on Machine Learning, Pattern Recognition Letters,” vol. 131, pp. 30–37, 2020.
[CrossRef] [Google Scholar] [Publisher Link]
[3] Thamali Madhushani Adhikari et al., “ A Review of Deep Learning Techniques Applied in Lung Cancer Diagnosis, Signal and Information Processing, Networking and Computers,” Proceedings of the 6th International Conference on Signal and Information Processing, Networking and Computers, Guiyang, China, pp. 800–807, 2020.
[CrossRef] [Google Scholar] [Publisher Link]
[4] A. Asuntha, and Andy Srinivasan, “ Deep Learning for Lung Cancer Detection and Classification, Multimedia Tools and Applications,” vol. 79, pp. 7731–7762, 2020.
[CrossRef] [Google Scholar] [Publisher Link]
[5] P. Mohamed Shakeel, M.A. Burhanuddin, and Mohamad Ishak Des, “ Lung Cancer Detection from CT Image Using Improved Profuse Clustering and Deep Learning Instantaneously Trained Neural Networks, Measurement,” vol. 145, pp. 702–712, 2019.
[CrossRef] [Google Scholar] [Publisher Link]
[6] D. Jude Hemanth, Omer Deperlioglu, and Utku Kose, “ An Enhanced Dia etic Retinopathy Detection and Classification Approach Using Deep Convolutional Neural Network, Neural Computing and Applications,” vol. 32, pp. 707–721, 2020.
[CrossRef] [Google Scholar] [Publisher Link]
[7] Arun Singh Yadav et al., “ A Feature Extraction using Pro a ilistic Neural Network and BTFSC-Net Model with DL for Brain Tumor Classification,” Journal of Imaging, vol. 9, no. 1, pp. 1-22, 2023.
[CrossRef] [Google Scholar] [Publisher Link]
[8] Marko Savic et al., “ Lung Nodule Segmentation with a Region-Based Fast Marching Method, Sensors,” vol. 21, no. 5, pp. 1-32, 2021.
[CrossRef] [Google Scholar] [Publisher Link]
[9] Peter J. Mazzone et al., “ Screening for Lung Cancer, CHEST Guideline and Expert Panel Report, Thoracic Oncology: Guideline and Consensus Statement,” vol. 160, no. 5, pp. e427-e494, 2021.
[CrossRef] [Google Scholar] [Publisher Link]
[10] Hamid Mithoowani, and Michela Fe raro, “ Non-Small-Cell Lung Cancer in 2022: A Review for General Practitioners in Oncology,” Current Oncology, vol. 29, no. 3, pp. 1828-1839, 2022.
[CrossRef] [Google Scholar] [Publisher Link]
[11] Monica Ramakrishnan et al., “ Automated Lung Cancer Nodule Detection,” Thesis, Santa Clara University, pp. 1–37, 2022.
[Publisher Link]
[12] Radhanath Patra, “ Prediction of Lung Cancer using Machine Learning Classifier,” Computer Science, Communication and Security, pp. 132–142, 2020.
[CrossRef] [Google Scholar] [Publisher Link]
[13] Md Rashidul Hasan, and Muntasir Al Ka ir, “ Lung Cancer Detection and Classification ased on Image Processing and Statistical Learning,” Journal of Emerging Trends in Engineering and Applied Sciences, vol. 11, no. 6, pp. 229-236, 2020.
[Google Scholar] [Publisher Link]
[14] Raj D, Rekha BS, “ Detection and Classification of LC Using Image Processing Technique,” J Emerg Technol Innov Res, vol. 6, no. 5, 2019.
[15] Amirhossein Aghamohammadi et al., “ TPCNN: Two-Path Convolutional Neural Network for Tumor and Liver Segmentation in CT Images Using a Novel Encoding Approach,” Expert Systems with Applications, vol. 183, 2021.
[CrossRef] [Google Scholar] [Publisher Link]
[16] Saeid Jafarzadeh Ghoushchi et al., “ An Extended Approach to Predict Retinopathy in Diabetic Patients Using the Genetic Algorithm and Fuzzy C-Means, BioMed Research Internatioal,” pp. 1-13, 2021.
[CrossRef] [Google Scholar] [Publisher Link]
[17] M.O. Khairandish et al., “ A Hy rid CNN-SVM Threshold Segmentation Approach for Tumor Detection and Classification of MRI Brain Images,” IRBM, vol. 43, pp. 290–299, 2022.
[CrossRef] [Google Scholar] [Publisher Link]
[18] Ziyuan Yang, Lu Leng, and Byung-Gyu Kim, “ StoolNet for Color Classification of Stool Medical Images, Electronics,” vol. 8, no. 12, pp. 1–15, 2019.
[CrossRef] [Google Scholar] [Publisher Link]
[19] Yiqing Zhang et al., “ Mask-Refined R-CNN: A Network for Refining O ject Details In Instance Segmentation, Sensors,” vol. 20, no. 4, pp. 1–16, 2020.
[CrossRef] [Google Scholar] [Publisher Link]
[20] Yu Liu et al., “ Brain Tumor Segmentation in Multimodal MRI via Pixel-Level and Feature-Level Image Fusion, Frontiers in Neuroscience,” vol. 16, pp. 1-15, 2022.
[CrossRef] [Google Scholar] [Publisher Link]
[21] Muhammad Afridi et al., “ Brain Tumor Imaging: Applications of Artificial Intelligence, Seminars in Ultrasound, CT and MRI,” vol. 43, pp. 153–169, 2022.
[CrossRef] [Google Scholar] [Publisher Link]
[22] S. Sasikala, M. Bharathi, and B.R. Sowmiya, “ Lung Cancer Detection and Classification Using Deep CNN,” International Journal of Innovative Technology and Exploring Engineering, vol. 8, no. 2S, pp. 259-262, 2019.
[Google Scholar] [Publisher Link]
[23] S.R. Sannasi Chakravarthy, and Harikumar Rajaguru, “ Lung Cancer Detection using Probabilistic Neural Network with modified CrowSearch Algorithm,” Asian Pacific Journal of Cancer Prevention, vol. 20, no. 7, pp. 2159-2166, 2019.
[CrossRef] [Google Scholar] [Publisher Link]
[24] Matko Šarić et al., “ CNN- ased Method for LC Detection in Whole Slide Histopathology Images,” 4th International Conference on Smart and Sustainable Technologies (SpliTech), Split, Croatia, pp. 1-4, 2019.
[CrossRef] [Google Scholar] [Publisher Link]