Improved Glioma Detection and Classification through the EVGG19 Model
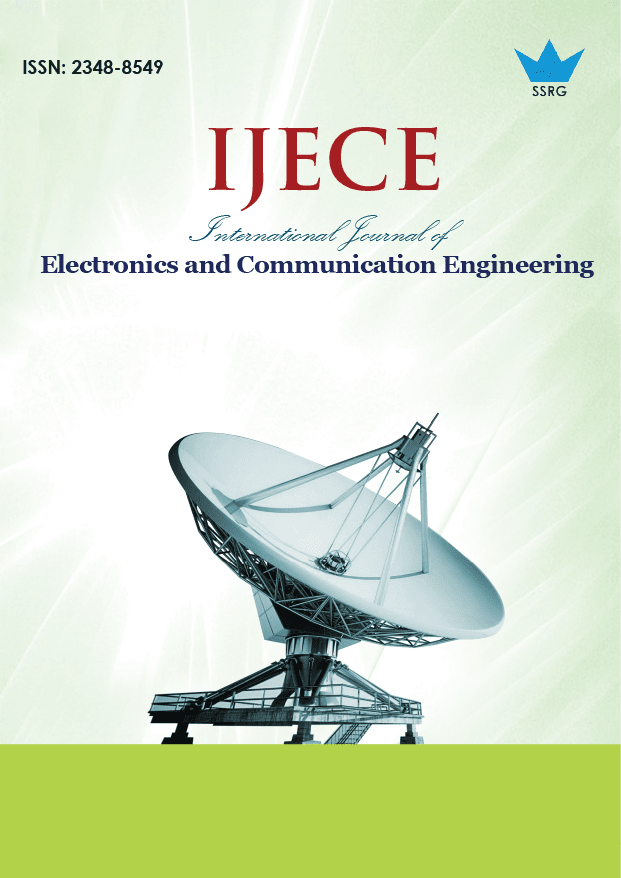
International Journal of Electronics and Communication Engineering |
© 2024 by SSRG - IJECE Journal |
Volume 11 Issue 8 |
Year of Publication : 2024 |
Authors : S. Kannan, S. Anusuya |
How to Cite?
S. Kannan, S. Anusuya, "Improved Glioma Detection and Classification through the EVGG19 Model," SSRG International Journal of Electronics and Communication Engineering, vol. 11, no. 8, pp. 282-293, 2024. Crossref, https://doi.org/10.14445/23488549/IJECE-V11I8P127
Abstract:
Gliomas, a diverse and complex category of brain tumors, present significant challenges in accurate classification due to their heterogeneous nature. Precise classification of gliomas into their respective subtypes and grades is crucial for effective clinical decision-making and personalized treatment planning. This study proposes an enhanced convolutional neural network architecture, EVGG19, designed specifically for the classification of gliomas using MRI data. This effort aims to incorporate domain-specific innovations and improve the glioma classification's accuracy and reliability. Our proposed workflow begins with the preprocessing and normalization of MRI images, followed by utilizing the DHA-ISSP model for accurate tumor segmentation. The segmented tumor regions are then fed into the EVGG19 model, which includes additional convolutional layers, increased model depth, dropout regularization, and a dedicated classification layer to refine the extraction and representation of features relevant to glioma classification. The performance of the EVGG19 model was rigorously evaluated using the TCGA dataset. Our model achieved an accuracy of 0.94, precision of 0.89, recall of 0.91, and F1 score of 0.9, significantly outperforming existing baseline models such as VGG Net-Based Deep Learning, UNet-VGG16 with transfer learning and VGG-UNET. Furthermore, EVGG19 demonstrated superior specificity (0.96), sensitivity (0.93), and AUC (0.97), along with the lowest MAE of 0.1 and MSE of 0.2. These findings demonstrate how well the EVGG19 model can distinguish glioma grades and subtypes, providing a robust tool for clinical application and furthering the potential for improved patient outcomes through more precise diagnostic capabilities.
Keywords:
Gliomas, Classification, EVGG19, Segmentation, Normalization, Tumor regions.
References:
[1] Muhammad Attique Khan et al., “Multimodal Brain Tumor Classification Using Deep Learning and Robust Feature Selection: A Machine Learning Application for Radiologists,” Diagnostics, vol. 10, no. 8, pp. 1-19, 2020.
[CrossRef] [Google Scholar] [Publisher Link]
[2] Dillip Ranjan Nayak, “Brain Tumor Classification Using Dense Efficient-Net,” Axioms, vol. 11, no. 1, pp. 1-13, 2022.
[CrossRef] [Google Scholar] [Publisher Link]
[3] P. Kavitha et al., “Brain Tumor Detection for Efficient Adaptation and Superior Diagnostic Precision by Utilizing MBConv-Finetuned-B0 and Advanced Deep Learning,” International Journal of Intelligent Engineering and Systems, vol. 17, no. 2, pp. 632-644, 2023.
[CrossRef] [Google Scholar] [Publisher Link]
[4] Champakamala Sundar Rao, and K. Karunakara, “Efficient Detection and Classification of Brain Tumor Using Kernel Based SVM for MRI,” Multimedia Tools and Applications, vol. 81, pp. 7393–7417, 2022.
[CrossRef] [Google Scholar] [Publisher Link]
[5] D. Dhinakaran et al., “Leveraging Semi-Supervised Graph Learning for Enhanced Diabetic Retinopathy Detection,” SSRG International Journal of Electronics and Communication Engineering, vol. 10, no. 8, pp. 9-21, 2023.
[CrossRef] [Google Scholar] [Publisher Link]
[6] Saif Ahmad, and Pallab K. Choudhury, “On the Performance of Deep Transfer Learning Networks for Brain Tumor Detection Using MR Images,” IEEE Access, vol. 10, pp. 59099-59114, 2022.
