A Bibliometric Study of Machine Learning in Precision Agriculture
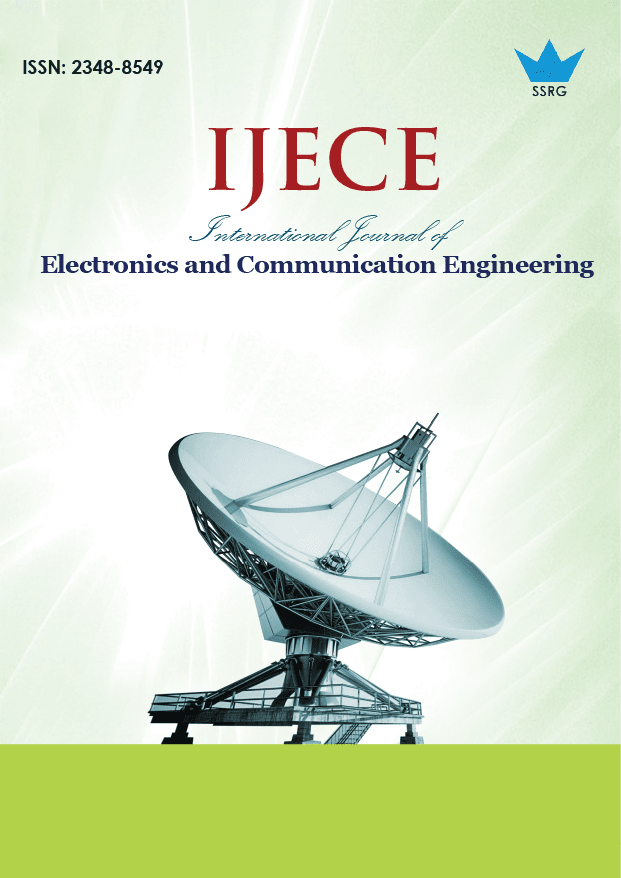
International Journal of Electronics and Communication Engineering |
© 2024 by SSRG - IJECE Journal |
Volume 11 Issue 6 |
Year of Publication : 2024 |
Authors : Mohamed Omar Abdullahi, Abdukadir Dahir Jimale, Yahye Abukar Ahmed, Abdulaziz Yasin Nageye |
How to Cite?
Mohamed Omar Abdullahi, Abdukadir Dahir Jimale, Yahye Abukar Ahmed, Abdulaziz Yasin Nageye, "A Bibliometric Study of Machine Learning in Precision Agriculture," SSRG International Journal of Electronics and Communication Engineering, vol. 11, no. 6, pp. 158-170, 2024. Crossref, https://doi.org/10.14445/23488549/IJECE-V11I6P114
Abstract:
Machine Learning (ML) has revolutionized precision agriculture, offering solutions to contemporary challenges in farming practices. This paper presents a comprehensive bibliometric analysis of ML applications in precision agriculture, leveraging the Scopus database and advanced visualization tools. Through quantitative and qualitative techniques, the study interprets key trends, influential publications, and emerging research areas within this interdisciplinary field. The analysis encompasses publication and citation trends, contributing countries, influential sources, authors, collaboration networks, thematic evolution, and trending topics. The findings highlight the growing significance of ML techniques in optimizing agricultural processes, enhancing sustainability, and fostering innovation. By providing a detailed understanding of the research landscape, this study enables stakeholders to identify emerging trends, foster collaborations, and advance the application of ML in agricultural practices.
Keywords:
Precision agriculture, Machine learning, Bibliometric analysis, Agricultural innovation, Collaborative networks, Thematic evolution.
References:
[1] Showkat Ahmad Bhat, and Nen-Fu Huang, “Big Data and AI Revolution in Precision Agriculture: Survey and Challenges,” IEEE Access, vol. 9, pp. 110209–110222, 2021.
[CrossRef] [Google Scholar] [Publisher Link]
[2] Ravesa Akhter, and Shabir Ahmad Sofi, “Precision Agriculture Using IoT Data Analytics and Machine Learning,” Journal of King Saud University - Computer and Information Sciences, vol. 34, no. 8, pp. 5602–5618, 2022.
