Attention Based Fused CNN for the Early Prediction of Gastrointestinal Disease
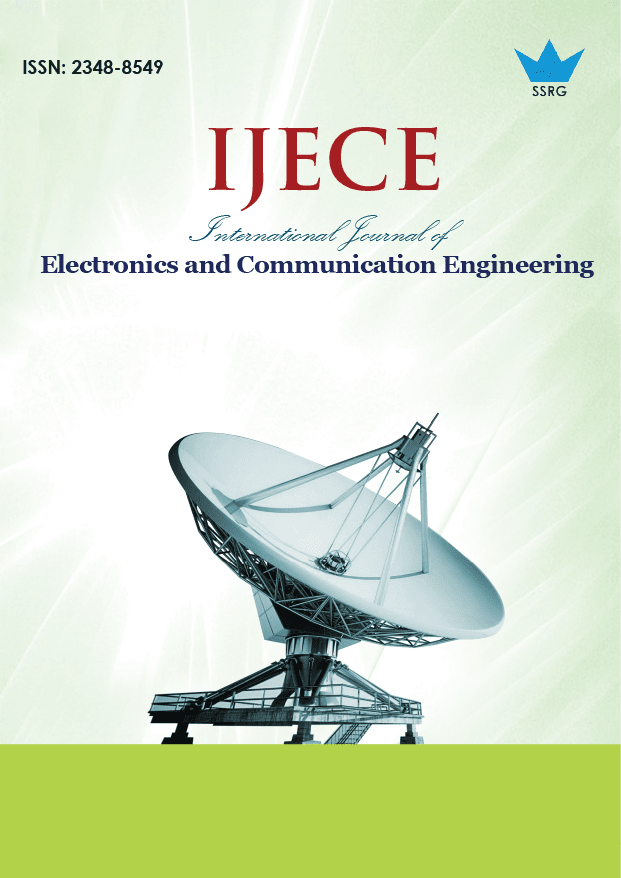
International Journal of Electronics and Communication Engineering |
© 2024 by SSRG - IJECE Journal |
Volume 11 Issue 8 |
Year of Publication : 2024 |
Authors : S. Mohanalakshmi, G. Hima Bindu, S. Esakki Rajavel, G. Sriram |
How to Cite?
S. Mohanalakshmi, G. Hima Bindu, S. Esakki Rajavel, G. Sriram, "Attention Based Fused CNN for the Early Prediction of Gastrointestinal Disease," SSRG International Journal of Electronics and Communication Engineering, vol. 11, no. 8, pp. 301-311, 2024. Crossref, https://doi.org/10.14445/23488549/IJECE-V11I8P129
Abstract:
This study proposes a standardized framework for early prediction of gastrointestinal disease diseases leveraging advanced image pre-processing, data augmentation, and an attention-based fused Convolutional Neural Network (CNN). The framework initiates meticulous image pre-processing, encompassing cropping, standardization and resizing methodologies to standardize image inputs and accentuate pertinent features. Following pre-processing, data augmentation strategies are implemented to diversify the training dataset, enhancing model robustness and generalization. By artificially generating diverse variations of the original images, data augmentation helps expand the training dataset. Techniques such as rotation, translation, scaling, and flipping are applied to augment the dataset, enabling the CNN algorithm to generalize better and capture a broader range of disease patterns. The key component of the framework is an attention-based fused CNN architecture designed to capture spatial and channel-wise dependencies within gastrointestinal images effectively. The attention mechanism allows the network to concentrate on informative regions while suppressing irrelevant noise, enhancing feature representation and classification performance. The experimental outcomes proved that the developed framework attains superior performance in the early prediction of gastrointestinal diseases with a high accuracy of 98.9%. The combination of image pre-processing, data augmentation and attention-based fused CNN contributes to improved accuracy and robustness.
Keywords:
Attention mechanism, Convolutional neural networks, Data augmentation, Early prediction, Gastrointestinal disease, Image pre-processing.
References:
[1] Furqan Rustam et al., “Wireless Capsule Endoscopy Bleeding Images Classification Using CNN Based Model,” IEEE Access, vol. 9, pp. 33675-33688, 2021.