[CrossRef] [Google Scholar] [Publisher Link]
[7] Viet Huan Le et al., “Development and Validation of CT-Based Radiomics Signature for Overall Survival Prediction in Multi-organ Cancer,” Journal of Digital Imaging, vol. 36, pp. 911–922, 2023.
[CrossRef] [Google Scholar] [Publisher Link]
[8] Mohammad Shahjahan Majib et al., “VGG-SCNet: A VGG Net-Based Deep Learning Framework for Brain Tumor Detection on MRI Images,” IEEE Access, vol. 9, pp. 116942-116952, 2021.
[CrossRef] [Google Scholar] [Publisher Link]
[9] Javeria Amin et al., “A New Model for Brain Tumor Detection Using Ensemble Transfer Learning and Quantum Variational Classifier,” Computational Intelligence and Neuroscience, vol. 2022, no. 1, pp. 1-13, 2022.
[CrossRef] [Google Scholar] [Publisher Link]
[10] Shahzad Ahmad Qureshi et al., “Intelligent Ultra-Light Deep Learning Model for Multi-Class Brain Tumor Detection,” Applied Sciences, vol. 12, no. 8, pp. 1-22, 2022.
[CrossRef] [Google Scholar] [Publisher Link]
[11] Anindya Apriliyanti Pravitasari et al., “UNet-VGG16 with Transfer Learning for MRI-Based Brain Tumor Segmentation,” Telkomnika (Telecommunication, Computing, Electronics and Control), vol. 18, no. 3, pp. 1310-1318, 2020.
[CrossRef] [Google Scholar] [Publisher Link]
[12] Shtwai Alsubai et al., “Ensemble Deep Learning for Brain Tumor Detection,” Frontiers in Computational Neuroscience, vol. 16, pp. 1- 14, 2022.
[CrossRef] [Google Scholar] [Publisher Link]
[13] Saikat Islam Khan et al., “Accurate Brain Tumor Detection Using Deep Convolutional Neural Network,” Computational and Structural Biotechnology Journal, vol. 20, pp. 4733–4745, 2022.
[CrossRef] [Google Scholar] [Publisher Link]
[14] Ali Nawaz et al., “VGG-UNET for Brain Tumor Segmentation and Ensemble Model for Survival Prediction,” 2021 International Conference on Robotics and Automation in Industry (ICRAI), Rawalpindi, Pakistan, pp. 1-6, 2021.
[CrossRef] [Google Scholar] [Publisher Link]
[15] Amjad Rehman et al., “Microscopic Brain Tumor Detection and Classification Using 3D CNN and Feature Selection Architecture,” Microscopy Research and Technique, vol. 84, no. 1, pp. 133–149, 2021.
[CrossRef] [Google Scholar] [Publisher Link]
[16] Aryan Sagar Methil, “Brain Tumor Detection Using Deep Learning and Image Processing,” 2021 International Conference on Artificial Intelligence and Smart Systems (ICAIS), Coimbatore, India, pp. 100-108, 2021.
[CrossRef] [Google Scholar] [Publisher Link]
[17] Haitham Alsaif et al., “A Novel Data Augmentation-Based Brain Tumor Detection Using Convolutional Neural Network,” Applied Sciences, vol. 12, no. 8, pp. 1-16, 2022.
[CrossRef] [Google Scholar] [Publisher Link]
[18] Hasnain Ali Shah et al., “A Robust Approach for Brain Tumor Detection in Magnetic Resonance Images Using Finetuned EfficientNet,” IEEE Access, vol. 10, pp. 65426-65438, 2022.
[CrossRef] [Google Scholar] [Publisher Link]
[19] Rajeev Kumar Gupta et al., “Brain Tumor Detection and Classification Using Cycle Generative Adversarial Networks,” Interdisciplinary Sciences, Computational Life Sciences, vol. 14, no. 2, pp. 485–502, 2022.
[CrossRef] [Google Scholar] [Publisher Link]
[20] Emrah Irmak, “Multi-Classification of Brain Tumor MRI Images Using Deep Convolutional Neural Network with Fully Optimized Framework,” Iranian Journal of Science and Technology, Transactions of Electrical Engineering, vol. 45, pp. 1015–1036, 2021.
[CrossRef] [Google Scholar] [Publisher Link]
[21] Ramin Ranjbarzadeh et al., “Brain Tumor Segmentation Based On Deep Learning and an Attention Mechanism Using MRI Mult-Modalities Brain Images,” Scientific Reports, vol. 11, 2021.
[CrossRef] [Google Scholar] [Publisher Link]
[22] G. Prabaharan et al., “AI-Enhanced Comprehensive Liver Tumor Prediction Using Convolutional Autoencoder and Genomic Signatures,” International Journal of Advanced Computer Science and Applications, vol. 15, no. 2, 2024.
[CrossRef] [Google Scholar] [Publisher Link]
[23] Mohamed Ait Amou et al., “A Novel MRI Diagnosis Method for Brain Tumor Classification Based on CNN and Bayesian Optimization,” Healthcare, vol. 10, no. 3, pp. 1-21, 2022.
[CrossRef] [Google Scholar] [Publisher Link]
[24] R. Ramani et al., “Integrated Normal Discriminant Analysis in Mapreduce for Diabetic Chronic Disease Prediction Using Bivariant Deep Neural Networks,” International Journal of Information Technology, pp. 1-15, 2024.
[CrossRef] [Google Scholar] [Publisher Link]
[25] Abdu Gumaei et al., “A Hybrid Feature Extraction Method with Regularized Extreme Learning Machine for Brain Tumor Classification,” IEEE Access, vol. 7, pp. 36266-36273, 2019.
[CrossRef] [Google Scholar] [Publisher Link]