AudioStamp: A Deep Learning Based Watermarking Procedure for Copyright Protection of Digital Audio Files
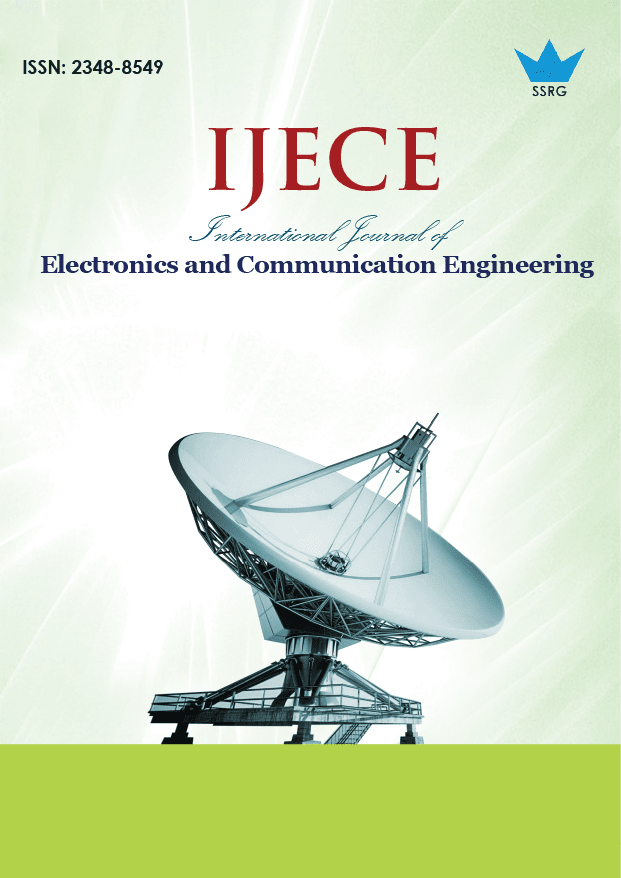
International Journal of Electronics and Communication Engineering |
© 2024 by SSRG - IJECE Journal |
Volume 11 Issue 7 |
Year of Publication : 2024 |
Authors : Abhijit Patil, Ramesh Shelke, Dilendra Hiran |
How to Cite?
Abhijit Patil, Ramesh Shelke, Dilendra Hiran, "AudioStamp: A Deep Learning Based Watermarking Procedure for Copyright Protection of Digital Audio Files," SSRG International Journal of Electronics and Communication Engineering, vol. 11, no. 7, pp. 108-116, 2024. Crossref, https://doi.org/10.14445/23488549/IJECE-V11I7P111
Abstract:
The ubiquitous use of digital media across various platforms has heightened the risk of copyright infringement and unauthorized distribution. Digital content such as images, audio, and video can be easily subjected to copyright violations if it is not adequately secured and protected using effective technological measures. In this paper, we explore different methods employed for safeguarding the copyright of digital media and propose a novel approach for copyright protection of audio files through the integration of watermarking techniques and neural networks. The proposed work concentrates on digital audio files. Our methodology leverages watermarking to embed ownership information or identifiers into audio files, ensuring their traceability and authenticity. Furthermore, we utilize neural networks, specifically encoder-decoder architecture, to enhance the robustness and security of the audio watermarking system. The primary objective of this innovative approach is to ensure robust protection of digital media without degrading the audio quality or clarity of embedded images. Utilizing sophisticated signal processing techniques, including wavelet transforms and denoising algorithms, the system embeds and subsequently reconstructs watermarked images within audio files with high fidelity. The goal is to strike an optimal balance between security and usability, providing content creators with a reliable method to safeguard their intellectual property. We evaluate the proposed method’s performance against critical parameters such as Maximum Correlation and Peak Signal-to-Noise Ratio (PSNR), among others. By training neural networks to embed watermarks imperceptibly and detect them accurately, we aim to provide a robust solution for copyright protection in the digital audio domain.
Keywords:
Copyright protection, Audio watermarking, Encoder-decoder, Deep Learning, Imperceptibility, Robustness.
