Optimized Schizophrenia Detection via EEG: A CNN-LSTM and CNN-GRU Ensemble with SVM
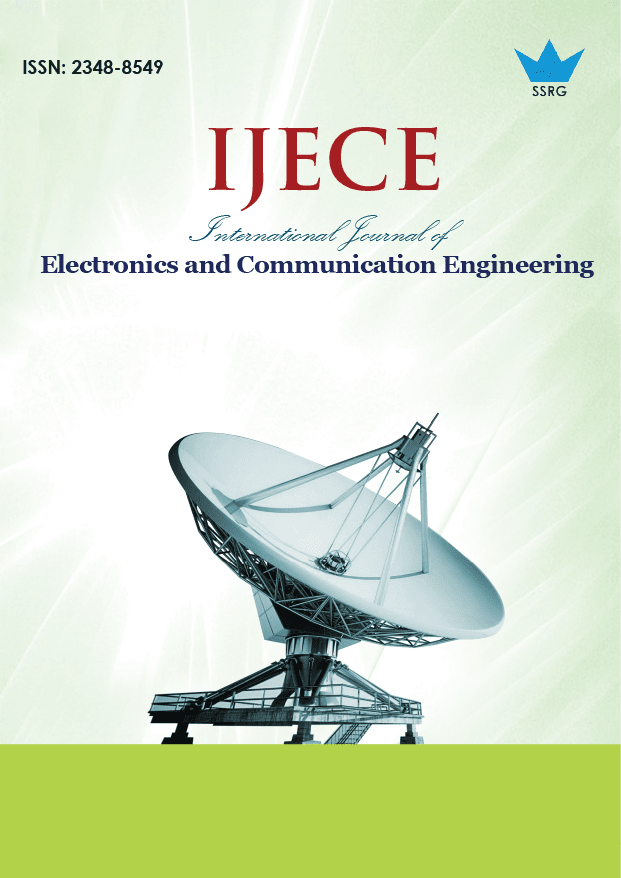
International Journal of Electronics and Communication Engineering |
© 2024 by SSRG - IJECE Journal |
Volume 11 Issue 8 |
Year of Publication : 2024 |
Authors : N. Shan, V. Amsaveni |
How to Cite?
N. Shan, V. Amsaveni, "Optimized Schizophrenia Detection via EEG: A CNN-LSTM and CNN-GRU Ensemble with SVM," SSRG International Journal of Electronics and Communication Engineering, vol. 11, no. 8, pp. 269-281, 2024. Crossref, https://doi.org/10.14445/23488549/IJECE-V11I8P126
Abstract:
Human illnesses that impact a person's ability to think, communicate, and behave are primarily classified as psychological and neurological disorders. Currently, neurological illnesses affect almost 12% of the world's population. About 1% of people worldwide have schizophrenia, a chronic psychological illness. The proposed ensemble model combines two hybrid Deep Learning (DL) approaches with the Support Vector Machine (SVM) as the meta learner. Convolutional Neural NetworkGated Recurrent Unit (CNN-GRU) and Convolutional Neural Network-Long Short-Term Memory (CNN-LSTM) are the DL approaches utilized in the ensemble approach for Schizophrenia detection. The EEG signals utilized in this study are sourced from the Kaggle repository, an extensive online database known for its diverse collection of high-quality medical data. This novel DL model for schizophrenia classification aims to enhance diagnostic accuracy and reduce reliance on expert interpretation. The first hybrid model, CNN-LSTM, attains an accuracy of 92.93% and CNN-GRU with an accuracy of 93.10%. Thus, the staking ensemble model achieves an overall accuracy of 94.85%, indicating strong overall correctness in classifying cases. Comparative simulations of the suggested approach against several current solutions in schizophrenia diagnosis reveal that the suggested approach attains superior accuracy when juxtaposed with other existing methods. These findings bolster the advantages of DL for neuroscientific research in general and psychiatric classification in particular.
Keywords:
Schizophrenia, Gated recurrent unit, Psychological disease, Long short-term memory, Convolutional neural network, Support vector machine.
References:
[1] Anne Berghöfer et al., “Quality of Life in Patients with Severe Mental Illness: A Cross-Sectional Survey in an Integrated Outpatient Health Care Model,” Quality of Life Research, vol. 29, pp. 2073-2087, 2020.
