Path Loss Performance Analysis of Low Power IEEE 802.11af Secondary TV White Space Devices Based on Field Measurements
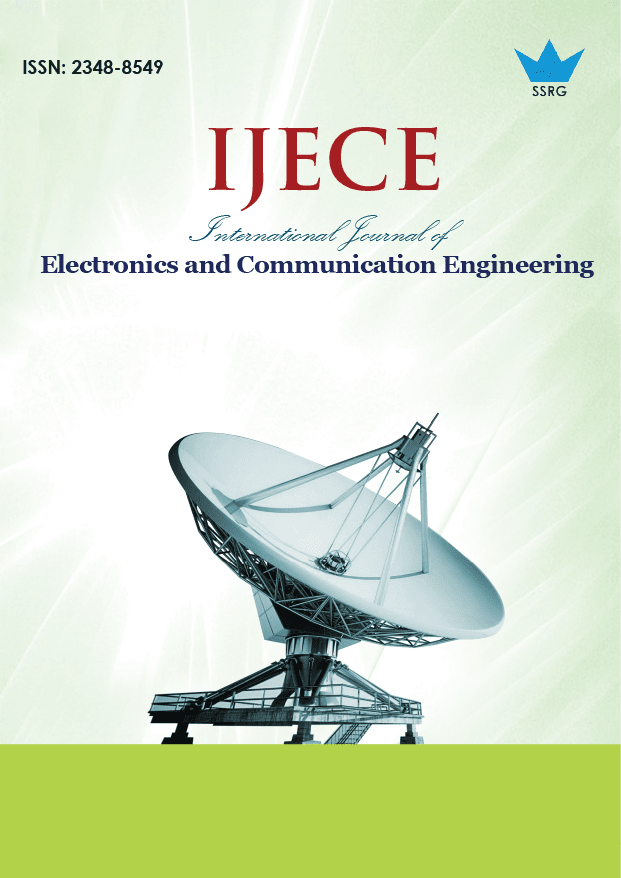
International Journal of Electronics and Communication Engineering |
© 2024 by SSRG - IJECE Journal |
Volume 11 Issue 7 |
Year of Publication : 2024 |
Authors : Alberto S. Banacia, Rosana J. Ferolin |
How to Cite?
Alberto S. Banacia, Rosana J. Ferolin, "Path Loss Performance Analysis of Low Power IEEE 802.11af Secondary TV White Space Devices Based on Field Measurements," SSRG International Journal of Electronics and Communication Engineering, vol. 11, no. 7, pp. 77-88, 2024. Crossref, https://doi.org/10.14445/23488549/IJECE-V11I7P108
Abstract:
The IEEE 802.11af standard is a wireless local area network that operates in the television white space, requiring it to avoid inducing damaging interference to licensed users such as incumbent digital and television services. To achieve this, precise tools for estimating path loss are essential to prevent both underestimation and overestimation, which could either limit the coverage of White Space Devices (WSDs) or fail to adequately shield primary or licensed users from unwanted emissions produced by secondary WSDs. This paper examines and compares the performance of various models for path loss, including Free Space Path Loss (FSPL), relevant portions of Recommendation ITU-R P.1411-11, and the linear and logarithmic regression models, against field measurement data to identify the most suitable path loss estimation model. The findings indicate that the logarithmic regression model exhibits the Root-Mean-Square Error (RMSE) that has the best performance, with a mean estimation error of 5 dB across all experimental locations where measurements were conducted. Additionally, the study suggests that the path loss model for free space can effectively provide a conservative path loss estimate for all sites, with an average overestimation of 18 dB, thereby ensuring adequate protection for primary users against potential interference from secondary users.
Keywords:
IEEE 802.11af, Path loss, TV white space.
