Exploring Hybrid GRU-LSTM Networks for Enhanced Music Generation
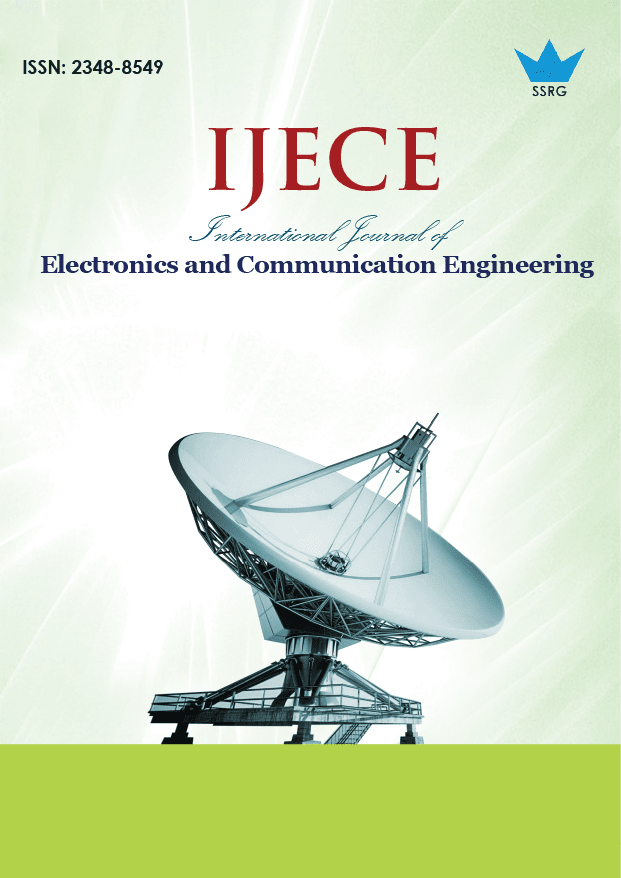
International Journal of Electronics and Communication Engineering |
© 2024 by SSRG - IJECE Journal |
Volume 11 Issue 7 |
Year of Publication : 2024 |
Authors : Suman Maria Tony, S. Sasikumar |
How to Cite?
Suman Maria Tony, S. Sasikumar, "Exploring Hybrid GRU-LSTM Networks for Enhanced Music Generation," SSRG International Journal of Electronics and Communication Engineering, vol. 11, no. 7, pp. 150-162, 2024. Crossref, https://doi.org/10.14445/23488549/IJECE-V11I7P115
Abstract:
The use of deep learning for creating music has been receiving a lot of interest nowadays due to its capacity for innovation and originality. This paper investigates how well hybrid networks that combine Gated Recurrent Units (GRU) and Long Short-Term Memory (LSTM) units perform music-generating tasks. Improving the model's ability to identify long-term relationships and maintain context while generating musical sequences is the aim of the proposed hybrid architecture. It accomplishes this by fusing the benefits of LSTM and GRU units. Comprehensive experiments are conducted on multiple music datasets to evaluate the performance of the hybrid GRU-LSTM networks in generating musical compositions. The quality of created music sequences is evaluated using performance measures like overall musicality, harmonic consistency, and melody coherence. Expert musicians also conduct qualitative assessments to offer insights into the creative and artistic elements of the developed compositions. When compared to current LSTM-based models, the results show how well the hybrid GRU-LSTM networks perform in generating high-quality music sequences with better coherence, consistency, and inventiveness. Furthermore, the study investigates the effects of various coaching strategies and design features on the performance of hybrid networks. All things considered, by exploring novel architectures and strategies for applying deep learning techniques to enhance the uniqueness and quality of generated music, this research enhances the field of music creation. The findings shed light on how hybrid GRU-LSTM networks might encourage innovation in the sector and raise the bar for music production skills.
Keywords:
Creativity, Deep Learning, Gated Recurrent Units, Hybrid networks, Harmonic consistency, Long Short-Term Memory, Music composition, Melody coherence, Quality assessment.
