Enhanced Breast Cancer Detection in Ultrasound Imaging Using an Attention Based U-Net++ Architecture for Improved Tumor Segmentation
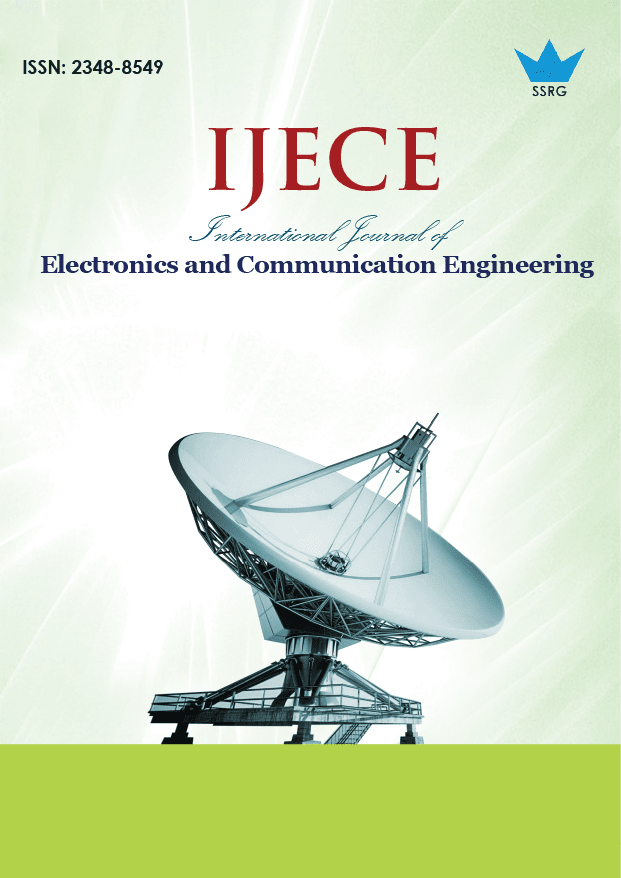
International Journal of Electronics and Communication Engineering |
© 2024 by SSRG - IJECE Journal |
Volume 11 Issue 8 |
Year of Publication : 2024 |
Authors : C. Valarmathi, S. John Justin Thangaraj |
How to Cite?
C. Valarmathi, S. John Justin Thangaraj, "Enhanced Breast Cancer Detection in Ultrasound Imaging Using an Attention Based U-Net++ Architecture for Improved Tumor Segmentation," SSRG International Journal of Electronics and Communication Engineering, vol. 11, no. 8, pp. 82-89, 2024. Crossref, https://doi.org/10.14445/23488549/IJECE-V11I8P109
Abstract:
Breast cancer, the most commonly occurring cancer among women and a leading reason of cancer mortality worldwide, necessitates early detection to reduce mortality rates. Breast Ultrasound (BUS) imaging, coupled with Computer Aided Diagnosis (CAD) systems, has emerged as a crucial, non-invasive, non-radioactive and low cost method for early breast cancer detection. Its suitability for large-scale screening and diagnosis, particularly in low-resource settings, further underscores its importance. However, the automatic segmentation of tumors in BUS images remains disputing due to the lower quality of the image, characterized by speckle noise, low contrast, feeble boundaries and artifacts. A histogram equalization was used as a pre-processing model to overcome such an issue. Additionally, the significant variation in tumor shape, size and echo strength across patients complicates the application of conventional segmentation methods that rely on strong priors to object features. This work proposes a novel attention-based U-Net++ architecture designed to address these challenges and achieve highly accurate breast cancer segmentation in BUS images. This model integrates an attention mechanism to boost the network’s ability to attention to pertinent image regions, improving tumours’ delineation despite noise and artifacts. The architecture incorporates the U-Net++’s strength in handling biomedical image segmentation tasks, incorporating skip connections to preserve spatial information and attention gates to filter and refine features at multiple scales. Through extensive experimentation and evaluation using Python, the attention-based U-Net++ demonstrates superior performance in segmenting tumors in BUS images, outperforming conventional models in terms of accuracy and robustness.
Keywords:
Attention gates, Attention-based U-Net++, BUS image, CAD systems, Histogram equalization.
References:
[1] Eman Radhi, and Mohammed Kamil, “An Automatic Segmentation of Breast Ultrasound Images Using U-Net Model,” Serbian Journal of Electrical Engineering, vol. 20, no. 2, pp. 191-203, 2023.
[CrossRef] [Google Scholar] [Publisher Link]
[2] Sushovan Chaudhury et al., “Effective Image Processing and Segmentation-Based Machine Learning Techniques for Diagnosis of Breast Cancer,” Computational and Mathematical Methods in Medicine, vol. 2022, no. 1, pp. 1-7, 2022.
[CrossRef] [Google Scholar] [Publisher Link]
[3] Gongping Chen et al., “Rethinking the Unpretentious U-Net for Medical Ultrasound Image Segmentation,” Pattern Recognition, vol. 142, 2023.
[CrossRef] [Google Scholar] [Publisher Link]
[4] Tianyu Zhao, and Hang Dai, “Breast Tumor Ultrasound Image Segmentation Method Based on Improved Residual U-Net Network,” Computational Intelligence and Neuroscience, vol. 2022, no. 1, pp. 1-9, 2022.