References:
[1] Wanxing Huang, “Copyright Protection of Music Multimedia Works Fused with Digital Audio Watermarking Algorithm,” International Journal of Grid and High Performance Computing, vol. 15, no. 2, pp. 1-17, 2023.
[CrossRef] [Google Scholar] [Publisher Link]
[2] Chang Liu et al., “DeAR: A Deep-Learning-Based Audio Re-Recording Resilient Watermarking,” Proceedings of the AAAI Conference on Artificial Intelligence, vol. 37, no. 11, pp. 13201-13209, 2023.
[CrossRef] [Google Scholar] [Publisher Link]
[3] Kosta Pavlovic, Slavko Kovacevic, and Igor Durovic, “Speech Watermarking Using Deep Neural Networks,” 2020 28th Telecommunications Forum, Belgrade, Serbia, pp. 1-4, 2020.
[CrossRef] [Google Scholar] [Publisher Link]
[4] Xinghua Qu et al., “AudioQR: Deep Neural Audio Watermarks for QR Code,” Proceedings of the Thirty-Second International Joint Conference on Artificial Intelligence AI for Good, pp. 6192-6200, 2023.
[CrossRef] [Google Scholar] [Publisher Link]
[5] Amita Singha, and Muhammad Ahsan Ullah, “Development of an Audio Watermarking with Decentralization of the Watermarks,” Journal of King Saud University - Computer and Information Sciences, vol. 34, no. 6, pp. 3055-3061, 2022.
[CrossRef] [Google Scholar] [Publisher Link]
[6] Xiangyang Wang, Wei Qi, and Panpan Niu, “A New Adaptive Digital Audio Watermarking Based on Support Vector Regression,” IEEE Transactions on Audio, Speech, and Language Processing, vol. 15, no. 8, pp. 2270-2277, 2007.
[CrossRef] [Google Scholar] [Publisher Link]
[7] Maha Charfeddine et al., “Audio Watermarking for Security and Non-Security Applications,” IEEE Access, vol. 10, pp. 12654-12677, 2022.
[CrossRef] [Google Scholar] [Publisher Link]
[8] Margarita N. Favorskaya, and Andrey I. Pakhirka, “Adaptive HVS Objectivity-Based Watermarking Scheme for Copyright Protection,” Procedia Computer Science, vol. 192, pp. 1441-1450, 2021.
[CrossRef] [Google Scholar] [Publisher Link]
[9] Kasorn Galajit et al., “Semi-Fragile Speech Watermarking Based on Singular-Spectrum Analysis with CNN-Based Parameter Estimation for Tampering Detection,” APSIPA Transactions on Signal and Information Processing, vol. 8, pp. 1-13, 2019.
[CrossRef] [Google Scholar] [Publisher Link]
[10] Prayoth Kumsawat, Kitti Attakitmongcol, and Arthit Srikaew, “Digital Audio Watermarking for Copyright Protection Based on Multiwavelet Transform,” Intelligence and Security Informatics: European Conference, Esbjerg, Denmark, pp. 155-164, 2008.
[CrossRef] [Google Scholar] [Publisher Link]
[11] Zulfiqar Ali, Muhammad Imran, and Mansour Alsulaiman, “An Automatic Digital Audio Authentication/Forensics System,” IEEE Access, vol. 5, pp. 2994-3007, 2017.
[CrossRef] [Google Scholar] [Publisher Link]
[12] Chong Wang et al., “An Audio Watermarking Scheme with Neural Network,” Advances in Neural Networks - ISNN 2005: Second International Symposium on Neural Networks, Chongqing, China, pp. 795-800, 2005.
[CrossRef] [Google Scholar] [Publisher Link]
[13] Farah Deeba et al., “Digital Watermarking Using Deep Neural Network,” International Journal of Machine Learning and Computing, vol. 10, no. 2, pp. 277-282, 2020.
[CrossRef] [Google Scholar] [Publisher Link]
[14] Seyed Mostafa Pourhashemi, Mohammad Mosleh, and Yousof Erfani, “A Novel Audio Watermarking Scheme Using Ensemble-Based Watermark Detector and Discrete Wavelet Transform,” Neural Computing and Applications, vol. 33, no. 11, pp. 6161-6181, 2021.
