Detecting the Possession of Harmful Weapons by Humans Through Surveillance System
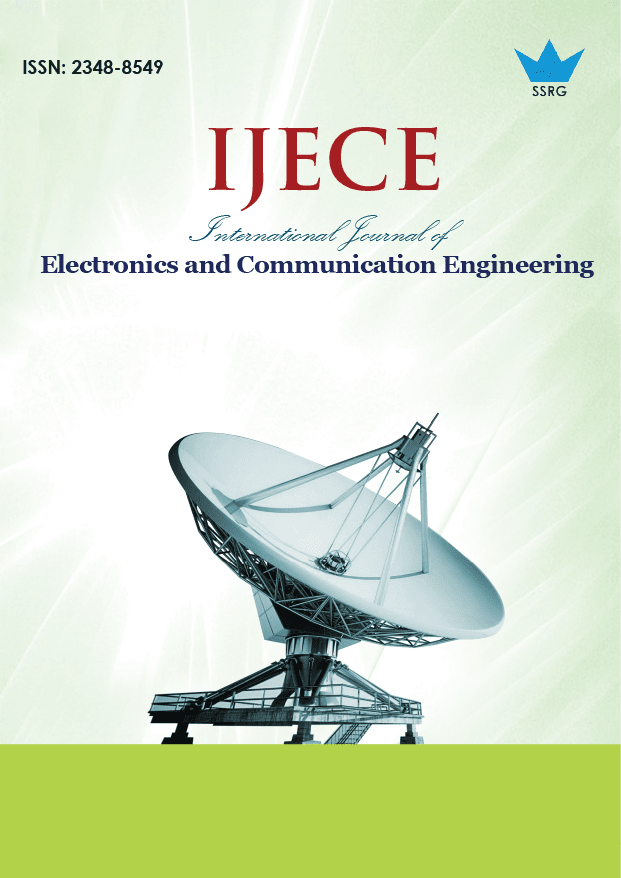
International Journal of Electronics and Communication Engineering |
© 2024 by SSRG - IJECE Journal |
Volume 11 Issue 7 |
Year of Publication : 2024 |
Authors : Nalini Manogaran, Suresh Annamalai, Malarvizhi Nandagopal, Koteeswaran Seerangan, Balamurugan Balusamy, Francesco Benedetto |
How to Cite?
Nalini Manogaran, Suresh Annamalai, Malarvizhi Nandagopal, Koteeswaran Seerangan, Balamurugan Balusamy, Francesco Benedetto, "Detecting the Possession of Harmful Weapons by Humans Through Surveillance System," SSRG International Journal of Electronics and Communication Engineering, vol. 11, no. 7, pp. 47-52, 2024. Crossref, https://doi.org/10.14445/23488549/IJECE-V11I7P105
Abstract:
Security Surveillance is a very tedious and time-consuming job. The system is to automate the task of analyzing video surveillance and alert systems. We will analyze the video feed in real-time and identify abnormal activities like gun and knife detection. There is much research going on in the industry about video surveillance, among them. The role of CCTV video has been overgrown, and CCTV cameras are placed all over the place for surveillance and security. The user gets notified for detecting the objects. It is crucial to proper surveillance for the safety and security of people and their assets. The libraries which have been used for detecting the object are TensorFlow, OpenCV, etc. The Convolutional Neural Network (CNN) is a deep learning algorithm that can take in an input image, assign importance to various aspects and objects in the image and be able to differentiate one from the other. The typical applications of deep surveillance are theft identification, violence detection, and detection of the chances of explosion.
Keywords:
Theft identification, Theft detection, Data processing, Object recognition, Security protocols.
References:
[1] Brad Alford, Edward Curran, and Shawn Cole, “Determining the Value of UAVs in Iraq,” The Journal of Conventional Weapons Destruction, vol. 22, no. 1, pp. 1-5, 2018.
[Google Scholar] [Publisher Link]
[2] Giuseppe Amato et al., “Towards Multimodal Surveillance for Smart Building Security,” Proceedings, vol. 2, no. 2, pp. 1-8, 2018.
[CrossRef] [Google Scholar] [Publisher Link]
[3] Pallavi S. Bangare et al., “The Online Home Security System: Ways to Protect Home from Intruders & Thefts,” International Journal of Innovative Technology and Exploring Engineering, vol. 2, no. 3, pp. 109-112, 2013.
[Google Scholar] [Publisher Link]
[4] J.S. Gadda and R.D. Patil, “Quad Copter (UAVS) for Border Security with GUI System,” International Journal of Engineering Research and Technology, vol. 2, no. 12, pp. 620-624, 2013.
[Google Scholar] [Publisher Link]
[5] Abhijit Mahalanobis et al., “Network Video Image Processing for Security, Surveillance, and Situational Awareness,” Proceedings of the SPIE - The International Society for Optical Engineering, FL, USA, vol. 5440, pp. 1-8, 2004.
[CrossRef] [Google Scholar] [Publisher Link]
[6] Mandar Shriram Munagekar, “Smart Surveillance System for Theft Detection Using Image Processing,” International Research Journal of Engineering and Technology, vol. 5, no. 8, pp. 232-234, 2018.
[Google Scholar] [Publisher Link]
[7] Srikar Banka et al., “Dynamic Based Face Authentication Using Video-Based Method,” International Journal of Computing and Digital Systems, vol. 10, pp. 1-9, 2020.
[Google Scholar]
[8] J. Rene Beulah et al., “Enhancing Detection of R2L Attacks by Multistage Clustering Based Outlier Detection,” Wireless Personal Communications, vol. 124, no. 3, pp. 2637-2659, 2022.
[CrossRef] [Google Scholar] [Publisher Link]
[9] J.B. Helonde et al., “EDCAM-Early Detection Congestion Avoidance Mechanism,” International Journal of Computer Application, vol. 7, no. 2, pp. 11-14, 2010.
[CrossRef] [Google Scholar] [Publisher Link]
[10] Jose L. Salazar González et al., “Real-Time Gun Detection in CCTV: An Open Problem,” Neural Networks, vol. 132, pp. 297-308, 2020.
[CrossRef] [Google Scholar] [Publisher Link]
[11] Fraol Gelana, and Arvind Yadav, “Firearm Detection from Surveillance Cameras Using Image Processing and Machine Learning Techniques,” Advances in Intelligent Systems and Computing, vol. 851, pp. 25-34, 2018.
[CrossRef] [Google Scholar] [Publisher Link]
[12] Azween Bin Abdullah et al., “Leather Image Quality Classification and Defect Detection System Using Mask R-CNN Model,” International Journal of Advanced Computer Science and Applications, vol. 15, no. 4, pp. 526-536, 2024.
[CrossRef] [Google Scholar] [Publisher Link]
[13] Muhammad Tahir Bhatti et al., “Weapon Detection in Real-Time CCTV Videos Using Deep Learning”, IEEE Access, vol. 9, pp. 34366-34382, 2021.
[CrossRef] [Google Scholar] [Publisher Link]
[14] Volkan Kaya, Servet Tuncer, and Ahmet Baran, “Detection and Classification of Different Weapon Types Using Deep Learning,” Applied Sciences, vol. 11 no. 16, pp. 1-13, 2021.
[CrossRef] [Google Scholar] [Publisher Link]