Data-Driven Insights into Gestational Diabetes Mellitus: Enhancing Models for Prediction by SVM Imputation for Personalized Pregnancy Care
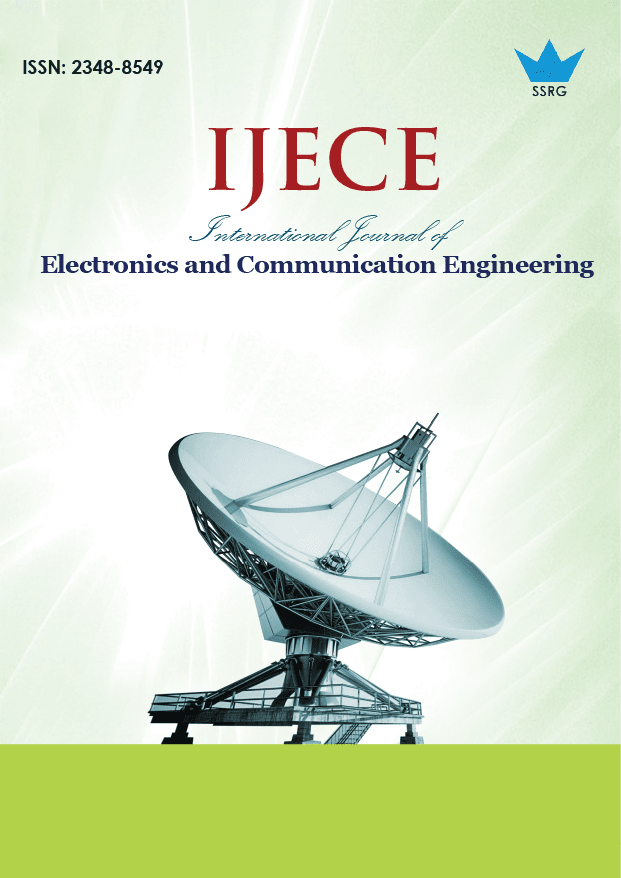
International Journal of Electronics and Communication Engineering |
© 2024 by SSRG - IJECE Journal |
Volume 11 Issue 8 |
Year of Publication : 2024 |
Authors : T. Sujatha, K. R. Ananthapadmanaban |
How to Cite?
T. Sujatha, K. R. Ananthapadmanaban, "Data-Driven Insights into Gestational Diabetes Mellitus: Enhancing Models for Prediction by SVM Imputation for Personalized Pregnancy Care," SSRG International Journal of Electronics and Communication Engineering, vol. 11, no. 8, pp. 140-150, 2024. Crossref, https://doi.org/10.14445/23488549/IJECE-V11I8P115
Abstract:
Gestational Diabetes Mellitus(GDM) stands as a vital health concern for pregnant individuals worldwide. The onset or detection of elevated blood sugar levels during pregnancy, representing a form of glucose intolerance, characterizes it. The implications of GDM extend beyond maternal health, as it also poses risks to the developing fetus, potentially leading to adverse outcomes such as macrosomia, birth injuries, and an increased likelihood of caesarean delivery. Machine learning helps overcome the mentioned problems. This work evaluates the performance of different machine learning models as well as compares them with an existing system, particularly the K-Nearest Neighbors (KNN) model, in predicting GDM during pregnancy. This evaluation aims to determine whether KNN outperforms alternative models in accurately predicting GDM. The dataset contains 3525 records with 17 attributes, of which 16 are independent attributes, and one is an outcome attribute. For preprocessing these records, the SVM imputation method is implemented to replace missing records in the dataset. The KNN of the Lazy category produces an effective result with an accuracy of 96.96%, 97% precision, and 97% recall, which is an efficient result, and the Decision Table demonstrates the lowest efficiency with 95.97% accuracy, 96% precision, and 96% recall. The proposed system of the KNN model gives 96.96% accuracy, 97% precision, 97% recall, 97% F1-Score, 0.03 deviations, and 0.01 seconds of time complexity, whereas the existing KNN model had 85% accuracy, 83% precision, 84.96% recall, 84% F1-Score, 0.1503 errors, and 0.5355 seconds of time complexity. The work assesses classification metrics and regression metrics on multilayer perceptron, random forest, Bayes net, decision table, and KNN models. The ultimate objective is to detect the most effective model for predicting GDM, which could improve the analysis and management of this medical complication during pregnancy.
