Energy Efficiency Optimization of Multiuser Cognitive Radio Network
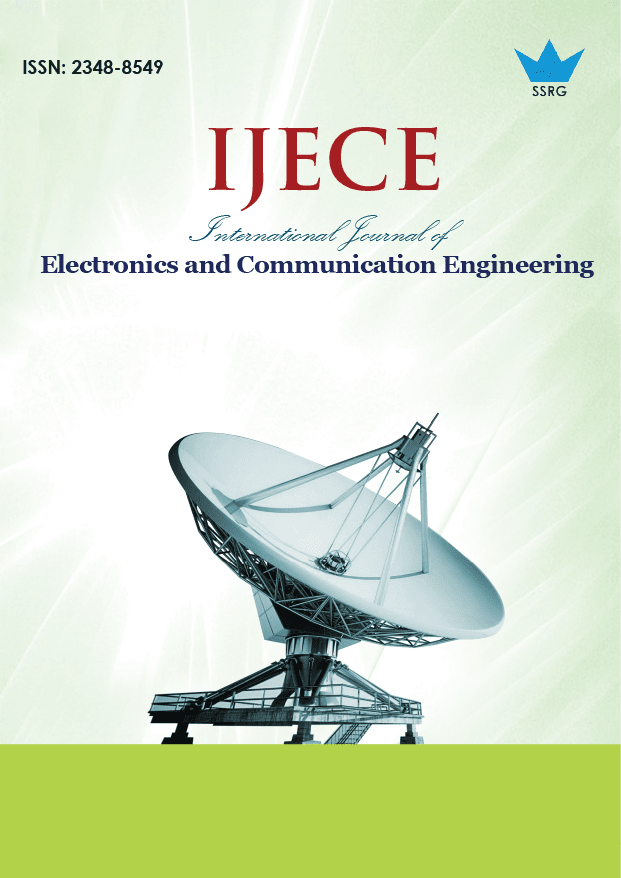
International Journal of Electronics and Communication Engineering |
© 2024 by SSRG - IJECE Journal |
Volume 11 Issue 8 |
Year of Publication : 2024 |
Authors : Arobindra Saikia, Ashim Jyoti Gogoi |
How to Cite?
Arobindra Saikia, Ashim Jyoti Gogoi, "Energy Efficiency Optimization of Multiuser Cognitive Radio Network," SSRG International Journal of Electronics and Communication Engineering, vol. 11, no. 8, pp. 11-21, 2024. Crossref, https://doi.org/10.14445/23488549/IJECE-V11I8P102
Abstract:
With the increasing demand for wireless devices in present times, the need for cognitive radio networks has risen. This increases the importance of the energy efficiency of the cognitive radio system. This work investigates the energy efficiency optimization of a multi-user single relay cognitive radio system. Collisions between data packets from numerous secondary users happen in a cognitive radio network, significantly reducing the throughput of the network, which causes a reduction in energy efficiency. This research presents a novel analytical energy efficiency model for the multi-user cognitive radio network. The proposed model makes it easy to formulate an optimization problem in such a way that the energy efficiency can be optimized by maintaining the collision probability within the permissible limit. As a result of this, an improvement in energy efficiency as well as the preservance of reliability of the system in the Cognitive Radio Network (CRN) is obtained. The energy efficiency optimization of the multiuser cognitive radio network is performed using optimization techniques like Particle Swarm Optimization (PSO), Human behavior based Particle Swarm Optimization (HPSO), and Aging Leader and Challengers Particle Swarm Optimization (ALCPSO). The simulation results demonstrate that, in comparison to alternative optimization methods, the optimized systems based on ALCPSO deliver higher energy efficiency. Additionally, a comparison is presented between the proposed method and the existing method for the multi-user cognitive radio system's possible energy efficiency. It is proved by the simulation results and analysis that the scheme proposed here improves the cognitive radio network's energy efficiency.
Keywords:
Energy efficiency, Aging leader and challengers particle swarm optimization, Multi user cognitive radio network, Secondary user, Transmission power.
References:
[1] Spectrum Efficiency Working Group, “Report of the Spectrum Efficiency Working Group,” Federal Communications Commission, Technical Report, 2002.
[Publisher Link]
[2] J. Mitola, and G.Q. Maguire, “Cognitive Radio: Making Software Radios More Personal,” IEEE Personal Communications, vol. 6, no. 4, pp. 13-18, 1999.