[CrossRef] [Google Scholar] [Publisher Link]
[3] Solemane Coulibaly et al., “Deep Learning for Precision Agriculture: A Bibliometric Analysis,” Intelligent Systems with
Applications, vol. 16, pp. 1-18, 2022.
[CrossRef] [Google Scholar] [Publisher Link]
[4] Priya Rani Bhagat, Farheen Naz, and Robert Magda, “Artificial Intelligence Solutions Enabling Sustainable Agriculture: A Bibliometric Analysis,” PLoS One, vol. 17, no. 6, pp. 1-19, 2022.
[CrossRef] [Google Scholar] [Publisher Link]
[5] Justin Zuopeng Zhang et al., “Big Data Analytics and Machine Learning: A Retrospective Overview and Bibliometric Analysis,” Expert Systems with Applications, vol. 184, 2021.
[CrossRef] [Google Scholar] [Publisher Link]
[6] Lefteris Benos et al., “Machine Learning in Agriculture: A Comprehensive Updated Review,” Sensors, vol. 21, no. 11, pp. 1-55, 2021.
[CrossRef] [Google Scholar] [Publisher Link]
[7] Abhinav Sharma et al., “Machine Learning Applications for Precision Agriculture: A Comprehensive Review,” IEEE Access, vol. 9, pp. 4843–4873, 2021.
[CrossRef] [Google Scholar] [Publisher Link]
[8] Rambod Abiri et al., “Application of Digital Technologies for Ensuring Agricultural Productivity,” Heliyon, vol. 9, no. 12, pp. 1-21, 2023.
[CrossRef] [Google Scholar] [Publisher Link]
[9] Anna Chlingaryan, Salah Sukkarieh, and Brett Whelan, “Machine Learning Approaches for Crop Yield Prediction and Nitrogen Status Estimation in Precision Agriculture: A Review,” Computers and Electronics in Agriculture, vol. 151, pp. 61-69, 2018.
[CrossRef] [Google Scholar] [Publisher Link]
[10] Sharvari Patil, Nikhil Aklade, and Ashish Ashok Uikey, “Revolutionizing Vegetable Value Chains: A Comprehensive Review of Digital Technologies and their Impact on Agricultural Transformation,” Current Journal of Applied Science and Technology, vol. 42, no. 47, pp. 54–65, 2023.
[CrossRef] [Google Scholar] [Publisher Link]
[11] Abdukadir Dahir, Mohamed Omar, and Yahye Abukar, “Internet of things based agricultural drought detection system: case study Southern Somalia,” Bulletin of Electrical Engineering and Informatics, vol. 12, no. 1, pp. 69–74, 2023.
[CrossRef] [Google Scholar] [Publisher Link]
[12] Yonghong Tian, Bing Zheng, and Zeyu Li, “Agricultural Greenhouse Environment Monitoring System Based on Internet of Things,” 3rd IEEE International Conference on Computer and Communications, Chengdu, China, pp. 2981-2985, 2017.
[CrossRef] [Google Scholar] [Publisher Link]
[13] Sarah Condran et al., “Machine Learning in Precision Agriculture: A Survey on Trends, Applications and Evaluations over Two Decades,” IEEE Access, vol. 10, pp. 73786–73803, 2022.
[CrossRef] [Google Scholar] [Publisher Link]
[14] Abhijeet Madhukar Haval, and F. Rahman, “Application of Machine Learning Techniques and the Internet of Things for Smart, Sustainable Agriculture,” BIO Web of Conferences, EDP Sciences, vol. 82, pp. 1-11, 2024.
[CrossRef] [Google Scholar] [Publisher Link]
[15] Sara Oleiro Araújo et al., “Machine Learning Applications in Agriculture: Current Trends, Challenges, and Future Perspectives,” Agronomy, vol. 13, no. 12, pp. 1-27, 2023.