Keywords:
Gestational diabetes mellitus, KNN, MLP, Decision table, Random forest.
References:
[1] A. Sumathi, S. Meganathan, and B. Vijila Ravisankar, “An Intelligent Gestational Diabetes Diagnosis Model Using Deep Stacked Autoencoder,” Computers, Materials & Continua, vol. 69, no. 3, pp. 3109-3126, 2021.
[CrossRef] [Google Scholar] [Publisher Link]
[2] A. Sumathi, and S. Meganathan, “Ensemble Classifier Technique to Predict Gestational Diabetes Mellitus (GDM),” Computer Systems Science and Engineering, vol. 40, no.1, pp. 313-325, 2022.
[CrossRef] [Google Scholar] [Publisher Link]
[3] A. Ghasemi, S. Zahediasl, and F. Azizi, “Application of Machine Learning to Predict Gestational Diabetes Mellitus Risk,” Journal of Diabetes Investigation, vol. 9, no. 5, pp. 1105-1113, 2018.
[4] C. Laine, S.R. Bailey, and J. Ma, “A Machine Learning-Based Model for Individualized Prediction of Gestational Diabetes Mellitus Risk,” Plos One, vol. 15, no. 7, 2020.
[5] M.T. Segura, and A. Sanchez, “Application of Machine Learning Techniques for Prediction of Gestational Diabetes Mellitus: A Systematic Review,” Medicine, vol. 57, no. 1, 2021.
[6] Grzegorz Żabiński et al., “Multiclassifier Majority Voting Analyses in Provenance Studies on Iron Artifacts,” Journal of Archaeological Science, vol. 113, pp. 1-15, 2020.
[CrossRef] [Google Scholar] [Publisher Link]
[7] Gabriel Cubillos et al. “Development of Machine Learning Models to Predict Gestational Diabetes Risk in the First Half of Pregnancy,” BMC Pregnancy Childbirth, vol. 23, pp. 1-18, 2023.
[CrossRef] [Google Scholar] [Publisher Link]
[8] Yi-Xin Li et al., “Prediction of Gestational Diabetes Mellitus at the First Trimester: Machine-Learning Algorithms,” Archives of Gynecology and Obstetrics, vol. 309, pp. 2557-2566, 2023.
[CrossRef] [Google Scholar] [Publisher Link]
[9] Iswaria Gnanadass, “Prediction of Gestational Diabetes by Machine Learning Algorithms,” IEEE Potentials, vol. 39, no. 6, pp. 32-37, 2020.
[CrossRef] [Google Scholar] [Publisher Link]
[10] Masahiro Watanabe et al., “Prediction of Gestational Diabetes Mellitus Using Machine Learning from Birth Cohort Data of the Japan Environment and Children's Study,” Scientific Reports, vol. 13, no. 1, pp. 1-11, 2023.
[CrossRef] [Google Scholar] [Publisher Link]
[11] Go Uchitachimoto et al., “Data Collaboration Analysis in Predicting Diabetes from a Small Amount of Health Checkup Data,” Scientific Reports, vol. 13, no. 1, pp. 1-8, 2023.
[CrossRef] [Google Scholar] [Publisher Link]
[12] Ashwini Tuppad, and Shantala Devi Patil, “Machine Learning for Diabetes Clinical Decision Support: A Review,” Advances in Computational Intelligence, vol. 2, pp. 1-24, 2022.
[CrossRef] [Google Scholar] [Publisher Link]
[13] Xiaoqi Hu et al., “Prediction Model for Gestational Diabetes Mellitus Using the XG Boost Machine Learning Algorithm,” Frontiers in Endocrinology, vol. 14, pp. 1-10, 2023.