[CrossRef] [Google Scholar] [Publisher Link]
[3] Tevfik Yucek, and Huseyin Arslan, “A Survey of Spectrum Sensing Algorithms for Cognitive Radio Applications,” IEEE Communications Surveys & Tutorials, vol. 11, no. 1, pp. 116-130, 2009.
[CrossRef] [Google Scholar] [Publisher Link]
[4] Ying-Chang Liang et al., “Sensing-Throughput Tradeoff for Cognitive Radio Networks,” IEEE Transactions on Wireless Communications, vol. 7, no. 4, pp. 1326-1337, 2008.
[CrossRef] [Google Scholar] [Publisher Link]
[5] S. Stotas, and A. Nallanathan, “Overcoming the Sensing-Throughput Tradeoff in Cognitive Radio Networks,” IEEE International Conference on Communications, Cape Town, South Africa, pp. 1-5, 2010.
[CrossRef] [Google Scholar] [Publisher Link]
[6] Rozeha A. Rashid et al., “Efficient In-Band Spectrum Sensing Using Swarm Intelligence for Cognitive Radio Network,” Canadian Journal of Electrical and Computer Engineering, vol. 38, no. 2, pp.106-115, 2015.
[CrossRef] [Google Scholar] [Publisher Link]
[7] Qian Zhang, Juncheng Jia, and Jin Zhang, “Cooperative Relay to Improve Diversity in Cognitive Radio Networks,” IEEE Communications Magazine, vol. 47, no. 2, pp. 111-117, 2009.
[CrossRef] [Google Scholar] [Publisher Link]
[8] Shaahin Tabatabaee, and Vahid Tabataba Vakili, “A New Method for Sensing Cognitive Radio Network under Malicious Attacker,” International Journal of Communications, Network and System Sciences, vol. 6, no. 1, pp. 60-65, 2013.
[CrossRef] [Google Scholar] [Publisher Link]
[9] Shiwei Huang et al., “Energy-Efficient Cooperative Spectrum Sensing with Amplify-and-Forward Relaying,” IEEE Communications Letters, vol. 16, no. 4, pp. 450-453, 2012.
[CrossRef] [Google Scholar] [Publisher Link]
[10] Shiwei Huang, Hongbin Chen, and Yan Zhang, “Optimal Power Allocation for Spectrum Sensing and Data Transmission in Cognitive Relay Networks,” IEEE Wireless Communications Letters, vol. 1, no. 1, pp. 26-29, 2012.
[CrossRef] [Google Scholar] [Publisher Link]
[11] Song Yaolian et al., “Energy Efficiency Optimization of Cognitive Relay Network Based on Cooperative Spectrum Sensing,” The Journal of China Universities of Posts and Telecommunications, vol. 22, no. 3, pp. 26-34, 2015.
[CrossRef] [Google Scholar] [Publisher Link]
[12] Subhankar Chatterjee, Santi P. Maity, and Tamaghna Acharya, “Energy Efficient Cognitive Radio System for Joint Spectrum Sensing and Data Transmission,” IEEE Journal on Emerging and Selected Topics in Circuits and Systems, vol. 4, no. 3, pp. 292-300, 2014.
[CrossRef] [Google Scholar] [Publisher Link]
[13] Shanshan Wang, Junshan Zhang, and Lang Tong, “Delay Analysis for Cognitive Radio Networks with Random Access: A Fluid Queue View,” Proceedings IEEE INFOCOM, San Diego, CA, USA, pp. 1-9, 2010.
[CrossRef] [Google Scholar] [Publisher Link]
[14] Shiwei Huang et al., “Sensing-Energy Trade-off in Cognitive Radio Networks with Relays,” IEEE Systems Journal, vol. 7, no. 1, pp. 68- 76, 2013.
[CrossRef] [Google Scholar] [Publisher Link]
[15] Surajit Basak, and Tamaghna Acharya, “Joint Power Allocation and Routing in Outage Constrained Cognitive Radio Ad Hoc Networks,” Mobile Networks and Applications, vol. 20, no. 5, pp. 636-648, 2015.
[CrossRef] [Google Scholar] [Publisher Link]
[16] Chai Zheng-Yi et al., “Throughput Optimization in Cognitive Wireless Network Based on Clone Selection Algorithm,” Computers & Electrical Engineering, vol. 52, pp. 328-336, 2016.
[CrossRef] [Google Scholar] [Publisher Link]
[17] Supreet Singh, and Surbhi Sharma, “Performance Optimisation of RF Energy Harvesting Relay-Based Interweave/Underlay Cognitive Radio Network,” International Journal of Electronics, vol. 104, no. 9, pp. 1546-1561, 2017.