References:
[1] Dynamic Spectrum Alliance: Worldwide Commercial Deployments, Pilots, & Trials, pp. 1-23, 2015. [Online]. Available: https://dynamicspectrumalliance.org/wp-content/uploads/2015/07/Pilots-and-Trials-Brochure_July-15.pdf
[2] “IEEE Standard for Information Technology - Telecommunications and Information Exchange between Systems - Local and Metropolitan Area Networks - Specific Requirement - Part 11: Wireless LAN Medium Access Control (MAC) and Physical Layer (PHY) Specifications Amendment 5: Television White Spaces (TVWS) Operation,” IEEE Std 802.11, 2014.
[Google Scholar]
[3] Kentaro Ishizu et al., “Field Experiment of Long-Distance Broadband Communications in TV White Space Using IEEE 802.22 and IEEE 802.11af,” 2014 International Symposium on Wireless Personal Multimedia Communications, Sydney, NSW, Australia, pp. 468-473, 2014.
[CrossRef] [Google Scholar] [Publisher Link]
[4] Antonio Montejo et al., “Implementation of a Multi-Hop Network at the University Campus Using an IEEE 802.11af-Compliant Network,” 2017 20th International Symposium on Wireless Personal Multimedia Communications, Bali, Indonesia, pp. 173-180, 2018.
[CrossRef] [Google Scholar] [Publisher Link]
[5] Jeric G. Brioso et al., “Vehicle to Vehicle Communications at Suburban Environment Using IEEE 802.11af Compliant Devices,” 2018 21st International Symposium on Wireless Personal Multimedia Communications, Chiang Rai, Thailand, pp. 85-89, 2018.
[CrossRef] [Google Scholar] [Publisher Link]
[6] Jeric G. Brioso et al., “Evaluation of IEEE 802.11af Compliant Devices for Vehicle to Infrastructure Communications in Suburban Environment,” 2018 IEEE Vehicular Networking Conference, Taipei, Taiwan, pp. 1-4, 2018.
[CrossRef] [Google Scholar] [Publisher Link]
[7] Tsuyoshi Shimomura, Teppei Oyama, and Hiroyuki Seki, “Analysis of TV White Space Availability in Japan,” 2012 IEEE Vehicular Technology Conference, Quebec City, QC, Canada, pp. 1-5, 2012.
[CrossRef] [Google Scholar] [Publisher Link]
[8] Theodore S. Rappaport, Wireless Communications: Principles and Practice, Prentice Hall PTR, pp. 1-707, 2002.
[Google Scholar] [Publisher Link]
[9] Hirokazu Sawada et al., “Path Loss and Throughput Estimation Models for an IEEE 802.11af Prototype,” 2015 IEEE 81st Vehicular Technology Conference, Glasgow, UK, pp. 1-5, 2015.
[CrossRef] [Google Scholar] [Publisher Link]
[10] Yee-Loo Foo, “Keep-Out Distance of the IEEE 802.11af System from Digital TV Broadcast Contour,” TENCON 2017 - 2017 IEEE Region 10 Conference, Penang, Malaysia, pp. 1185-1188, 2017.
[CrossRef] [Google Scholar] [Publisher Link]
[11] International Telecommunications Union, Radio Communications Bureau, “Recommendation ITUR P.1411-11: Propagation Data and Prediction Methods for the Planning of Short-Range Outdoor Radio Communication Systems and Radio Local Area Networks in the Frequency Range 300MHz to 100 GHz,” P Series, ITU Recommmendations, pp. 1-52, 2021.
[Google Scholar] [Publisher Link]
[12] P. Almorox-Gonzalez, and J.I. Alonso, “Software Tool for Planning Wireless Local Area Networks (WLAN),” The European Conference on Wireless Technology, Paris, France, pp. 387-390, 2005.
[CrossRef] [Google Scholar] [Publisher Link]
[13] Mingming Li, and Dongxu Wang, “Indoor Coverage Performance Comparison between IEEE 802.11g and IEEE 802.11 ah of Wireless Nodes in M2M Network,” Internet of Vehicles-Technologies and Services: First International Conference, Beijing, China, pp. 211-217, 2014.