[CrossRef] [Google Scholar] [Publisher Link]
[16] Mohd Javaid et al., “Understanding the Potential Applications of Artificial Intelligence in Agriculture Sector,” Advanced Agrochem, vol. 2, no. 1, pp. 15–30, 2023.
[CrossRef] [Google Scholar] [Publisher Link]
[17] Sakshi Balyan et al., “Seeding a Sustainable Future: Navigating the Digital Horizon of Smart Agriculture,” Sustainability (Switzerland), vol. 16, no. 2, pp. 1-21, 2024.
[CrossRef] [Google Scholar] [Publisher Link]
[18] Kavery Ganguly, Ashok Gulati, and Joachim von Braun, “Innovations Spearheading the Next Transformations in India’s Agriculture,” 2017.
[CrossRef] [Google Scholar] [Publisher Link]
[19] Massimo Aria, and Corrado Cuccurullo, “Bibliometrix: An R-Tool for Comprehensive Science Mapping Analysis,” Journal of Informetrics, vol. 11, no. 4, pp. 959–975, 2017.
[CrossRef] [Google Scholar] [Publisher Link]
[20] Guozhu Mao et al., “Research on Biomass Energy and Environment from the Past to the Future: A Bibliometric Analysis,” Science of the Total Environment, vol. 635, pp. 1081–1090, 2018.
[CrossRef] [Google Scholar] [Publisher Link]
[21] Marcelo Werneck Barbosa, “Uncovering Research Streams on Agri-Food Supply Chain Management: A Bibliometric Study,” Global Food Security, vol. 28, 2021.
[CrossRef] [Google Scholar] [Publisher Link]
[22] Bipul Kumar et al., “Digital Mediation in Business-To-Business Marketing: A Bibliometric Analysis,” Industrial Marketing Management, vol. 85, pp. 126–140, 2020.
[CrossRef] [Google Scholar] [Publisher Link]
[23] B.M. Gupta, and S. Bhattacharya, “Bibliometric Approach towards Mapping the Dynamics of Science and Technology,” DESIDOC Bulletin of Information Technology, vol. 24, no. 1, pp. 3–8, 2004.
[CrossRef] [Google Scholar]
[24] Claudio A. Bonilla, José M. Merigó, and Carolina Torres-Abad, “Economics in Latin America: A Bibliometric Analysis,” Scientometrics, vol. 105, no. 2, pp. 1239–1252, 2015.
[CrossRef] [Google Scholar] [Publisher Link]
[25] Nees van Eck, and Ludo Waltman, “Software Survey: VOSviewer, A Computer Program for Bibliometric Mapping,” Scientometrics, vol. 84, no. 2, pp. 523–538, 2010.
[CrossRef] [Google Scholar] [Publisher Link]
[26] Hans W. Ittmann, “A Bibliometric Analysis of the Journal of Transport and Supply Chain Management,” Journal of Transport and Supply Chain Management, vol. 15, pp. 1-15, 2021.
[CrossRef] [Google Scholar] [Publisher Link]
[27] Jasman Tuyon et al., “Sustainable Financial Services: Reflection and Future Perspectives,” Journal of Financial Services Marketing, vol. 28, no. 4, pp. 664–690, 2023.
[CrossRef] [Google Scholar] [Publisher Link]
[28] Massimo Aria, Michelangelo Misuraca, and Maria Spano, “Mapping the Evolution of Social Research and Data Science on 30 Years of Social Indicators Research,” Social Indicators Research, vol. 149, no. 3, pp. 803–831, 2020.
[CrossRef] [Google Scholar] [Publisher Link]
[29] Judit Bar-Ilan, “Informetrics at the Beginning of the 21st Century - A Review,” Journal of Informetrics, vol. 2, no. 1. pp. 1–52, 2008.
[CrossRef] [Google Scholar] [Publisher Link]
[30] Matthew E. Falagas et al., “Comparison of PubMed, Scopus, Web of Science, and Google Scholar: Strengths and Weaknesses,” The FASEB Journal, vol. 22, no. 2, pp. 338–342, 2008.
[CrossRef] [Google Scholar] [Publisher Link]