Ensemble Machine Learning for Classification of Autism Spectrum Disorder in Toddlers and Adults
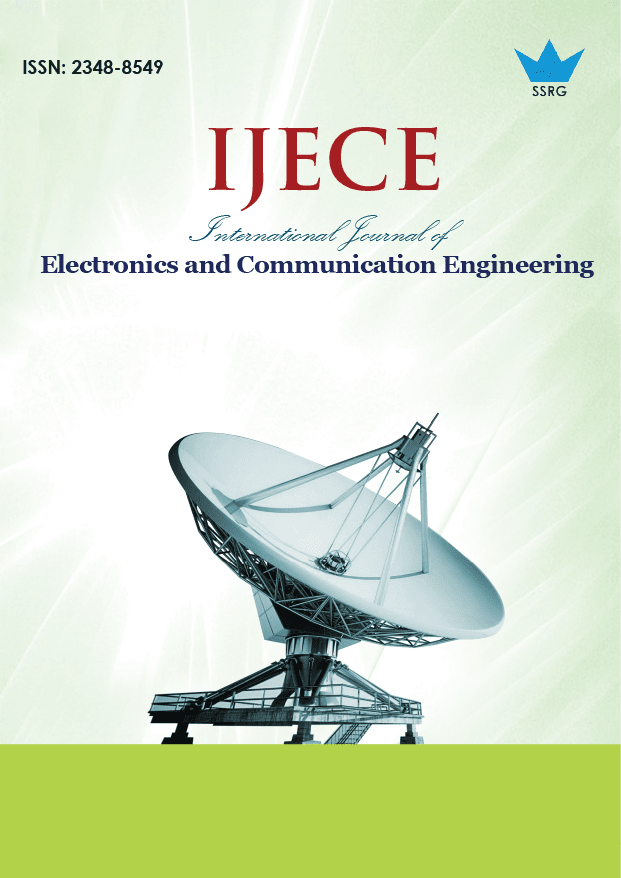
International Journal of Electronics and Communication Engineering |
© 2024 by SSRG - IJECE Journal |
Volume 11 Issue 7 |
Year of Publication : 2024 |
Authors : Yogita Dubey, Atharva Patrikar, Padmesh Kimmatkar, Prajwal Dehankar, Punit Fulzele |
How to Cite?
Yogita Dubey, Atharva Patrikar, Padmesh Kimmatkar, Prajwal Dehankar, Punit Fulzele, "Ensemble Machine Learning for Classification of Autism Spectrum Disorder in Toddlers and Adults," SSRG International Journal of Electronics and Communication Engineering, vol. 11, no. 7, pp. 117-127, 2024. Crossref, https://doi.org/10.14445/23488549/IJECE-V11I7P112
Abstract:
Autism Spectrum Disorder (ASD) is a neurological disorder. A Person with ASD always faces challenges in communicating socially and is involved in repetitive behaviors. Accurate and timely diagnosis is essential for efficient assistance and action. This paper presents the ensemble machine learning methodology to classify ASD in both toddlers and adults. Four distinct algorithms, Gradient Boosting (GB), Histogram Boosting (HB), Extreme Gradient Boosting (XGB) and Adaptive Boosting (ADB), are used. Explorative data analysis is performed to show the impact of behavioural features and individual characteristics on ASD in toddlers and adults. Quantitative analysis demonstrates that XGB outperforms with classification accuracy, log loss and F1-score, precision and recall. The findings indicate that the ensemble machine learning methodology has great potential to improve the diagnostic procedures for ASD, possibly resulting in an earlier and more accurate diagnosis of the condition.
Keywords:
Ensemble machine learning, Boosting algorithms, Autism classification, Parametric analysis, Quantitative assessment, Toddlers and Adults.
References:
[1] Fadi Thabtah, Neda Abdelhamid, and David Peebles, “A Machine Learning Autism Classification Based on Logistic Regression Analysis,” Health Information Science and Systems, vol. 7, 2019.
[CrossRef] [Google Scholar] [Publisher Link]
[2] Ugur Erkan, and Dang N.H. Thanh, “Autism Spectrum Disorder Detection with Machine Learning Methods,” Current Psychiatry Research and Reviews Formerly: Current Psychiatry Reviews, vol. 15, no. 4, pp. 297-308, 2019.
