Heterogeneous Sketch-Face Photo Recognition in Forensic Science Laboratories
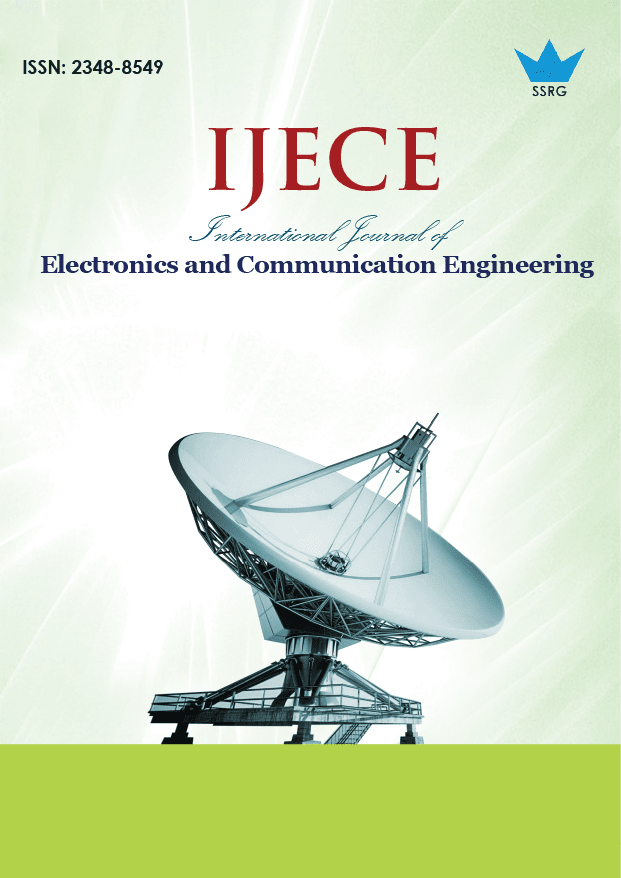
International Journal of Electronics and Communication Engineering |
© 2024 by SSRG - IJECE Journal |
Volume 11 Issue 8 |
Year of Publication : 2024 |
Authors : Devendra A. Itole, M. P.Sardey, Milind P. Gajare |
How to Cite?
Devendra A. Itole, M. P.Sardey, Milind P. Gajare, "Heterogeneous Sketch-Face Photo Recognition in Forensic Science Laboratories," SSRG International Journal of Electronics and Communication Engineering, vol. 11, no. 8, pp. 260-268, 2024. Crossref, https://doi.org/10.14445/23488549/IJECE-V11I8P125
Abstract:
This research presents a pioneering framework, termed X-Bridge, aimed at automating the identification of diverse faces through facial sketches. The significance of this framework lies in its potential applications in security and surveillance domains. The study advances the field by a. Conducting an in-depth analysis of conventional neural network architectures utilized for image classification, particularly focusing on their effectiveness in facial recognition tasks. b. Investigating the latest parameters essential for accurate facial recognition and their integration into various neural network structures to enhance performance. c. Assessing potential cross-modal connections that could facilitate more robust facial recognition systems.d. Introducing a novel Generative Adversarial Network (GAN)-based strategy, X-Bridge, specifically tailored to surpass existing standards on a meticulously curated dataset dedicated to facial recognition. Through these endeavors, the XBridge framework exceeds current benchmarks, demonstrating its efficacy in automating facial recognition tasks. This research contributes to the advancement of automated facial recognition technology, offering promising implications for security and surveillance applications.
Keywords:
Cross-modal bridge, Heterogeneous face identification, Image-to-sketch conversion, Machine learning, Artificial neural networks, Categorization, Validation, Identifying.
References:
[1] Ishaan Gulrajani et al., “Improved Training of Wasserstein Gans,” Proceedings of the 31st International Conference on Neural Information Processing Systems, Long Beach California, USA, pp. 5769-5779, 2017.
[Google Scholar] [Publisher Link]
[2] Anders Boesen Lindbo Larsen et al., “Autoencoding Beyond Pixels Using a Learned Similarity Metric,” Proceedings of The 33rd International Conference on Machine Learning, vol. 48, pp. 1558-1566, 2016.
[Google Scholar] [Publisher Link]
[3] Ivan Gruber, “Generating Facial Images Using Vaegan,” Student Scientific Conference, pp. 38-39, 2018.
[Google Scholar] [Publisher Link]
[4] Mehdi Mirza, and Simon Osindero, “Conditional Generative Adversarial Nets,” arXiv, pp. 1-7, 2014.
[CrossRef] [Google Scholar] [Publisher Link]
[5] Diederik P. Kingma, and Max Welling, “Auto-Encoding Variational Bayes,” arXiv, pp. 1-14, 2013.
[CrossRef] [Google Scholar] [Publisher Link]
[6] R. Hadsell, S. Chopra, and Y. LeCun, “Dimensionality Reduction by Learning an Invariant Mapping,” 2006 IEEE Computer Society Conference on Computer Vision and Pattern Recognition (CVPR’06), New York, NY, USA, pp. 1735-1742, 2006.