[CrossRef] [Google Scholar] [Publisher Link]
[15] Yassine Himeur, and Bachir Boudraa, “Secure and Robust Audio Watermarking System for Copyright Protection,” 2012 24th International Conference on Microelectronics, Algiers, Algeria, pp. 1-4, 2012.
[CrossRef] [Google Scholar] [Publisher Link]
[16] Zihan Wang et al., “Data Hiding with Deep Learning: A Survey Unifying Digital Watermarking and Steganography,” IEEE Transactions on Computational Social Systems, vol. 10, no. 6, pp. 2985-2999, 2023.
[CrossRef] [Google Scholar] [Publisher Link]
[17] Muhammad Rasyid Redha Ansori et al., “HADES: Hash-Based Audio Copy Detection System for Copyright Protection in Decentralized Music Sharing,” IEEE Transactions on Network and Service Management, vol. 20, no. 3, pp. 2845-2853, 2023.
[CrossRef] [Google Scholar] [Publisher Link]
[18] Lukas Tegendal, “Watermarking in Audio Using Deep Learning,” Master Thesis, Linköping University, pp. 1-50, 2019.
[Google Scholar] [Publisher Link]
[19] Farida Aboelezz, “Watermarking Audio Files with Copyrights,” Bachelor Thesis, the German University in Cairo, pp. 1-82, 2022.
[Google Scholar]
[20] Preeti Garg, and R. Rama Kishore, “An Efficient and Secured Blind Image Watermarking Using ABC Optimization in DWT and DCT Domain,” Multimedia Tools and Applications, vol. 81, pp. 36947-37964, 2022.
[CrossRef] [Google Scholar] [Publisher Link]
[21] Saoussen Ben Jabra, and Mohamed Ben Farah, “Deep Learning-Based Watermarking Techniques Challenges: A Review of Current and Future Trends,” Circuits, Systems, Signal Process, vol. 43, pp. 4339-4368, 2024.
[CrossRef] [Google Scholar] [Publisher Link]
[22] Farnaz Arab, Salwani Mohd Daud, and Siti Zaiton Hashim, “Discrete Wavelet Transform Domain Techniques,” 2013 International Conference on Informatics and Creative Multimedia, Kuala Lumpur, Malaysia, pp. 340-345, 2013.
[CrossRef] [Google Scholar] [Publisher Link]
[23] Numpy.correlate, Numpy. [Online]. Available: https://numpy.org/doc/stable/reference/generated/numpy.correlate.html
[24] 50K Celebrity Faces Image Dataset, Kaggle. [Online]. Available: https://www.kaggle.com/datasets/farzadnekouei/50k-celebrity-faces-image-dataset
[25] Nguyen Chi Sy, Ha Hoang Kha, and Nguyen Minh Hoang, “An Efficient Robust Blind Watermarking Method Based on Convolution Neural Networks in Wavelet Transform Domain,” International Journal Machine Learning Computing, vol. 10, no. 5, pp. 675-684, 2020.
[CrossRef] [Google Scholar] [Publisher Link]
[26] N.V. Lalitha, Ch. Srinivasa Rao, and P.V.Y. Jaya Sree, “Localization of Copy-Move Forgery in Speech Signals through Watermarking Using DCT-QIM,” International Journal of Electronics and Telecommunications, vol. 65, no. 3, pp. 527-532, 2019.
[CrossRef] [Google Scholar] [Publisher Link]
[27] Arashdeep Kaur, Malay Kishore Dutta, and Jiri Prinosil, “General Regression Neural Network Based Audio Watermarking Algorithm Using Torus Automorphism,” 2018 41st International Conference on Telecommunications and Signal Processing, Athens, Greece, pp. 1- 4, 2018.
[CrossRef] [Google Scholar] [Publisher Link]
[28] Hadi Latifpour, Mohammad Mosleh, and Mohammad Kheyrandish, “An Intelligent Audio Watermarking Based on KNN Learning Algorithm,” International Journal of Speech Technology, vol. 18, pp. 697-706, 2015.
[CrossRef] [Google Scholar] [Publisher Link]