References:
[1] Shuyu Li, and Yunsick Sung, “MRBERT: Pre-Training of Melody and Rhythm for Automatic Music Generation,” Mathematics, vol. 11, no. 4, pp. 1-14, 2023.
[CrossRef] [Google Scholar] [Publisher Link]
[2] Miguel Civit et al., “A Systematic Review of Artificial Intelligence-Based Music Generation: Scope, Applications, and Future Trends,” Expert Systems with Applications, vol. 209, pp. 1-16, 2022.
[CrossRef] [Google Scholar] [Publisher Link]
[3] Zongyu Yin et al., “Deep Learning’s Shallow Gains: A Comparative Evaluation of Algorithms for Automatic Music Generation,” Machine Learning, vol. 112, no. 5, pp. 1785-1822, 2023.
[CrossRef] [Google Scholar] [Publisher Link]
[4] Luntian Mou et al., “Memomusic Version 2.0: Extending Personalized Music Recommendation with Automatic Music Generation,” 2022 IEEE International Conference on Multimedia and Expo Workshops (ICMEW), Taipei City, Taiwan, pp. 1-6, 2022.
[CrossRef] [Google Scholar] [Publisher Link]
[5] Shulei Ji, Xinyu Yang, and Jing Luo, “A Survey on Deep Learning for Symbolic Music Generation: Representations, Algorithms, Evaluations, and Challenges,” ACM Computing Surveys, vol. 56, no. 1, pp. 1-39, 2023.
[CrossRef] [Google Scholar] [Publisher Link]
[6] Yi-Jen Shih et al., “Theme Transformer: Symbolic Music Generation with Theme-Conditioned Transformer,” IEEE Transactions on Multimedia, vol. 25, pp. 3495-3508, 2022.
[CrossRef] [Google Scholar] [Publisher Link]
[7] Dyasha Dash, and Kathleen Agres, “AI-Based Affective Music Generation Systems: A Review of Methods, and Challenges,” arXiv, pp. 1-26, 2023.
[CrossRef] [Google Scholar] [Publisher Link]
[8] Prasant Singh Yadav et al., “A Lightweight Deep Learning-Based Approach for Jazz Music Generation in MIDI Format,” Computational Intelligence and Neuroscience, vol. 2022, no. 1, pp. 1-7, 2022.
[CrossRef] [Google Scholar] [Publisher Link]
[9] Jacopo de Berardinis et al., “Measuring the Structural Complexity of Music: From Structural Segmentations to the Automatic Evaluation of Models for Music Generation,” IEEE/ACM Transactions on Audio, Speech, and Language Processing, vol. 30, pp. 1963-1976, 2022.
[CrossRef] [Google Scholar] [Publisher Link]
[10] Shobhan Banerjee et al., “Music Generation Using Time Distributed Dense Stateful Char-RNNs,” IEEE 7th International Conference for Convergence in Technology, pp. 1-5, 2022.
[CrossRef] [Google Scholar] [Publisher Link]
[11] Jingwei Zhao, Gus Xia, and Ye Wang, “Domain Adversarial Training On Conditional Variational Auto-Encoder For Controllable Music Generation,” arXiv, pp. 1-8, 2022.
[CrossRef] [Google Scholar] [Publisher Link]
[12] Chenfei Kang et al., “EmoGen: Eliminating Subjective Bias in Emotional Music Generation,” arXiv, pp. 1-12, 2023.
[CrossRef] [Google Scholar] [Publisher Link]
[13] Pedro Sarmento et al., “GTR-CTRL: Instrument and Genre Conditioning For Guitar-Focused Music Generation with Transformers,” International Conference on Computational Intelligence in Music, Sound, Art, and Design, pp. 260-275, 2023.
[CrossRef] [Google Scholar] [Publisher Link]
[14] Zongyu Yin et al., “Measuring When a Music Generation Algorithm Copies Too Much: The Originality Report, Cardinality Score, and Symbolic Fingerprinting by Geometric Hashing,” SN Computer Science, vol. 3, no. 5, pp. 1-18, 2022.
[CrossRef] [Google Scholar] [Publisher Link]