[CrossRef] [Google Scholar] [Publisher Link]
[3] Tania Akter et al., “Machine Learning-Based Models for Early Stage Detection of Autism Spectrum Disorders,” IEEE Access, vol. 7, pp. 166509-166527, 2019.
[CrossRef] [Google Scholar] [Publisher Link]
[4] Milan N. Parikh, Hailong Li, and Lili He, “Enhancing Diagnosis of Autism with Optimized Machine Learning Models and Personal Characteristic Data,” Frontiers in Computational Neuroscience, vol. 13, pp. 1-5, 2019.
[CrossRef] [Google Scholar] [Publisher Link]
[5] Fadi Thabtah, and David Peebles, “A New Machine Learning Model Based on Induction of Rules for Autism Detection,” Health Informatics Journal, vol. 26, no. 1, pp. 264-286, 2020.
[CrossRef] [Google Scholar] [Publisher Link]
[6] Fadi Thabtah, “Machine Learning in Autistic Spectrum Disorder Behavioral Research: A Review and Ways Forward,” Informatics for Health and Social Care, vol. 44, no. 3, pp. 278-297, 2019.
[CrossRef] [Google Scholar] [Publisher Link]
[7] Elizabeth Stevens et al., “Identification and Analysis of Behavioral Phenotypes in Autism Spectrum Disorder via Unsupervised Machine Learning,” International Journal of Medical Informatics, vol. 129, pp. 29-36, 2019.
[CrossRef] [Google Scholar] [Publisher Link]
[8] Fadi Thabtah, “An Accessible and Efficient Autism Screening Method for Behavioural Data and Predictive Analyses,” Health Informatics Journal, vol. 25, no. 4, pp. 1739-1755, 2019.
[CrossRef] [Google Scholar] [Publisher Link]
[9] Naseer Ahmed Khan et al., “A Three-Stage Teacher, Student Neural Networks and Sequential Feed Forward Selection-Based Feature Selection Approach for the Classification of Autism Spectrum Disorder,” Brain Sciences, vol. 10, no. 10, pp. 1-22, 2020.
[CrossRef] [Google Scholar] [Publisher Link]
[10] Irene Chaidi, and Athanasios Drigas, “Autism, Expression, and Understanding of Emotions: Literature Review,” International Journal of Online and Biomedical Engineering, vol. 16, no. 2, pp. 94-111, 2020.
[CrossRef] [Google Scholar] [Publisher Link]
[11] Mokhlesur Rahman et al., “A Review of Machine Learning Methods of Feature Selection and Classification for Autism Spectrum Disorder,” Brain Sciences, vol. 10, no. 12, pp. 1-23, 2020.
[CrossRef] [Google Scholar] [Publisher Link]
[12] Yuanrui Zheng, Tingyan Deng, and Yaozheng Wang, “Autism Classification Based on Logistic Regression Model,” 2021 IEEE 2nd International Conference on Big Data, Artificial Intelligence and Internet of Things Engineering, Nanchang, China, pp. 579-582, 2021.
[CrossRef] [Google Scholar] [Publisher Link]
[13] R. Sujatha et al., “A Machine Learning Way to Classify Autism Spectrum Disorder,” International Journal of Emerging Technologies in Learning, vol. 16, no. 6, pp. 182-200, 2021.
[CrossRef] [Google Scholar] [Publisher Link]
[14] Kaushik Vakadkar, Diya Purkayastha, and Deepa Krishnan, “Detection of Autism Spectrum Disorder in Children Using Machine Learning Techniques,” SN Computer Science, vol. 2, pp. 1-9, 2021.
[CrossRef] [Google Scholar] [Publisher Link]
[15] Nurul Amirah Mashudi, Norulhusna Ahmad, and Norliza Mohd Noor, “Classification of Adult Autistic Spectrum Disorder Using Machine Learning Approach,” IAES International Journal of Artificial Intelligence, vol. 10, no. 3, pp. 743-751, 2021.
[CrossRef] [Google Scholar] [Publisher Link]
[16] Md Delowar Hossain et al., “Detecting Autism Spectrum Disorder Using Machine Learning Techniques,” Health Information Science and Systems, vol. 9, 2021.
[CrossRef] [Google Scholar] [Publisher Link]
[17] Muhammad Kashif Hanif et al., “Employing Machine Learning-Based Predictive Analytical Approaches to Classify Autism Spectrum Disorder Types,” Complexity, vol. 2022, no. 1, pp. 1-10, 2022.
