Automated Detection of Cyber security Intrusions in Healthcare Systems Using Several Approaches
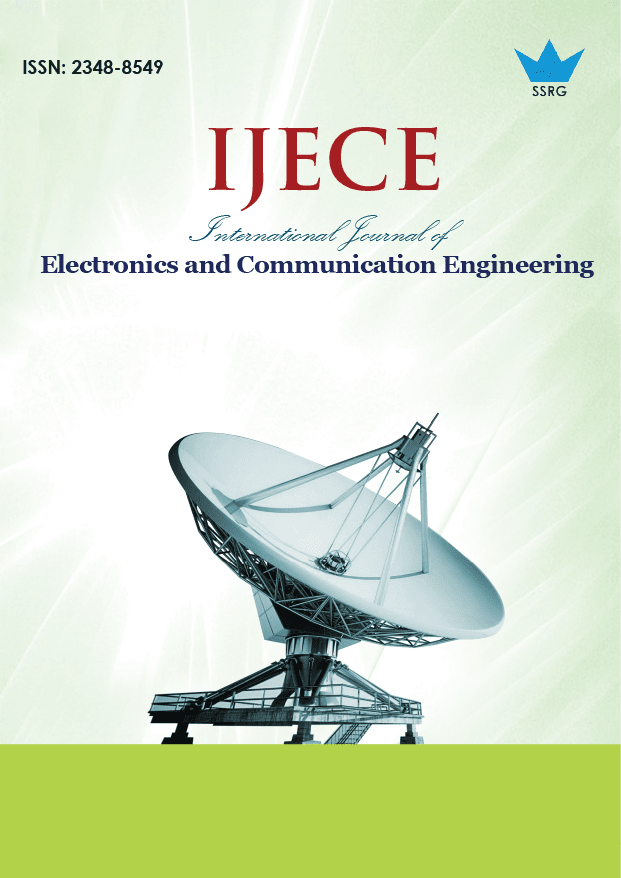
International Journal of Electronics and Communication Engineering |
© 2024 by SSRG - IJECE Journal |
Volume 11 Issue 7 |
Year of Publication : 2024 |
Authors : Shamija Sherryl. R. M. R, Sudhan. M. B, Deeptha. R, Karpagam. T |
How to Cite?
Shamija Sherryl. R. M. R, Sudhan. M. B, Deeptha. R, Karpagam. T, "Automated Detection of Cyber security Intrusions in Healthcare Systems Using Several Approaches," SSRG International Journal of Electronics and Communication Engineering, vol. 11, no. 7, pp. 220-227, 2024. Crossref, https://doi.org/10.14445/23488549/IJECE-V11I7P122
Abstract:
To ensure that patients are receiving the proper care, the healthcare data must be improved, real-time monitored, and accurate in illness detection. Thus, machine learning techniques are widely employed in Smart Healthcare Systems (SHS) to extract valuable features for tracking patient behaviors and forecasting various diseases from diverse and high-dimensional healthcare data. The kidneys gradually lose their functionality as a result of Chronic Kidney Disease (CKD). It talks about a medical condition that damages the kidneys and has an impact on a person's overall health. In this study, Recursive Feature Elimination (RFE) and multilayer perceptrons are used to develop a model for identifying anomalies and cyber-attacks (MLP). Experimental data are used to evaluate the suggested MLP model's performance. Recall, precision, accuracy, and F1- score are only a few of the performance metrics used to forecast patient activities. When compared to the RFE technique, the recommended strategy provides the highest levels of accuracy, precision, recall, and F1 score. Specifically, 98.56% recall, 98.13% F1-score, 98.76 accuracy, and 98.93% precision are obtained using the proposed MLP technique. When the outcomes were compared to recent state-of-the-art and machine learning algorithms from recent times, they performed better.
Keywords:
Internet of things, Cyber security intrusions, Smart healthcare systems, Intrusion detection, Machine learning.
References:
[1] Sara Alromaihi, Wael Elmedany, and Chitra Balakrishna, “Cyber Security Challenges of Deploying IoT in Smart Cities for Healthcare Applications,” 2018 6th International Conference on Future Internet of Things and Cloud Workshops (FiCloudW), Barcelona, Spain, pp. 140-145, 2018.
[CrossRef] [Google Scholar] [Publisher Link]
[2] Aysha K. Alharam, and Wael Elmedany, “The Effects of Cyber-Security on Healthcare Industry,” 2017 9th IEEE-GCC Conference and Exhibition (GCCCE), Manama, Bahrain, pp. 1-9, 2017.