[CrossRef] [Google Scholar] [Publisher Link]
[5] Donya Khaledyan et al., “Enhancing Breast Ultrasound Segmentation through Fine-Tuning and Optimization Techniques: Sharp Attention Unet,” Plos one, vol. 18, no. 12, pp. 1-22, 2023.
[CrossRef] [Google Scholar] [Publisher Link]
[6] Yue Zhou et al., “Multi-Task Learning for Segmentation and Classification of Tumors in 3D Automated Breast Ultrasound Images,” Medical Image Analysis, vol. 70, 2021.
[CrossRef] [Google Scholar] [Publisher Link]
[7] Qinghua Huang et al., “Segmentation of Breast Ultrasound Image with Semantic Classification of Superpixels,” Medical Image Analysis, vol. 61, 2020.
[CrossRef] [Google Scholar] [Publisher Link]
[8] Muhammad Sakib Khan Inan, Fahim Irfan Alam, and Rizwan Hasan, “Deep Integrated Pipeline of Segmentation Guided Classification of Breast Cancer from Ultrasound Images,” Biomedical Signal Processing and Control, vol. 75, 2022.
[CrossRef] [Google Scholar] [Publisher Link]
[9] Kailuo Yu, Sheng Chen, and Yanghuai Chen, “Tumor Segmentation in Breast Ultrasound Image by Means of Res Path Combined with Dense Connection Neural Network,” Diagnostics, vol. 11, no. 9, pp. 1-14, 2021.
[CrossRef] [Google Scholar] [Publisher Link]
[10] Bryar Shareef et al., “Estan: Enhanced Small Tumor - Aware Network for Breast Ultrasound Image Segmentation,” Healthcare, vol. 10, no. 11, pp. 1-14, 2022.
[CrossRef] [Google Scholar] [Publisher Link]
[11] Zhemin Zhuang et al., “Multi-Features-Based Automated Breast Tumor Diagnosis using Ultrasound Image and Support Vector Machine,” Computational Intelligence and Neuroscience, vol. 2021, no. 1, pp. 1-12, 2021.
[CrossRef] [Google Scholar] [Publisher Link]
[12] Meng Xu, Kuan Huang, and Xiaojun Qi, “A Regional-Attentive Multi-Task Learning Framework for Breast Ultrasound Image Segmentation and Classification,” IEEE Access, vol. 11, pp. 5377-5392, 2023.
[CrossRef] [Google Scholar] [Publisher Link]
[13] Sara Laghmati et al., “Segmentation of Breast Cancer on Ultrasound Images using Attention U-Net Model,” International Journal of Advanced Computer Science and Applications, vol. 14, no. 8, pp. 770-778, 2023.
[CrossRef] [Google Scholar] [Publisher Link]
[14] Taukir Alam et al., “Improving Breast Cancer Detection and Diagnosis through Semantic Segmentation Using the Unet3+ Deep Learning Framework,” Biomedicines, vol. 11, no. 6, pp. 1-12, 2023.
[CrossRef] [Google Scholar] [Publisher Link]
[15] Shahed Hossain et al.,, “Automated Breast Tumor Ultrasound Image Segmentation with Hybrid UNet and Classification Using Fine-Tuned CNN Model,” Heliyon, vol. 9, no. 11, pp. 1-31, 2023.
[CrossRef] [Google Scholar] [Publisher Link]
[16] Hao Feng et al., “Identifying Malignant Breast Ultrasound Images Using ViT-Patch,” Applied Sciences, vol. 13, no. 6, pp. 1-12, 2023.
[CrossRef] [Google Scholar] [Publisher Link]
[17] Shuai Zhang et al., “Fully Automatic Tumor Segmentation of Breast Ultrasound Images with Deep Learning,” Journal of Applied Clinical Medical Physics, vol. 24, no. 1, pp. 1-13, 2023.
[CrossRef] [Google Scholar] [Publisher Link]
[18] Alessandro Sebastian Podda et al., “Fully-Automated Deep Learning Pipeline for Segmentation and Classification of Breast Ultrasound Images,” Journal of Computational Science, vol. 63, 2022.
[CrossRef] [Google Scholar] [Publisher Link]
[19] Yingtao Zhang et al., “BUSIS: A Benchmark for Breast Ultrasound Image Segmentation,” Healthcare, vol. 10, no. 4, pp. 1-16, 2022.
[CrossRef] [Google Scholar] [Publisher Link]
[20] Yaozhong Luo, Qinghua Huang, and Xuelong Li, “Segmentation Information with Attention Integration for Classification of Breast Tumor In Ultrasound Image,” Pattern Recognition, vol. 124, 2022.
[CrossRef] [Google Scholar] [Publisher Link]
[21] Xiaoyan Shen et al., “Lesion Segmentation in Breast Ultrasound Images Using the Optimized Marked Watershed Method,” Biomedical Engineering Online, vol. 20, no. 1, pp. 1-23, 2021.
[CrossRef] [Google Scholar] [Publisher Link]
[22] Rania Almajalid et al., “Development of a Deep-Learning-Based Method for Breast Ultrasound Image Segmentation,” 17th IEEE International Conference on Machine Learning and Applications, Orlando, FL, USA, pp. 1103-1108, 2018.
[CrossRef] [Google Scholar] [Publisher Link]