[CrossRef] [Google Scholar] [Publisher Link]
[14] Burçin Kurt et al., “Prediction of Gestational Diabetes Using Deep Learning and Bayesian Optimization and Traditional Machine Learning Techniques,” Medical & Biological Engineering & Computing, vol. 61, pp. 1649-1660, 2023.
[CrossRef] [Google Scholar] [Publisher Link]
[15] Yipeng Wang et.al, “Identify Gestational Diabetes Mellitus by Deep Learning Model from Cell-Free DNA at the Early Gestation Stage,” Briefings in Bioinformatics, vol. 25, no. 1, pp. 1-13, 2024.
[CrossRef] [Google Scholar] [Publisher Link]
[16] Mohammad Shahin et al., “Using Machine Learning and Deep Learning Algorithms for Downtime Minimization in Manufacturing Systems: An Early Failure Detection Diagnostic Service,” The International Journal of Advanced Manufacturing Technology, vol. 128, pp. 3857-3883, 2023.
[CrossRef] [Google Scholar] [Publisher Link]
[17] Zheqing Zhang et al., “Machine Learning Prediction Models for Gestational Diabetes Mellitus: Meta-Analysis,” Journal of Medical Internet Research, vol. 24, no. 3, pp. 1-15, 2022.
[CrossRef] [Google Scholar] [Publisher Link]
[18] Hosam El-Sofany et al., “A Proposed Technique Using Machine Learning for the Prediction of Diabetes Disease through a Mobile App,” International Journal of Intelligent Systems, vol. 2024, no. 1, pp. 1-13, 2024.
[CrossRef] [Google Scholar] [Publisher Link]
[19] Yuhan Du et al., “An Explainable Machine Learning-Based Clinical Decision Support System for Prediction of Gestational Diabetes Mellitus,” Scientific Reports, vol. 12, pp. 1-14, 2022.
[CrossRef] [Google Scholar] [Publisher Link]
[20] Chun-Yang Chou, Ding-Yang Hsu, and Chun-Hung Chou, “Predicting the Onset of Diabetes with Machine Learning Methods,” Journal of Personalized Medicine, vol. 13, no. 3, pp. 1-18, 2023.
[CrossRef] [Google Scholar] [Publisher Link]
[21] Sumathi Amarnath, Meganathan Selvamani, and Vijayakumar Varadarajan, “Prognosis Model for Gestational Diabetes using Machine Learning Techniques,” Sensors and Materials, vol. 33, no. 9, pp. 3011-3025, 2021.
[CrossRef] [Google Scholar] [Publisher Link]
[22] Daniela Mennickent et al., “Machine Learning-Based Models for Gestational Diabetes Mellitus Prediction before 24-28 Weeks of Pregnancy: A Review,” Artificial Intelligence in Medicine, vol. 132, 2022.
[CrossRef] [Google Scholar] [Publisher Link]
[23] P. Nagaraj, and P. Deepalakshmi, “Diabetes Prediction Using Enhanced SVM and Deep Neural Network Learning Techniques: An Algorithmic Approach for Early Screening of Diabetes,” International Journal of Healthcare Information Systems and Informatics, vol. 16, no. 4, pp. 1-20, 2021.
[CrossRef] [Google Scholar] [Publisher Link]
[24] Bruno Basil et al., “A First Trimester Prediction Model and Nomogram for Gestational Diabetes Mellitus Based on Maternal Clinical Risk Factors in a Resource-Poor Setting,” BMC Pregnancy Childbirth, vol. 24, no. 1, pp. 1-8, 346.
[CrossRef] [Google Scholar] [Publisher Link]
[25] Sumathi Santhosh, Gestational Diabetes Mellitus (GDM Data Set), Kaggle, 2022. [Online]. Available: https://www.kaggle.com/datasets/sumathisanthosh/gestational-diabetes-mellitus-gdm-data-set