[CrossRef] [Google Scholar] [Publisher Link]
[18] Mousumi Bala et al., “Efficient Machine Learning Models for Early Stage Detection of Autism Spectrum Disorder,” Algorithms, vol. 15, no. 5, pp. 1-22, 2022.
[CrossRef] [Google Scholar] [Publisher Link]
[19] Mengyi Liao, Hengyao Duan, and Guangshuai Wang, “Application of Machine Learning Techniques to Detect the Children with Autism Spectrum Disorder,” Journal of Healthcare Engineering, vol. 2022, no. 1, pp. 1-10, 2022.
[CrossRef] [Google Scholar] [Publisher Link]
[20] Muhammad Shoaib Farooq et al., “Detection of Autism Spectrum Disorder (ASD) in Children and Adults Using Machine Learning,” Scientific Reports, vol. 13, pp. 1-13, 2023.
[CrossRef] [Google Scholar] [Publisher Link]
[21] Dhuha Dheyaa Khudhur, and Saja Dheyaa Khudhur, “The Classification of Autism Spectrum Disorder by Machine Learning Methods on Multiple Datasets for Four Age Groups,” Measurement: Sensors, vol. 27, pp. 1-6, 2023.
[CrossRef] [Google Scholar] [Publisher Link]
[22] Chelsea M. Parlett-Pelleriti et al., “Applications of Unsupervised Machine Learning in Autism Spectrum Disorder Research: A Review,” Review Journal of Autism and Developmental Disorders, vol. 10, pp. 406-421, 2023.
[CrossRef] [Google Scholar] [Publisher Link]
[23] Roberta Simeoli et al., “Using Machine Learning for Motion Analysis to Early Detect Autism Spectrum Disorder: A Systematic Review,” Review Journal of Autism and Developmental Disorders, pp. 1-20, 2024.
[CrossRef] [Google Scholar] [Publisher Link]
[24] D. Aarthi, and S. Kannimuthu, “A Comprehensive Analysis of Autism Spectrum Disorder Using Machine Learning Algorithms: Survey,” Proceedings of Power Engineering and Intelligent Systems, vol. 1, pp. 241-253, 2023.
[CrossRef] [Google Scholar] [Publisher Link]
[25] S. Jayanthi et al., “Mental Health Status Monitoring for People with Autism Spectrum Disorder Using Machine Learning,” International Journal of Information Technology, vol. 16, pp. 43-51, 2023.
[CrossRef] [Google Scholar] [Publisher Link]
[26] Fadi, Autism Screening Data for Toddlers, Kaggle, 2018.
[Google Scholar] [Publisher Link]
[27] Faizunnabi, Autism Screening, Kaggle. [Online]. Available: https://www.kaggle.com/datasets/faizunnabi/autism-screening
[28] Fadi Thabtah, “Autism Spectrum Disorder Screening: Machine Learning Adaptation and DSM-5 Fulfillment,” Proceedings of the 1 st International Conference on Medical and Health Informatics, Taichung City, Taiwan, pp. 1-6, 2017.
[CrossRef] [Google Scholar] [Publisher Link]
[29] Jerome H. Friedman, “Greedy Function Approximation: A Gradient Boosting Machine,” Annals of Statistics, vol. 29, no. 5, pp. 1189- 1232, 2001.
[CrossRef] [Google Scholar] [Publisher Link]
[30] Tianqi Chen, and Carlos Guestrin, “XGBoost: A Scalable Tree Boosting System,” Proceedings of the 22nd ACM SIGKDD International Conference on Knowledge Discovery and Data Mining, San Francisco, California, USA, pp. 785-794, 2016.
[CrossRef] [Google Scholar] [Publisher Link]
[31] Yoav Freund, and Robert E Schapire, “A Decision-Theoretic Generalization of On-Line Learning and an Application to Boosting,” Journal of Computer and System Sciences, vol. 55, no. 1, pp. 119-139, 1997.
[CrossRef] [Google Scholar] [Publisher Link]
[32] Guolin Ke et al., “LightGBM: A Highly Efficient Gradient Boosting Decision Tree,” Proceedings of the 31st International Conference on Neural Information Processing Systems, pp. 3149-3157, 2017.
[Google Scholar] [Publisher Link]