HR-NET: Revolutionizing Diabetic Retinopathy Diagnosis with High-Resolution Analysis
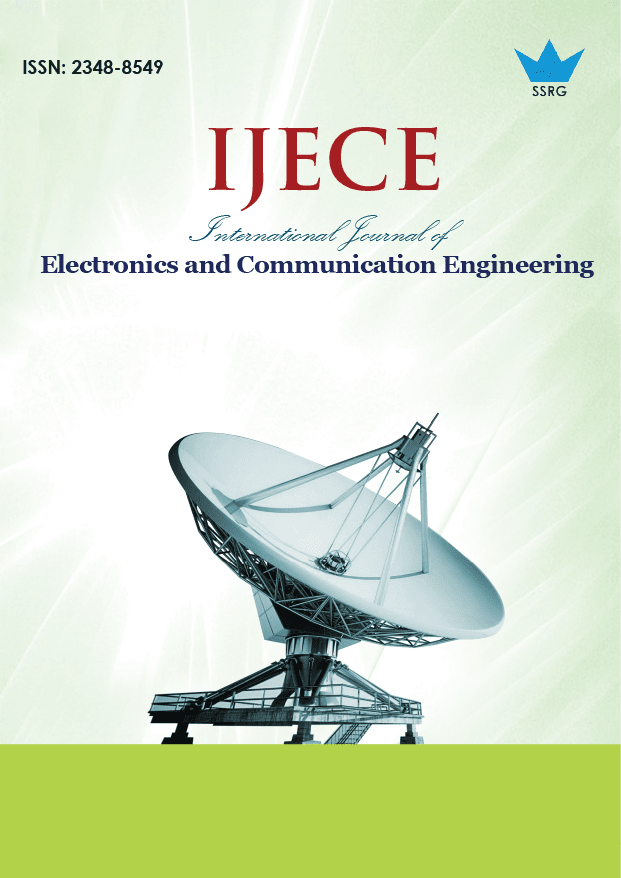
International Journal of Electronics and Communication Engineering |
© 2024 by SSRG - IJECE Journal |
Volume 11 Issue 7 |
Year of Publication : 2024 |
Authors : A. Jasni, I. Sowmy |
How to Cite?
A. Jasni, I. Sowmy, "HR-NET: Revolutionizing Diabetic Retinopathy Diagnosis with High-Resolution Analysis," SSRG International Journal of Electronics and Communication Engineering, vol. 11, no. 7, pp. 65-76, 2024. Crossref, https://doi.org/10.14445/23488549/IJECE-V11I7P107
Abstract:
One of the most prevalent consequences of diabetes and the primary cause of blindness globally is Diabetic Retinopathy, or DR. Early recognition of DR is vital for timely intervention and prevention of vision loss. However, existing methodologies for DR detection often suffer from limitations in accuracy and robustness. This study addresses these challenges by proposing a novel methodology for DR detection using the High-Resolution Network (HR-Net) architecture. The proposed approach begins with the collection of retinal images from a publicly available dataset and applies rigorous preprocessing as well as augmentation methods to boost the dataset's quality and diversity. The preprocessed images are then inputted into the HR-Net model, which leverages its unique architectural features, including high-resolution representation and multi-resolution fusion, to analyze retinal images effectively. Several performance measures are employed to assess the suggested model's effectiveness, and it is contrasted with previous models. Outcomes demonstrate that the proposed framework attains a remarkable accuracy of 95.67%, outperforming state-of-the-art methodologies. The significance of the proposed model lies in its ability to accurately detect DR-related abnormalities in retinal images, thereby facilitating early diagnosis and intervention. The study underscores the potential of HR-Net architecture in enhancing diabetic retinopathy diagnosis and treatment, offering promising prospects for improving clinical outcomes and reducing the burden of vision loss associated with DR.
Keywords:
Diabetic retinopathy, HR-Net, Retina, Deep Learning, Hemorrhages.
References:
[1] Alex Krizhevsky, Ilya Sutskever, and Geoffrey E. Hinton, “ImageNet Classification with Deep Convolutional Neural Networks,” Communications of the ACM, vol. 60, no. 6, pp. 84-90, 2017.
[CrossRef] [Google Scholar] [Publisher Link]
[2] David R. Whiting et al., “IDF Diabetes Atlas: Global Estimates of the Prevalence of Diabetes for 2011 and 2030,” Diabetes Research and Clinical Practice, vol. 94, no. 3, pp. 311-321, 2011.
[CrossRef] [Google Scholar] [Publisher Link]
[3] Diabetic Retinopathy, Mayoclinic. [Online]. Available: https://www.mayoclinic.org/diseases-conditions/diabetic-retinopathy/symptoms-causes/syc-20371611
[4] Shirin Hajeb Mohammad Alipour, Hossein Rabbani, and Mohammad Reza Akhlaghi, “Diabetic Retinopathy Grading by Digital Curvelet Transform,” Computational and Mathematical Methods in Medicine, vol. 2012, no. 1, pp. 1-11, 2012.
[CrossRef] [Google Scholar] [Publisher Link]
[5] Howard E. LeWine, Retinopathy, Harvard Medical School, 2023. [Online]. Available: https://www.health.harvard.edu/a_to_z/retinopathy-a-to-z
[6] Thippa Reddy Gadekallu et al., “Deep Neural Networks to Predict Diabetic Retinopathy,” Journal of Ambient Intelligence and Humanized Computing, vol. 14, pp. 5407-5420, 2023.
