The Analysis and Comparison of LMS-Based Filtering Techniques for EEG Signals: Towards Informed Decision Making
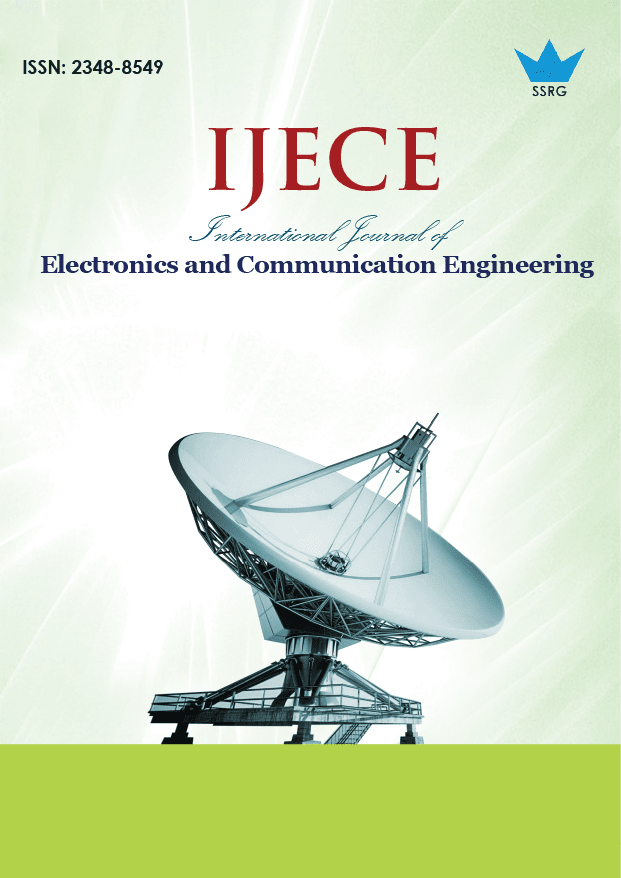
International Journal of Electronics and Communication Engineering |
© 2024 by SSRG - IJECE Journal |
Volume 11 Issue 8 |
Year of Publication : 2024 |
Authors : Garlet Llaza Huamani, Bryan Sanchez Urure, Estefany Huaman Colque, Jesús Talavera S. |
How to Cite?
Garlet Llaza Huamani, Bryan Sanchez Urure, Estefany Huaman Colque, Jesús Talavera S., "The Analysis and Comparison of LMS-Based Filtering Techniques for EEG Signals: Towards Informed Decision Making," SSRG International Journal of Electronics and Communication Engineering, vol. 11, no. 8, pp. 123-130, 2024. Crossref, https://doi.org/10.14445/23488549/IJECE-V11I8P113
Abstract:
Electroencephalography (EEG) is essential for observing brain activity. It offers non-invasive, high-resolution insights into neural dynamics. Despite its clinical and research applications, EEG signals are prone to noise from powerline interference, muscle artifacts and environmental sources. This study evaluates adaptive filtering techniques—LMS, NLMS, PNLMS and IPNLMS—for denoising EEG signals. A dataset of 23 EEG recordings contaminated with noise was used. Accelerometer signals served as reference inputs. The algorithms were assessed using Mean Squared Error (MSE) Signal-toNoise Ratio (SNR) and Pearson Correlation Coefficient. PNLMS was found to be the most effective. It achieved the lowest MSE (0.9193), highest SNR (1.0768) and highest correlation (46.9025%). While PNLMS excels in noise reduction it has computational demands that may limit its use in wearable devices. NLMS offers a practical balance. It balances performance and efficiency. Future work includes hybrid algorithms. Real-time implementations will be addressed. Adaptive parameter tuning will also be covered. These aim to enhance EEG signal processing and its applications in clinical and research environments.
Keywords:
EEG signal, LMS filtering, Motion artifact, NLMS, PNLMS, IPNLMS.
References:
[1] Paul Sauseng, and Wolfgang Klimesch, “What Does Phase Information of Oscillatory Brain Activity Tell Us About Cognitive Processes?,” Neuroscience & Biobehavioral Reviews, vol. 32, no. 5, pp. 1001-1013, 2008.
[CrossRef] [Google Scholar] [Publisher Link]
[2] U. Rajendra Acharya et al., “Deep Convolutional Neural Network for the Automated Detection and Diagnosis of Seizure Using EEG Signals,” Computers in Biology and Medicine, vol. 100, pp. 270-278, 2018.
