Smart Sight: Intelligent Vision for Stroke Lesion Detection and Classification
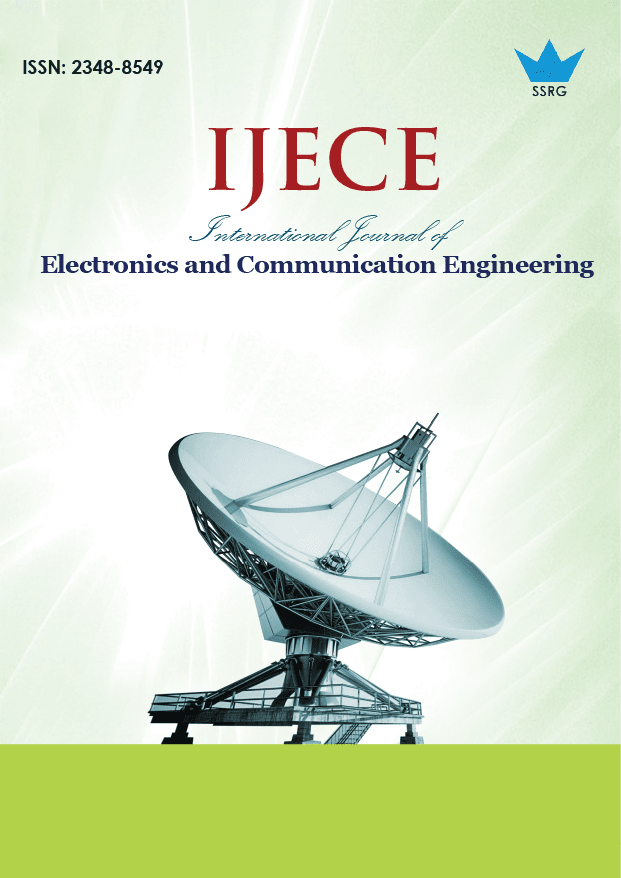
International Journal of Electronics and Communication Engineering |
© 2024 by SSRG - IJECE Journal |
Volume 11 Issue 8 |
Year of Publication : 2024 |
Authors : M. Fathima Beevi, N. Santhi, N. Ramasamy |
How to Cite?
M. Fathima Beevi, N. Santhi, N. Ramasamy, "Smart Sight: Intelligent Vision for Stroke Lesion Detection and Classification," SSRG International Journal of Electronics and Communication Engineering, vol. 11, no. 8, pp. 98-110, 2024. Crossref, https://doi.org/10.14445/23488549/IJECE-V11I8P111
Abstract:
Stroke is the traumatic condition of nerve cells that block down the physical activity of the victims within a short span of time. It is the leading reason for disability and mortality among the older population. A timely diagnosis is a crucial step in case of stroke treatment. Contemporary diagnostic techniques, including Magnetic Resonance Imaging (MRI) and ComputerAided Tomography (CT), have wide applications in detecting stroke lesions. This paper introduces a novel approach for addressing the critical task of accurately segmenting stroke lesions from medical images, which is vital for precise diagnosis and effective treatment planning. The proposed approach integrates DenseNet-201 and Capsule Network architectures to develop a hybrid deep learning model. DenseNet-201 serves as a feature extractor, facilitating enhanced feature propagation and gradient flow throughout the network. Meanwhile, Capsule Network introduces capsules to handle hierarchical relationships, improving the model’s ability to capture intricate spatial hierarchies in the data. The dataset used for training and evaluation consists of brain CT images sourced from the Kaggle Repository, including both normal and stroke brain CT images. Through preprocessing and augmentation techniques, the dataset’s quality and diversity are enhanced to ensure effective model training. Experiment results show how effective the suggested hybrid model is, achieving an accuracy of 93.45%, precision of 92.18%, recall of 92.56%, and F1-Score 92.36%. When compared to other approaches currently in use, the suggested method performs better in terms of robustness and segmentation accuracy. Overall, this hybrid deep learning model offers a promising solution to the challenges of stroke lesion detection and classification, with implications for improving patient care and treatment outcomes in clinical settings.
Keywords:
Stroke lesions, CT imaging, Deep Learning models, Detection, Classification, Capsule network.
References:
[1] Maarten G. Lansberg et al., “MRI Profile and Response to Endovascular Reperfusion After Stroke (DEFUSE 2): A Prospective Cohort Study,” The Lancet Neurology, vol. 11, no. 10, pp. 860-867, 2012.
[CrossRef] [Google Scholar] [Publisher Link]
[2] Senthil Kumar Thiyagarajan, and Kalpana Murugan “A Systematic Review on Techniques Adapted for Segmentation and Classification of Ischemic Stroke Lesions from Brain MR Images,” Wireless Personal Communications, vol. 118, pp. 1225-1244, 2021.
[CrossRef] [Google Scholar] [Publisher Link]
[3] A. Gregory Sorensen et al., “Hyperacute Stroke: Simultaneous Measurement of Relative Cerebral Blood Volume, Relative Cerebral Blood Flow, and Mean Tissue Transit Time,” Radiology, vol. 210, no. 2, pp. 519-527, 1999.
[CrossRef] [Google Scholar] [Publisher Link]
[4] Max Wintermark et al., “Acute Stroke Imaging Research Roadmap II,” Stroke, vol. 44, no. 9, pp. 2628-2639, 2013.