[CrossRef] [Google Scholar] [Publisher Link]
[14] Siva Priya Thiagarajah et al., “An Investigation on the Use of ITU-R P.1411-7 in 802.11N Path Loss Modelling,” Progress In Electromagnetics Research Letters, vol. 50, pp. 91-98, 2014.
[CrossRef] [Google Scholar] [Publisher Link]
[15] Siva Priya Thiagarajah et al., “Applying ITU-R P.1411-7 Estimation for Urban 802.11N in Network Planning,” Progress In Electromagnetics Research Letters, vol. 54, pp. 55-59, 2015.
[CrossRef] [Google Scholar] [Publisher Link]
[16] Supachai Phaiboon, and Pisit Phokharatkul, “Multi-Boundary Empirical Path Loss Model for 433 MHz WSN in Agriculture Areas Using Fuzzy Linear Regression,” Sensors, vol. 23, no. 7, pp. 1-20, 2023.
[CrossRef] [Google Scholar] [Publisher Link]
[17] Yan Zhang et al., “Path Loss Prediction Based on Machine Learning: Principle, Method, and Data Expansion,” Applied Sciences, vol. 9, no. 9, pp. 1-18, 2019.
[CrossRef] [Google Scholar] [Publisher Link]
[18] Usman Sammani Sani, Owais Ahmed Malik, and Daphne Teck Ching Lai, “Improving Path Loss Prediction Using Environmental Feature Extraction from Satellite Images: Hand-Crafted vs. Convolutional Neural Network,” Applied Sciences, vol. 12, no. 15, pp. 1-24, 2022.
[CrossRef] [Google Scholar] [Publisher Link]
[19] Moamen Alnatoor, Mohammed Omari, and Mohammed Kaddi, “Path Loss Models for Cellular Mobile Networks Using Artificial Intelligence Technologies in Different Environments,” Applied Sciences, vol. 12, no. 24, pp. 1-23, 2022.
[CrossRef] [Google Scholar] [Publisher Link]
[20] Konstantinos Gatsis, “Linear Regression over Networks with Communication Guarantees,” Proceedings of the 3rd Conference on Learning for Dynamics and Control, vol. 144, pp. 767-778, 2021.
[Google Scholar] [Publisher Link]
[21] Yongjun Zhang, and Ling Ye, “Indoor Localization Method Based on AP and Local Linear Regression Algorithm,” 2017 IEEE 17th International Conference on Communication Technology, Chengdu, China, pp. 1122-1126, 2017.
[CrossRef] [Google Scholar] [Publisher Link]
[22] Liangping Ma et al., “Understanding Linear Regression for Wireless Sensor Network Time Synchronization,” pp. 325-328, 2007.
[Google Scholar]
[23] Manish Kumar Sahu, and Sunil Patil, “Linear Regression Based Power Optimization of Wireless Sensor Network in Smart City,” IOP Conference Series: Materials Science and Engineering, vol. 1085, no. 1, pp. 1-8, 2021.
[CrossRef] [Google Scholar] [Publisher Link]
[24] “IEEE Standard for Information Technology - Telecommunications and Information Exchange between Systems - Local and Metropolitan Area Networks - Part 11: Wireless LAN Medium Access Control (MAC) and Physical Layer (PHY) Specifications,” IEEE Computer Society, pp. 1-198, 2014.
[Google Scholar]
[25] Robert Kissell, and James Poserina, Optimal Sports Math, Statistics and Fantasy, Elsevier Science, pp. 1-352, 2017.
[Google Scholar] [Publisher Link]
[26] David Freedman, Statistical Models: Theory and Practice, Cambridge University Press, pp. 1-442, 2009.
[Google Scholar] [Publisher Link]
[27] John Fox, and Sanford Weisberg, An R Companion to Applied Regression, SAGE Publications, pp. 1-608, 2018.
[Google Scholar] [Publisher Link]