[CrossRef] [Google Scholar] [Publisher Link]
[18] Dan Wu, Liang Zhou, and Yueming Cai, “Social-Aware Rate Based Content Sharing Mode Selection for D2D Content Sharing Scenarios,” IEEE Transactions on Multimedia, vol. 19, no. 11, pp. 2571-2582, 2017.
[CrossRef] [Google Scholar] [Publisher Link]
[19] Yan-Chao Zhao et al., “Throughput Optimization in Cognitive Radio Networks Ensembling Physical Layer Measurement,” Journal of Computer Science and Technology, vol. 30, pp. 1290-1305, 2015.
[CrossRef] [Google Scholar] [Publisher Link]
[20] Liang Zhou, “Mobile Device-to-Device Video Distribution,” ACM Transactions on Multimedia Computing, Communications, and Applications, vol. 12, no. 3, pp. 1-23, 2016.
[CrossRef] [Google Scholar] [Publisher Link]
[21] Yaqing Wang et al., “Maximizing Average Throughput of Cooperative Cognitive Radio Networks Based on Energy Harvesting,” Sensors, vol. 22, no. 22, pp. 1-17, 2022.
[CrossRef] [Google Scholar] [Publisher Link]
[22] Ning Wang et al., “Distributed Energy Efficiency Optimization for Multi-User Cognitive Radio Networks Over MIMO Interference Channels: A Non-Cooperative Game Approach,” IEEE Access, vol. 8, pp. 26701-26714, 2020.
[CrossRef] [Google Scholar] [Publisher Link]
[23] Kaleem Arshid et al., “Energy Efficiency in Cognitive Radio Network Using Cooperative Spectrum Sensing Based on Hybrid Spectrum Handoff,” Egyptian Informatics Journal, vol. 23, no. 4, pp. 77-88, 2022.
[CrossRef] [Google Scholar] [Publisher Link]
[24] Meenakshi Awasthi, M.J. Nigam, and Vijay Kumar, “Optimal Sensing and Transmission of Energy Efficient Cognitive Radio Networks,” Wireless Personal Communications, vol. 111, no. 2, pp. 1283-1294, 2019.
[CrossRef] [Google Scholar] [Publisher Link]
[25] Y. Shi, and R.C. Eberhart, “Empirical Study of Particle Swarm Optimization,” Proceedings of the 1999 Congress on Evolutionary Computation-CEC99, Washington, DC, USA, vol. 3, pp. 1945-1950, 1999.
[CrossRef] [Google Scholar] [Publisher Link]
[26] Y. Shi, and R. Eberhart, “A Modified Particle Swarm Optimizer,” 1998 IEEE International Conference on Evolutionary Computation Proceedings. IEEE World Congress on Computational Intelligence, Anchorage, AK, USA, pp. 69-73, 1998.
[CrossRef] [Google Scholar] [Publisher Link]
[27] Hao Liu et al., “Human Behavior-Based Particle Swarm Optimization,” The Scientific World Journal, vol. 2014, no. 1, pp. 1-14, 2014.
[CrossRef] [Google Scholar] [Publisher Link]
[28] Wei-Neng Chen et al., “Particle Swarm Optimization with an Aging Leader and Challengers,” IEEE Transactions on Evolutionary Computation, vol. 17, no. 2, pp. 241-258, 2013.
[CrossRef] [Google Scholar] [Publisher Link]
[29] Seyedali Mirjalili, and Andrew Lewis, “The Whale Optimization Algorithm,” Advances in Engineering Software, vol. 95, pp. 51-67, 2016.
[CrossRef] [Google Scholar] [Publisher Link]
[30] Chabungbam Lison Singh, K.L. Baishnab, and Ch. Anandini, “Analysis and Optimization of Noises of an Analog Circuit via PSO Algorithms,” Microsystem Technologies, vol. 25, no. 5, pp. 1793-1807, 2017.
[CrossRef] [Google Scholar] [Publisher Link]
[31] Ye Jun, and Zhu Qi, “Optimization of Cooperative Sensing Based on Energy Consume in Cognitive Radio Networks,” IEEE Conference Anthology, China, pp. 1-5, 2013.
[CrossRef] [Google Scholar] [Publisher Link]
[32] Nitin Sharma et al., “On the Use of Particle Swarm Optimization for Adaptive Resource Allocation in Orthogonal Frequency Division Multiple Access Systems with Proportional Rate Constraints,” Information Sciences, vol. 182, no. 1, pp. 115-124, 2012.
[CrossRef] [Google Scholar] [Publisher Link]