Content Based Image Retrieval using Spectral Feature Extraction Methods
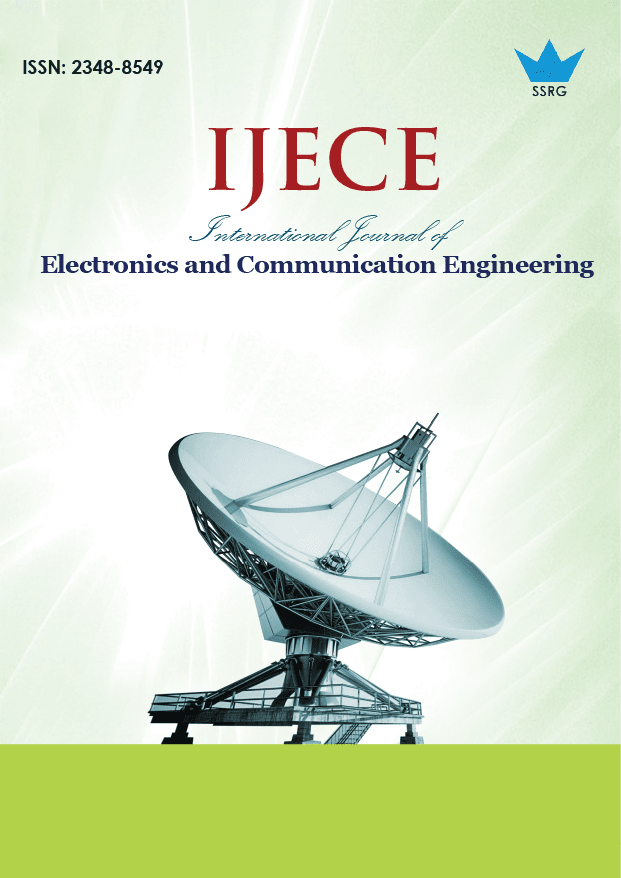
International Journal of Electronics and Communication Engineering |
© 2015 by SSRG - IJECE Journal |
Volume 2 Issue 4 |
Year of Publication : 2015 |
Authors : Nawade Rajlaxmi and Dr.Mrs.Lokhande S.S |
How to Cite?
Nawade Rajlaxmi and Dr.Mrs.Lokhande S.S, "Content Based Image Retrieval using Spectral Feature Extraction Methods," SSRG International Journal of Electronics and Communication Engineering, vol. 2, no. 4, pp. 10-13, 2015. Crossref, https://doi.org/10.14445/23488549/IJECE-V2I4P104
Abstract:
Content-based image retrieval (CBIR) is a technique which uses visual content to search and compare images from large scale image databases according to the interests of users. Visual content of the image like color, shape, texture and spatial layout are widely used in CBIR. Texture features has been successfully used to provide a meaningful tool for searching image databases, as texture images generally contain unique visual patterns or spatial arrangements of pixels, so that describing textures, gray- level or color alone, may not yield to classify similar ones. In this paper we mainly concentrate on Spectral methods which extract texture features from the energy distribution in the frequency domain. The most popular spectral feature extraction methods are Fourier, wavelet, and Gabor filtering. In the first process it will extract the features from query and database images to a distinguishable extent using Gabor and wavelet Transforms. In the second process it involves matching these features to yield a result that is visually similar by feature matching process.
Keywords:
CBIR, Texture, Feature extraction, Gabor transform, Wavelet transform
References:
[1] F. Long, H. Zhang and D. Dagan Feng., "Fundamentals of Content-Based Image Retrieval". Multimedia Information Retrieval and Management–Technological Fundamentals and Applications, Springer-Verlag, pp. 1-26, 2003.
[2] Y. Rui and T.S. Huang . 1999, 10, pp. 39–62. “Image Retrieval: current techniques, promising directions, andOpen issues, Visual Commun, Image Representation
[3] P. Wu, B. S. Manjunath, S. Newsam, and H.D. Shin. A texture descriptor for browsing and similarity retrieval. Journal of Signal Processing: Image Communication, 16(1-2):33{43, 2000.
[4] M. Pietikainen, T. Ojala, and Z. Xu. Rotation invariant texture classification using feature distributions. Pattern Recognition, 33:43{52, 2000.
[5] Wan Siti Halimatul Munirah Wan Ahmad and Mohammad Faizal Ahmad Fauzi, 2008,” Comparison of Different Feature Extraction Techniques in Content- Based Image Retrieval for CT Brain images”, IEEE,
[6] J. Weszka, C. Dyer, and A. Rosenfold. A comparative study of texture measures for terrain classification. IEEE Transaction on System, Man and Cybernetics, 6(4):269{285, 1976.
[7] W. Y. Ma and B. S. Manjunath. A comparision of wavelet transform features for texture image annotation. In Proc. of IEEE International Conference on Image Processing, pages II:256{259, 1995.