[CrossRef] [Google Scholar] [Publisher Link]
[7] Yi Sun et al., “Deep Learning Face Representation by Joint Identification-Verification,” Proceedings of the 27th International Conference on Neural Information Processing Systems, Montreal Canada, vol. 2, pp. 1988-1996, 2014.
[Google Scholar] [Publisher Link]
[8] Florian Schroff, Dmitry Kalenichenko, and James Philbin, “FaceNet: A Unified Embedding for Face Recognition and Clustering,” 2015 IEEE Conference on Computer Vision and Pattern Recognition (CVPR), Boston, MA, USA, pp. 815-823, 2015.
[CrossRef] [Google Scholar] [Publisher Link]
[9] Yandong Wen et al., “A Discriminative Feature Learning Approach for Deep Face Recognition,” Computer Vision–ECCV 2016: 14th European Conference, Amsterdam, The Netherlands, pp. 499-515, 2016.
[CrossRef] [Google Scholar] [Publisher Link]
[10] Yu Liu, Hongyang Li, and Xiaogang Wang, “Learning Deep Features via Congenerous Cosine Loss for Person Recognition,” arXiv, pp. 1-7, 2017.
[CrossRef] [Google Scholar] [Publisher Link]
[11] Jiankang Deng et al., “Arcface: Additive Angular Margin Loss for Deep Face Recognition,” 2019 IEEE/CVF Conference on Computer Vision and Pattern Recognition (CVPR), Long Beach, CA, USA, pp. 4685-4694, 2019.
[CrossRef] [Google Scholar] [Publisher Link]
[12] Rajeev Ranjan, Carlos D. Castillo, and Rama Chellappa, “L2-Constrained Softmax Loss for Discriminative Face Verification,” arXiv, pp. 1-10, 2017.
[CrossRef] [Google Scholar] [Publisher Link]
[13] Feng Wang et al., “Normface: L2 Hypersphere Embedding for Face Verification,” Proceedings of the 25th ACM International Conference on Multimedia, Mountain View California, USA, pp. 1041-1049, 2017.
[CrossRef] [Google Scholar] [Publisher Link]
[14] Hao Wang et al., “Cosface: Large Margin Cosine Loss for Deep Face Recognition,” 2018 IEEE/CVF Conference on Computer Vision and Pattern Recognition, Salt Lake City, UT, USA, pp. 5265-5274, 2018.
[CrossRef] [Google Scholar] [Publisher Link]
[15] Feng Wang et al., “Additive Margin Softmax for Face Verification,” IEEE Signal Processing Letters, vol. 25, no. 7, pp. 926-930, 2018.
[CrossRef] [Google Scholar] [Publisher Link]
[16] A Beginner’s Guide to Generative Adversarial Networks (GANS), Skymind. [Online]. Available: https://skymind.com/wiki/generative-adversarial-network-gan
[17] Cs231n Convolution Neural Networks for Visual Recognition. [Online]. Available: http://cs231n.github.io/
[18] "Neuronov´e s´ıtˇe." [Online]. Available: http://www.kky.zcu.cz/cs/courses/neu
[19] T. Kohonen, M. Schroeder, and T. Huang, Self-Organizing Maps, 3rd ed., Springer-Verlag New York, Secaucus, NJ, USA, 2001.
[Publisher Link]
[20] Lior Wolf, Tal Hassner, and Itay Maoz, “Face Recognition in Unconstrained Videos with Matched Background Similarity,” Proceedings of the 2011 IEEE Conference on Computer Vision and Pattern Recognition, Colorado Springs, CO, USA, pp. 529-534, 2011.
[CrossRef] [Google Scholar] [Publisher Link]
[21] Ralph Gross et al., “Multi-Pie,” Image and Vision Computing, vol. 28, no. 5, pp. 807-813, 2010.
[CrossRef] [Google Scholar] [Publisher Link]
[22] Yaniv Taigman et al., “Deepface: Closing the Gap to Human-Level Performance in Face Verification,” 2014 IEEE Conference on Computer Vision and Pattern Recognition, Columbus, OH, USA, pp. 1701-1708, 2014.
[CrossRef] [Google Scholar] [Publisher Link]
[23] Wen Gao et al., “The CAS-PEAL Large-Scale Chinese Face Database and Baseline Evaluations.” IEEE Transactions on Systems, Man, and Cybernetics- Part A: Systems and Humans, vol. 38, no. 1, pp. 149-161, 2008.
[CrossRef] [Google Scholar] [Publisher Link]
[24] Zhiwu Huang et al., “A Benchmark and Comparative Study of Video-Based Face Recognition on Cox Face Database,” IEEE Transactions on Image Processing, vol. 24, no. 12, pp. 5967-5981, 2015.
[CrossRef] [Google Scholar] [Publisher Link]