Deep Residual Network and Water Cloud Model-Based Soil Moisture Retrieval Using Satellite Images
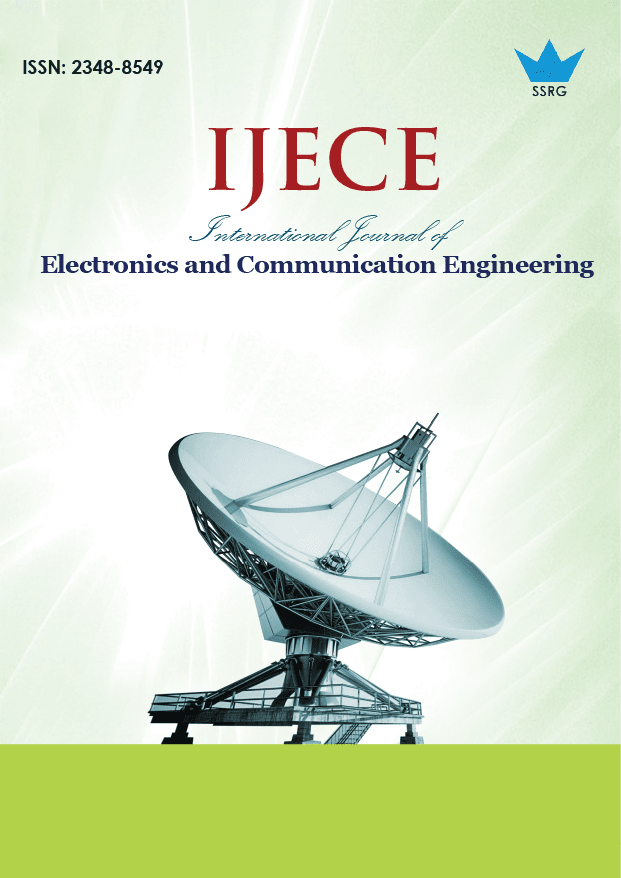
International Journal of Electronics and Communication Engineering |
© 2024 by SSRG - IJECE Journal |
Volume 11 Issue 7 |
Year of Publication : 2024 |
Authors : Sanjay B. Waykar, Rajesh Kadu, Amitkumar Manekar |
How to Cite?
Sanjay B. Waykar, Rajesh Kadu, Amitkumar Manekar, "Deep Residual Network and Water Cloud Model-Based Soil Moisture Retrieval Using Satellite Images," SSRG International Journal of Electronics and Communication Engineering, vol. 11, no. 7, pp. 89-97, 2024. Crossref, https://doi.org/10.14445/23488549/IJECE-V11I7P109
Abstract:
Soil moisture is a critical part of the link between land carbon cycle and surface-ground water motion. It also is vital in energy exchange between land/atmosphere; furthermore, soil moisture is an important determinant process for plant growth and productivity. By making the technique use satellite imagery, it is able to predict soil moisture content more accurately rather than using vegetation indices like the “Wide Dynamic Range Vegetation Index (WDRVI), Simple Ratio (SR), or Green Leaf Area Index (GLAI)”. A Deep Residual Network (DRN)-based “water cloud model “for soil moisture” forecasting is generated according to the vegetation indices. Additionally, soil moisture is derived using vegetation indices such as the “Wide Dynamic Range Vegetation Index (WDRVI), Simple Ratio (SR), and Green Leaf Area Index (GLAI)”. Performance of the developed DRNbased “water cloud model “was also evaluated in terms of RMSE and estimation error. The objective of this research work is to come up with a new modeling approach of soil moisture retrieval by improving “water cloud model “with satellite images and vegetation indices in one hand, and consequently to verify its performance through the application of Deep Residual Network (DRN)-based “water cloud model “on another hand. According to the experimental results, the suggested DRN-based “water cloud model “performed better than the others, with the smallest estimate error and Root Mean Square Error (RMSE) values of 0.523 and 0.69, respectively. The developed method’s estimation error is lower than that of the Semi-Empirical Water Cloud Model, Deep Multi Model Fusion Network, Genetic Algorithm (GA), Convolution Neural Network and Regression (CNNR), and Particle Swarm Optimization (PSO), respectively, at 94.14%, 65.32%, 91.63%, 63.12%, and 91.10%. The developed scheme’s RMSE is lower than that of the CNNR, PSO, Genetic Algorithm GA, Deep Multi Model Fusion Network, Semi Empirical Water Cloud Model, and 93.52%, 90.33%, 93.11%, and 89.72%, respectively.