[CrossRef] [Google Scholar] [Publisher Link]
[3] Jalal Al-Muhtadi et al., “Cybersecurity and Privacy Issues for Socially Integrated Mobile Healthcare Applications Operating in a Multi-Cloud Environment,” Health Informatics Journal, vol. 25, no. 2, pp. 315-329, 2019.
[CrossRef] [Google Scholar] [Publisher Link]
[4] Sudeep Tanwar, Karan Parekh, and Richard Evans, “Blockchain-Based Electronic Healthcare Record System for Healthcare 4.0 Applications,” Journal of Information Security and Applications, vol. 50, 2020.
[CrossRef] [Google Scholar] [Publisher Link]
[5] Anastasiia Strielkina et al., “Cybersecurity of Healthcare IoT-Based Systems: Regulation and Case-Oriented Assessment,” 2018 IEEE 9 th International Conference on Dependable Systems, Services and Technologies (DESSERT), Kyiv, Ukraine, pp. 67-73, 2018.
[CrossRef] [Google Scholar] [Publisher Link]
[6] M. Sornalakshmi et al., “RETRACTED ARTICLE: Hybrid Method for Mining Rules Based on Enhanced Apriori Algorithm with Sequential Minimal Optimization in Healthcare Industry,” Neural Computing and Applications, vol. 34, pp. 10597-10610, 2020.
[CrossRef] [Google Scholar] [Publisher Link]
[7] Parisa Naraei, Abdolreza Abhari, and Alireza Sadeghian, “Application of Multilayer Perceptron Neural Networks and Support Vector Machines in Classification of Healthcare Data,” 2016 Future Technologies Conference, San Francisco, CA, USA, pp. 848-852, 2016.
[CrossRef] [Google Scholar] [Publisher Link]
[8] Utkarsh Agrawal et al., “Hybrid Wolf-Bat Algorithm for Optimization of Connection Weights in Multi-layer Perceptron,” ACM Transactions on Multimedia Computing, Communications, and Applications, vol. 16, no. 1s, pp. 1-20, 2020.
[CrossRef] [Google Scholar] [Publisher Link]
[9] Xu-Dong Li et al., “Multi-Layer Perceptron Classification Method of Medical Data Based on Biogeography-Based Optimization Algorithm with Probability Distributions,” Applied Soft Computing, vol. 121, 2022.
[CrossRef] [Google Scholar] [Publisher Link]
[10] Anil Chacko, and Thaier Hayajneh, “Security and Privacy Issues with IoT in Healthcare,” EAI Endorsed Transactions on Pervasive Health and Technology, vol. 4, no. 14, pp. 1-7, 2018.
[CrossRef] [Google Scholar] [Publisher Link]
[11] Rashad J. McFarland, and Samuel BO Olatunbosun, “An Exploratory Study on the use of Internet_of_Medical_Things (IoMT) in the Healthcare Industry and their Associated Cybersecurity Risks,” Proceedings on the International Conference on Internet Computing (ICOMP), pp. 115-121, 2019.
[Google Scholar]
[12] Duncan Sparrell, “Cyber-Safety in Healthcare IOT,” 2019 ITU Kaleidoscope: ICT for Health: Networks, Standards and Innovation (ITU K), Atlanta, GA, USA, pp. 1-8, 2019.
[CrossRef] [Google Scholar] [Publisher Link]
[13] Abdulaziz A. Albesher, “IoT in Health-Care: Recent Advances in the Development of Smart Cyber-Physical Ubiquitous Environments,” IJCSNS International Journal of Computer Science and Network Security, vol. 19, no. 2, pp. 181-186, 2019.
[Google Scholar] [Publisher Link]
[14] Aarón Echeverría et al., “Cybersecurity Model Based on Hardening for Secure Internet of Things Implementation,” Applied Sciences, vol. 11, no. 7, pp. 1-28, 2021.
[CrossRef] [Google Scholar] [Publisher Link]
[15] Nicole M. Thomasian, and Eli Y. Adashi, “Cybersecurity in the Internet of Medical Things,” Health Policy and Technology, vol. 10, no. 3, 2021.
[CrossRef] [Google Scholar] [Publisher Link]
[16] Amir Djenna, Djamel Eddine Saidouni, and Wafia Abada, “A Pragmatic Cybersecurity Strategies for Combating IoT-Cyberattacks,” 2020 International Symposium on Networks, Computers and Communications (ISNCC), pp. 1-6, 2020.