[CrossRef] [Google Scholar] [Publisher Link]
[2] Vanteemar S. Sreeraj et al., “Effect of Add-On Transcranial Alternating Current Stimulation (tACS) on Persistent Delusions in Schizophrenia,” Psychiatry Research, vol. 290, 2020.
[CrossRef] [Google Scholar] [Publisher Link]
[3] Sher Singh, Deepa Khanna, and Sanjeev Kalra, “Role of Neurochemicals in Schizophrenia,” Current Psychopharmacology, vol. 9, no. 2, pp. 144-161, 2020.
[CrossRef] [Google Scholar] [Publisher Link]
[4] Sergey N. Mosolov, and Polina A. Yaltonskaya, “Primary and Secondary Negative Symptoms in Schizophrenia,” Frontiers in Psychiatry, vol. 12, pp. 1-12, 2022.
[CrossRef] [Google Scholar] [Publisher Link]
[5] Ayşe Gül Eker, Nevcihan Duru, and Kadir Eker, “EEG Signals and Spectrogram with Deep Learning Approaches Emotion Analysis with Images,” 2022 7th International Conference on Computer Science and Engineering (UBMK), Diyarbakir, Turkey, pp. 1-5, 2022.
[CrossRef] [Google Scholar] [Publisher Link]
[6] Robert A. McCutcheon, John H. Krystal, and Oliver D. Howes, “Dopamine and Glutamate in Schizophrenia: Biology, Symptoms and Treatment,” World Psychiatry, vol. 19, no. 1, pp. 15-33, 2020.
[CrossRef] [Google Scholar] [Publisher Link]
[7] Zülfikar Aslan, and Mehmet Akin, “A Deep Learning Approach in Automated Detection of Schizophrenia Using Scalogram Images of EEG Signals,” Physical and Engineering Sciences in Medicine, vol. 45, no. 1, pp. 83-96, 2022.
[CrossRef] [Google Scholar] [Publisher Link]
[8] Aleksander Wawer et al., “Single and Cross-Disorder Detection for Autism and Schizophrenia,” Cognitive Computation, vol. 14, pp. 461- 473, 2022.
[CrossRef] [Google Scholar] [Publisher Link]
[9] Carla Barros et al., “From Sound Perception to Automatic Detection of Schizophrenia: An EEG-Based Deep Learning Approach,” Frontiers in Psychiatry, vol. 12, pp. 1-17, 2022.
[CrossRef] [Google Scholar] [Publisher Link]
[10] Mengjiao Hu et al., “Structural and Diffusion MRI based Schizophrenia Classification using 2D Pretrained and 3D Naive Convolutional Neural Networks,” Schizophrenia Research, vol. 243, pp. 330-341, 2022.
[CrossRef] [Google Scholar] [Publisher Link]
[11] P. Supriya Patro et al., “Lightweight 3D Convolutional Neural Network for Schizophrenia Diagnosis Using MRI Images and Ensemble Bagging Classifier,” arXiv, pp. 1-25, 2022.
[CrossRef] [Google Scholar] [Publisher Link]
[12] Jihoon Oh et al., “Identifying Schizophrenia using Structural MRI with a Deep Learning Algorithm,” Frontiers in Psychiatry, vol. 11, pp. 1-11, 2020.
[CrossRef] [Google Scholar] [Publisher Link]
[13] Smith K. Khare, Varun Bajaj, and U. Rajendra Acharya, “SPWVD-CNN for Automated Detection of Schizophrenia Patients Using EEG Signals,” IEEE Transactions on Instrumentation and Measurement, vol. 70, pp. 1-9, 2021.
[CrossRef] [Google Scholar] [Publisher Link]
[14] JinChi Zheng et al., “Diagnosis of Schizophrenia Based on Deep Learning Using fMRI,” Computational and Mathematical Methods in Medicine, vol. 2021, no. 1, pp. 1-7, 2021.
[CrossRef] [Google Scholar] [Publisher Link]
[15] Yesh Khandelwal, Ensemble Stacking for Machine Learning and Deep Learning, Analytics Vidhya, 2021. [Online]. Available: https://www.analyticsvidhya.com/blog/2021/08/ensemble-stacking-for-machine-learning-and-deep-learning/
[16] Yan Holtz, Heatmap, Data-to-Viz.com. [Online]. Available: https://www.data-to-viz.com/graph/heatmap.html
[17] Chapter 1: Introduction to EEG Spectrograms, Atlas of Intensive Care Quantitative EEG, Springer Publishing Company, 2023.
[CrossRef] [Publisher Link]