Keywords:
Soil moisture, Satellite images, Water cloud model, Deep residual network, Vegetation index.
References:
[1] Kamal Kumar, K.S. Hari Prasad, and M.K. Arora, “Estimation of Water Cloud Model Vegetation Parameters Using a Genetic Algorithm,” Hydrological Sciences Journal, vol. 57, no. 4, pp. 776-789, 2012.
[CrossRef] [Google Scholar] [Publisher Link]
[2] S. Said, U.C. Kothyari, and M.K. Arora, “Vegetation Effects on Soil Moisture Estimation from ERS-2 SAR Images,” Hydrological Sciences Journal, vol. 57, no. 3, pp. 517-534, 2012.
[CrossRef] [Google Scholar] [Publisher Link]
[3] Grigorios Tsagkatakis, Mahta Moghaddam, and Panagiotis Tsakalides, “Deep Multi-Modal Satellite and in-Situ Observation Fusion for Soil Moisture Retrieval,” 2021 IEEE International Geoscience and Remote Sensing Symposium IGARSS, Brussels, Belgium, pp. 6339- 6342, 2021.
[CrossRef] [Google Scholar] [Publisher Link]
[4] Jian Liu et al., “Soil Moisture Retrieval in Farmland Areas with Sentinel Multi-Source Data Based on Regression Convolutional Neural Networks,” Sensors, vol. 21, no. 3, pp. 1-21, 2021.
[CrossRef] [Google Scholar] [Publisher Link]
[5] Sentinel-1 Dataset. [Online]. Available: https://scihub.copernicus.eu/dhus/#/home
[6] Landsat-8 OLI Dataset. [Online]. Available: https://earthexplorer.usgs.gov/
[7] Sonia I. Seneviratne et al., “Investigating Soil Moisture–Climate Interactions in A Changing Climate: A Review,” Earth-Science Reviews, vol. 99, no. 3-4, pp. 125-161, 2010.
[CrossRef] [Google Scholar] [Publisher Link]
[8] Kaighin A. McColl et al., “The Global Distribution and Dynamics of Surface Soil Moisture,” Nature Geoscience, vol. 10, no. 2, pp. 100- 104, 2017.
[CrossRef] [Google Scholar] [Publisher Link]
[9] S.U. Susha Lekshmi, D.N. Singh, and Maryam Shojaei Baghini, “A Critical Review of Soil Moisture Measurement,” Measurement, vol. 54, pp. 92-105, 2014.
[CrossRef] [Google Scholar] [Publisher Link]
[10] Venkat Lakshmi, “Remote Sensing of Soil Moisture,” International Scholarly Research Notices, 2013.
[CrossRef] [Google Scholar] [Publisher Link]
[11] Prashant K. Srivastava, “Satellite Soil Moisture: Review of Theory and Applications in Water Resources,” Water Resources Management, vol. 31, no. 10, pp. 3161-3176, 2017.
[CrossRef] [Google Scholar] [Publisher Link]
[12] Jian Peng et al., “Spatial Downscaling of Satellite Soil Moisture Data Using a Vegetation Temperature Condition Index,” IEEE Transactions on Geoscience and Remote Sensing, vol. 54, no. 1, pp. 558-566, 2015.
[CrossRef] [Google Scholar] [Publisher Link]
[13] M.E. Holzman, R. Rivas, and M.C. Piccolo, “Estimating Soil Moisture and the Relationship with Crop Yield Using Surface Temperature and Vegetation Index,” International Journal of Applied Earth Observation and Geoinformation, vol. 28, pp. 181-192, 2014.
[CrossRef] [Google Scholar] [Publisher Link]
[14] A. Bannari et al., “A Review of Vegetation Indices,” Remote Sensing Reviews, vol. 13, no. 1-2, pp. 95-120, 1995.
[CrossRef] [Google Scholar] [Publisher Link]
[15] Emmanouil Psomiadis et al., “The Role of Spatial and Spectral Resolution on the Effectiveness of Satellite-Based Vegetation Indices,” Remote Sensing for Agriculture, Ecosystems, and Hydrology XVIII, vol. 9998, pp. 509-521, 2016.
[CrossRef] [Google Scholar] [Publisher Link]
[16] Ali Ben Abbes, Ramata Magagi, and Kalifa Goita, “Soil Moisture Estimation from Smap Observations Using Long Short-Term Memory (LSTM),” IGARSS 2019 - 2019 IEEE International Geoscience and Remote Sensing Symposium, Yokohama, Japan, pp. 1590-1593, 2019.