[CrossRef] [Google Scholar] [Publisher Link]
[17] Pramila Arulanthu, and Eswaran Perumal, “An Intelligent IoT with Cloud Centric Medical Decision Support System for Chronic Kidney Disease Prediction,” International Journal of Imaging Systems and Technology, vol. 30, no. 3, pp. 815-827, 2020.
[CrossRef] [Google Scholar] [Publisher Link]
[18] Suliman A. Alsuhibany et al., “Ensemble of Deep Learning based Clinical Decision Support System for Chronic Kidney Disease Diagnosis in Medical Internet of Things Environment,” Computational Intelligence and Neuroscience, vol. 2021, no. 1, pp. 1-13, 2021.
[CrossRef] [Google Scholar] [Publisher Link]
[19] Mehdi Hosseinzadeh et al., “A Diagnostic Prediction Model for Chronic Kidney Disease in Internet of Things Platform,” Multimedia Tools and Applications, vol. 80, pp. 16933-16950, 2021.
[CrossRef] [Google Scholar] [Publisher Link]
[20] Alaa Noor et al., “An IoT based mHealth Platform for Chronic Kidney Disease Patients,” 2019 1st International Conference on Advances in Science, Engineering and Robotics Technology (ICASERT), Dhaka, Bangladesh, pp. 1-6, 2019.
[CrossRef] [Google Scholar] [Publisher Link]
[21] Phanindra Reddy Kannari, Noorullah Shariff Chowdary, and Rajkumar Laxmikanth Biradar, “An Anomaly-Based Intrusion Detection System Using Recursive Feature Elimination Technique for Improved Attack Detection,” Theoretical Computer Science, vol. 931, pp. 56-64, 2022.
[CrossRef] [Google Scholar] [Publisher Link]
[22] M. Akshay Kumaar et al., “A Hybrid Framework for Intrusion Detection in Healthcare Systems Using Deep Learning,” Frontiers in Public Health, vol. 9, pp. 1-18, 2022.
[CrossRef] [Google Scholar] [Publisher Link]
[23] Mustufa Haider Abidi, Hisham Alkhalefah, and Mohamed K. Aboudaif, “Enhancing Healthcare Data Security and Disease Detection Using Crossover-Based Multilayer Perceptron in Smart Healthcare Systems,” CMES-Computer Modeling in Engineering & Sciences, vol. 139, no, 1, pp. 1-21, 2024.
[CrossRef] [Google Scholar] [Publisher Link]
[24] Wenjuan Lian et al., “An Intrusion Detection Method based on Decision Tree-Recursive Feature Elimination in Ensemble Learning,” Mathematical Problems in Engineering, vol. 2020, no. 1, pp. 1-15, 2020.
[CrossRef] [Google Scholar] [Publisher Link]
[25] Darshana Upadhyay et al., “Intrusion Detection in SCADA based Power Grids: Recursive Feature Elimination Model with Majority Vote Ensemble Algorithm,” IEEE Transactions on Network Science and Engineering, vol. 8, no. 3, pp. 2559-2574, 2021.
[CrossRef] [Google Scholar] [Publisher Link]
[26] Ya Li, Seyed-mohsen Ghoreishi, and Alibek Issakhov, “Improving the Accuracy of Network Intrusion Detection System in Medical IoT Systems Through Butterfly Optimization Algorithm,” Wireless Personal Communications, vol. 126, no. 3, pp. 1999-2017, 2022.
[CrossRef] [Google Scholar] [Publisher Link]
[27] Oliver Kornyo et al., “Botnet Attacks Classification in AMI Networks with Recursive Feature Elimination (RFE) and Machine Learning Algorithms,” Computers & Security, vol. 135, 2023.
[CrossRef] [Google Scholar] [Publisher Link]
[28] Yan Zhang et al., “A Comparative Study of Cyber Security Intrusion Detection in Healthcare Systems,” International Journal of Critical Infrastructure Protection, vol. 44, 2024.
[CrossRef] [Google Scholar] [Publisher Link]
[29] Mavra Mehmood et al., “A Hybrid Approach for Network Intrusion Detection,” CMC-Computers Material Continua, vol. 70, no. 1, pp. 91-107, 2022.
[CrossRef] [Google Scholar] [Publisher Link]
[30] Priya Das, and Sohail Saif, Intrusion Detection in IoT-Based Healthcare using ML and DL Approaches: A Case Study, Artificial Intelligence and Cyber Security in Industry 4.0, pp. 271-294, 2023.
[CrossRef] [Google Scholar] [Publisher Link]