[CrossRef] [Google Scholar] [Publisher Link]
[5] M. Koenig et al., “Perfusion CT of the Brain: Diagnostic Approach for Early Detection of Ischemic Stroke,” Radiology, vol. 209, no. 1, pp. 85-93, 1998.
[CrossRef] [Google Scholar] [Publisher Link]
[6] Albert Clèrigues et al., “Acute and Sub-Acute Stroke Lesion Segmentation from Multimodal MRI,” Computer Methods and Programs in Biomedicine, vol. 194, 2020.
[CrossRef] [Google Scholar] [Publisher Link]
[7] Amish Kumar et al., “CSNet: A New DeepNet Framework for Ischemic Stroke Lesion Segmentation,” Computer Methods and Programs in Biomedicine, vol. 193, 2020.
[CrossRef] [Google Scholar] [Publisher Link]
[8] Sanaz Nazari-Farsani et al., “Automated Segmentation of Acute Stroke Lesions Using a Data-Driven Anomaly Detection on Diffusion Weighted MRI,” Journal of Neuroscience Methods, vol. 333, 2020.
[CrossRef] [Google Scholar] [Publisher Link]
[9] Naofumi Tomita et al., “Automatic Post-Stroke Lesion Segmentation on MR Images Using 3D Residual Convolutional Neural Network,” NeuroImage: Clinical, vol. 27, pp. 1-7, 2020.
[CrossRef] [Google Scholar] [Publisher Link]
[10] Liyuana Cui et al., “Deep Symmetric Threedimensional Convolutional Neural Networks for Identifying Acute Ischemic Stroke Via Diffusion-Weighted Images,” Journal of X-Ray Science and Technology, vol. 29, no. 4, pp. 551-566, 2021.
[CrossRef] [Google Scholar] [Publisher Link]
[11] Yi-Chia Wei et al., “Semantic Segmentation Guided Detector for Segmentation, Classification, and Lesion Mapping of Acute Ischemic Stroke in MRI Images,” NeuroImage: Clinical, vol. 35, pp. 1-12, 2022.
[CrossRef] [Google Scholar] [Publisher Link]
[12] Sanaz Nazari-Farsani et al., “Predicting Final Ischemic Stroke Lesions from Initial Diffusion-Weighted Images Using a Deep Neural Network,” NeuroImage: Clinical, vol. 37, pp. 1-10, 2023.
[CrossRef] [Google Scholar] [Publisher Link]
[13] G.B. Praveen et al., “Ischemic Stroke Lesion Segmentation Using Stacked Sparse Autoencoder,” Computers in Biology and Medicine, vol. 99, pp. 38-52, 2018.
[CrossRef] [Google Scholar] [Publisher Link]
[14] Rongzhao Zhang et al., “Automatic Segmentation of Acute Ischemic Stroke from DWI Using 3-D Fully Convolutional DenseNets,” IEEE Transactions on Medical Imaging, vol. 37, no. 9, pp. 2149-2160, 2018.
[CrossRef] [Google Scholar] [Publisher Link]
[15] Long Zhang et al., “Ischemic Stroke Lesion Segmentation Using Multi-Plane Information Fusion,” IEEE Access, vol. 8, pp. 45715-45725, 2020.
[CrossRef] [Google Scholar] [Publisher Link]
[16] Albert Clèrigues et al., “Acute Ischemic Stroke Lesion Core Segmentation in CT Perfusion Images Using Fully Convolutional Neural Networks,” Computers in Biology and Medicine, vol. 115, pp. 1-7, 2019.
[CrossRef] [Google Scholar] [Publisher Link]
[17] Mohsen Soltanpour et al., “Ischemic Stroke Lesion Prediction in CT Perfusion Scans Using Multiple Parallel U-Nets Following by a Pixel-Level Classifier,” IEEE 19th International Conference on Bioinformatics and Bioengineering, Athens, Greece, pp. 957-963, 2019.
[CrossRef] [Google Scholar] [Publisher Link]
[18] Wenyue Shi, and Heng Liu, “Modified U-Net Architecture for Ischemic Stroke Lesion Segmentation and Detection,” IEEE 4th Advanced Information Technology, Electronic and Automation Control Conference, Chengdu, China, pp. 1068-1071, 2019.
[CrossRef] [Google Scholar] [Publisher Link]
[19] Batyrkhan Omarov et al., “Modified UNet Model for Brain Stroke Lesion Segmentation on Computed Tomography Images,” Computers, Materials & Continua, vol. 71, no. 3, 2022.
[CrossRef] [Google Scholar] [Publisher Link]
[20] Aayush Jaiswal et al., “Classification of the COVID-19 Infected Patients Using DenseNet201 Based Deep Transfer Learning,” Journal of Biomolecular Structure and Dynamics, vol. 39, no. 15, pp. 5682-5689, 2021.
[CrossRef] [Google Scholar] [Publisher Link]
[21] Guoyan Li et al., “Compound Fault Diagnosis of Planetary Gearbox Based on Improved LTSS-BoW Model and Capsule Network,” Sensors, vol. 24, no. 3, pp. 1-20, 2024.
[CrossRef] [Google Scholar] [Publisher Link]