[CrossRef] [Google Scholar] [Publisher Link]
[17] Shuai Huang et al., “Soil Moisture Retrival Based on Sentinel-1 Imagery under Sparse Vegetation Coverage,” Sensors, vol. 19, no. 3, pp. 1-18, 2019.
[CrossRef] [Google Scholar] [Publisher Link]
[18] Zhicong Chen et al., “Deep Residual Network-Based Fault Detection and Diagnosis of Photovoltaic Arrays Using Current-Voltage Curves and Ambient Conditions,” Energy Conversion and Management, vol. 198, 2019.
[CrossRef] [Google Scholar] [Publisher Link]
[19] Anil B. Gavade, and Vijay S. Rajpurohit, “Sparse-FCM and Deep Learning for Effective Classification of Land Area in Multi-Spectral Satellite Images,” Evolutionary Intelligence, pp. 1-17, 2020.
[CrossRef] [Google Scholar] [Publisher Link]
[20] Abhilash Singh et al., “Comparison of Different Dielectric Models to Estimate Penetration Depth of L-and S-Band SAR Signals Into the Ground Surface,” Geographies, vol. 2, no. 4, pp. 734-742, 2022.
[CrossRef] [Google Scholar] [Publisher Link]
[21] Prabhjot Kaur et al., “A Novel Transfer Deep Learning Method for Detection and Classification of Plant Leaf Disease,” Journal of Ambient Intelligence and Humanized Computing, vol. 14, pp. 12407-12424, 2022.
[CrossRef] [Google Scholar] [Publisher Link]
[22] Sijia Hao et al., “Model-Based Mechanism Analysis of “7.20” Flash Flood Disaster in Wangzongdian River Basin,” Water, vol. 15, no. 2, pp. 1-15, 2023.
[CrossRef] [Google Scholar] [Publisher Link]
[23] Mukund Pratap Singh, and Pitam Singh, “Multi-Criteria GIS Modeling for Optimum Route Alignment Planning in Outer Region of Allahabad City, India,” Arabian Journal of Geosciences, vol. 10, 2017.
[CrossRef] [Google Scholar] [Publisher Link]
[24] Mukund Pratap Singh, Pitam Singh, and Priyamvada Singh, “Fuzzy AHP-Based Multi-Criteria Decision-Making Analysis for Route Alignment Planning Using Geographic Information System (GIS),” Journal of Geographical Systems, vol. 21, pp. 395-432, 2019.
[CrossRef] [Google Scholar] [Publisher Link]
[25] Zhenghua Huang et al., “D3CNNs: Dual Denoiser Driven Convolutional Neural Networks for Mixed Noise Removal in Remotely Sensed Images,” Remote Sensing, vol. 15, no. 2, pp. 1-20, 2023.
[CrossRef] [Google Scholar] [Publisher Link]
[26] Abhilash Singh et al., “A Deep Learning Approach to Predict the Number of K-Barriers for Intrusion Detection over a Circular Region Using Wireless Sensor Networks,” Expert Systems with Applications, vol. 211, 2023.
[CrossRef] [Google Scholar] [Publisher Link]
[27] Mohsen Jamali et al., “Wheat Leaf Traits Monitoring Based on Machine Learning Algorithms and High-Resolution Satellite Imagery,” Ecological Informatics, vol. 74, 2023.
[CrossRef] [Google Scholar] [Publisher Link]
[28] M. Lavreniuk et al., “Reviewing Deep Learning Methods in the Applied Problems of Economic Monitoring Based on Geospatial Data,” Cybernetics and Systems Analysis, vol. 58, pp. 1008-1020, 2022.
[CrossRef] [Google Scholar] [Publisher Link]
[29] Yanling Wang et al., “A Comprehensive Study of Deep Learning for Soil Moisture Prediction,” Hydrology and Earth System Sciences, vol. 28, no. 4, pp. 917-943, 2024.
[CrossRef] [Google Scholar] [Publisher Link]
[30] Rong Fu et al., “A Soil Moisture Prediction Model, Based on Depth and Water Balance Equation: A Case Study of the Xilingol League Grassland,” International Journal of Environmental Research and Public Health, vol. 20, no. 2, pp. 1-18, 2023.
[CrossRef] [Google Scholar] [Publisher Link]
[31] Abhilash Singh, and Kumar Gaurav, “Deep Learning and Data Fusion to Estimate Surface Soil Moisture from Multi-Sensor Satellite Images,” Scientific Reports, vol. 13, pp. 1-20, 2023.
[CrossRef] [Google Scholar] [Publisher Link]