[CrossRef] [Google Scholar] [Publisher Link]
[7] Nagaraja Gundluru et al., “Enhancement of Detection of Diabetic Retinopathy Using Harris Hawks Optimization with Deep Learning Model,” Computational Intelligence and Neuroscience, vol. 2022, no. 1, pp. 1-13, 2022.
[CrossRef] [Google Scholar] [Publisher Link]
[8] Anas Bilal et al., “AI-Based Automatic Detection and Classification of Diabetic Retinopathy Using U-Net and Deep Learning,” Symmetry, vol. 14, no. 7, pp. 1-19, 2022.
[CrossRef] [Google Scholar] [Publisher Link]
[9] Wejdan L. Alyoubi, Maysoon F. Abulkhair, and Wafaa M. Shalash, “Diabetic Retinopathy Fundus Image Classification and Lesions Localization System Using Deep Learning,” Sensors, vol. 21, no. 11, pp. 1-22, 2021.
[CrossRef] [Google Scholar] [Publisher Link]
[10] Ping-Nan Chen et al., “General Deep Learning Model for Detecting Diabetic Retinopathy,” BMC Bioinformatics, vol. 22, pp. 1-14, 2021.
[CrossRef] [Google Scholar] [Publisher Link]
[11] Zubair Khan et al., “Diabetic Retinopathy Detection Using VGG-NIN a Deep Learning Architecture,” IEEE Access, vol. 9, pp. 61408- 61416, 2021.
[CrossRef] [Google Scholar] [Publisher Link]
[12] Borys Tymchenko, Philip Marchenko, and Dmitry Spodarets, “Deep Learning Approach to Diabetic Retinopathy Detection,” arXiv, pp. 1-9, 2020.
[CrossRef] [Google Scholar] [Publisher Link]
[13] Akhilesh Kumar Gangwar, and Vadlamani Ravi, “Diabetic Retinopathy Detection Using Transfer Learning and Deep Learning,” Evolution in Computational Intelligence, Advances in Intelligent Systems and Computing, vol. 1176, pp. 679-689, 2020.
[CrossRef] [Google Scholar] [Publisher Link]
[14] Imran Qureshi, Jun Ma, and Qaisar Abbas, “Diabetic Retinopathy Detection and Stage Classification in Eye Fundus Images Using Active Deep Learning,” Multimedia Tools and Applications, vol. 80, pp. 11691-11721, 2021.
[CrossRef] [Google Scholar] [Publisher Link]
[15] Silky Goel et al., “Deep Learning Approach for Stages of Severity Classification in Diabetic Retinopathy Using Color Fundus Retinal Images,” Mathematical Problems in Engineering, vol. 2021, no. 1, pp. 1-8, 2021.
[CrossRef] [Google Scholar] [Publisher Link]
[16] Gaurav Saxena et al., “Improved and Robust Deep Learning Agent for Preliminary Detection of Diabetic Retinopathy Using Public Datasets,” Intelligence-Based Medicine, vol. 3-4, pp. 1-11, 2020.
[CrossRef] [Google Scholar] [Publisher Link]
[17] Shu-I Pao et al., “Detection of Diabetic Retinopathy Using Bichannel Convolutional Neural Network,” Journal of Ophthalmology, vol. 2020, no. 1, pp. 1-7, 2020.
[CrossRef] [Google Scholar] [Publisher Link]
[18] Ganjar Alfian et al., “Deep Neural Network for Predicting Diabetic Retinopathy from Risk Factors,” Mathematics, vol. 8, no. 9, pp. 1-19, 2020.
[CrossRef] [Google Scholar] [Publisher Link]
[19] Feng Li et al., “Automatic Detection of Diabetic Retinopathy in Retinal Fundus Photographs Based on Deep Learning Algorithm,” Translational Vision Science & Technology, vol. 8, no. 6, pp. 1-13, 2019.
[CrossRef] [Google Scholar] [Publisher Link]
[20] Zhentao Gao et al., “Diagnosis of Diabetic Retinopathy Using Deep Neural Networks,” IEEE Access, vol. 7, pp. 3360-3370, 2019.
[CrossRef] [Google Scholar] [Publisher Link]
[21] OpenCV Library. [Online]. Available: https://opencv.org/
[22] Connor Shorten, and Taghi M. Khoshgoftaar, “A survey on Image Data Augmentation for Deep Learning,” Journal of Big Data, vol. 6, pp. 1-48, 2019.
[CrossRef] [Google Scholar] [Publisher Link]
[23] Jingdong Wang et al., “Deep High-Resolution Representation Learning for Visual Recognition,” IEEE Transactions on Pattern Analysis and Machine Intelligence, vol. 43, no. 10, pp. 3349-3364, 2021.
[CrossRef] [Google Scholar] [Publisher Link]
[24] NumPy. [Online]. Available: https://numpy.org/
[25] An End-to-End Platform for Machine Learning, Tensorflow. [Online]. Available: https://www.tensorflow.org/
[26] Scikit-Learn Machine Learning in Python, Scikit-Learn. [Online]. Available: https://scikit-learn.org/stable/