[CrossRef] [Google Scholar] [Publisher Link]
[3] Pejman Memar, and Farhad Faradji, “A Novel Multi-Class EEG-Based Sleep Stage Classification System,” IEEE Transactions on Neural Systems and Rehabilitation Engineering, vol. 26, no. 1, pp. 84-95, 2018.
[CrossRef] [Google Scholar] [Publisher Link]
[4] Betul Ay et al., “Automated Depression Detection Using Deep Representation and Sequence Learning with EEG Signals,” Journal of Medical Systems, vol. 43, 2019.
[CrossRef] [Google Scholar] [Publisher Link]
[5] P.S. Hamilton, “A Comparison of Adaptive and Nonadaptive Filters for Reduction of Power Line Interference in the ECG,” IEEE Transactions on Biomedical Engineering, vol. 43, no. 1, pp. 105-109, 1996.
[CrossRef] [Google Scholar] [Publisher Link]
[6] I.I. Goncharova et al., “EMG Contamination of EEG: Spectral and Topographical Characteristics,” Clinical Neurophysiology, vol. 114, no. 9, pp. 1580-1593, 2003.
[CrossRef] [Google Scholar] [Publisher Link]
[7] Andrei La Rosa et al., “Exploring NLMS and IPNLMS Adaptive Filtering VLSI Hardware Architectures for Robust EEG Signal Artifacts Elimination,” 2020 27th IEEE International Conference on Electronics, Circuits and Systems, Glasgow, UK, pp. 1-4, 2020.
[CrossRef] [Google Scholar] [Publisher Link]
[8] Salim Çınar, “Design of an Automatic Hybrid System for Removal of Eye-Blink Artifacts from EEG Recordings,” Biomedical Signal Processing and Control, vol. 67, 2021.
[CrossRef] [Google Scholar] [Publisher Link]
[9] Alia Darroudi et al., “EEG Adaptive Noise Cancellation Using Information Theoretic Approach,” Bio-Medical Materials and Engineering, vol. 28, no. 4, pp. 325-338, 2017.
[CrossRef] [Google Scholar] [Publisher Link]
[10] K.A. Lee, and W.S. Gan, “Improving Convergence of the NLMS Algorithm Using Constrained Subband Updates,” IEEE Signal Processing Letters, vol. 11, no. 9, pp. 736-739, 2004.
[CrossRef] [Google Scholar] [Publisher Link]
[11] D.L. Duttweiler, “Proportionate Normalized Least-Mean-Squares Adaptation in Echo Cancelers,” IEEE Transactions on Speech and Audio Processing, vol. 8, no. 5, pp. 508-518, 2000.
[CrossRef] [Google Scholar] [Publisher Link]
[12] Constantin Paleologu, Jacob Benesty, and Silviu Ciochină, “An Improved Proportionate NLMS Algorithm Based on the l0 Norm,” 2010 IEEE International Conference on Acoustics, Speech and Signal Processing, Dallas, TX, USA, pp. 309-312, 2010.
[CrossRef] [Google Scholar] [Publisher Link]
[13] Binqiang Chen et al., “Removal of Power Line Interference From ECG Signals Using Adaptive Notch Filters of Sharp Resolution,” IEEE Access, vol. 7, pp. 150667-150676, 2019.
[CrossRef] [Google Scholar] [Publisher Link]
[14] Muhammad Zahak Jamal, Dong-Hyun Lee, and Dong Jin Hyun, “Real Time Adaptive Filter Based EMG Signal Processing and Instrumentation Scheme for Robust Signal Acquisition Using Dry EMG Electrodes,” 2019 16th International Conference on Ubiquitous Robots (UR), Jeju, Korea (South), pp. 683-688, 2019.
[CrossRef] [Google Scholar] [Publisher Link]
[15] Kevin T. Sweeney et al., “A Methodology for Validating Artifact Removal Techniques for Physiological Signals,” IEEE Transactions on Information Technology in Biomedicine, vol. 16, no. 5, pp. 918-926, 2012.
[CrossRef] [Google Scholar] [Publisher Link]
[16] Ary L. Goldberger et al., “PhysioBank, PhysioToolkit, and PhysioNet: Components of a New Research Resource for Complex Physiologic Signals,” Circulation, vol. 101, no. 23, pp. 215-220, 2000.
[CrossRef] [Google Scholar] [Publisher Link]
[17] Jarelh Galdos et al., “Comparison and Evaluation of LMS-Derived Algorithms Applied on ECG Signals Contaminated with Motion Artifact during Physical Activities,” Applied Computer Science, vol. 20, no. 1, pp. 157-172, 2024.
[CrossRef] [Google Scholar] [Publisher Link]