[CrossRef] [Google Scholar] [Publisher Link]
[2] Dongxu Ye et al., “Design and Control of a Magnetically-Actuated Capsule Robot with Biopsy Function,” IEEE Transactions on Biomedical Engineering, vol. 69, no. 9, pp. 2905-2915, 2022.
[CrossRef] [Google Scholar] [Publisher Link]
[3] Xiaohan Xing, Yixuan Yuan, and Max Q.H. Meng, “Zoom in Lesions for Better Diagnosis: Attention Guided Deformation Network for WCE Image Classification,” IEEE Transactions on Medical Imaging, vol. 39, no. 12, pp. 4047-4059, 2020.
[CrossRef] [Google Scholar] [Publisher Link]
[4] Zhenyuan Ning et al., “Pattern Classification for Gastrointestinal Stromal Tumors by Integration of Radiomics and Deep Convolutional Features,” IEEE Journal of Biomedical and Health Informatics, vol. 23, no. 3, pp. 1181-1191, 2018.
[CrossRef] [Google Scholar] [Publisher Link]
[5] Tao Li, and Liling Long, “Imaging Examination and Quantitative Detection and Analysis of Gastrointestinal Diseases Based on Data Mining Technology,” Journal of Medical Systems, vol. 44, no. 1, 2020.
[CrossRef] [Google Scholar] [Publisher Link]
[6] Muhammad Attique Khan et al., “Computer-Aided Gastrointestinal Diseases Analysis from Wireless Capsule Endoscopy: A Framework of Best Features Selection,” IEEE Access, vol. 8, pp. 132850-132859, 2020.
[CrossRef] [Google Scholar] [Publisher Link]
[7] Mosleh Hmoud Al-Adhaileh et al., “Deep Learning Algorithms for Detection and Classification of Gastrointestinal Diseases,” Complexity, vol. 2021, no. 1, pp. 1-12, 2021.
[CrossRef] [Google Scholar] [Publisher Link]
[8] Mehshan Ahmed Khan et al., “Gastrointestinal Diseases Segmentation and Classification Based on Duo-Deep Architectures,” Pattern Recognition Letters, vol. 131, pp. 193-204, 2020.
[CrossRef] [Google Scholar] [Publisher Link]
[9] Muhammad Sharif et al., “Deep CNN and Geometric Features-Based Gastrointestinal Tract Diseases Detection and Classification from Wireless Capsule Endoscopy Images,” Journal of Experimental and Theoretical Artificial Intelligence, vol. 33, no. 4, pp. 577-599, 2021.
[CrossRef] [Google Scholar] [Publisher Link]
[10] Subhashree Mohapatra et al., “Wavelet Transform and Deep Convolutional Neural Network-Based Smart Healthcare System for Gastrointestinal Disease Detection,” Interdisciplinary Sciences: Computational Life Sciences, vol. 13, pp. 212-228, 2021.
[CrossRef] [Google Scholar] [Publisher Link]
[11] Muhammad Attique Khan et al., “StomachNet: Optimal Deep Learning Features Fusion for Stomach Abnormalities Classification,” IEEE Access, vol. 8, pp. 197969-197981, 2020.
[CrossRef] [Google Scholar] [Publisher Link]
[12] Xinle Wang et al., “Celiac Disease Diagnosis from Videocapsule Endoscopy Images with Residual Learning and Deep Feature Extraction,” Computer Methods and Programs in Biomedicine, vol. 187, 2020.
[CrossRef] [Google Scholar] [Publisher Link]
[13] Şaban Öztürk, and Umut Özkaya, “Residual LSTM Layered CNN for Classification of Gastrointestinal Tract Diseases,” Journal of Biomedical Informatics, vol. 113, pp. 1-10, 2021.
[CrossRef] [Google Scholar] [Publisher Link]
[14] Shuai Wang et al., “Multi-Scale Context-Guided Deep Network for Automated Lesion Segmentation with Endoscopy Images of Gastrointestinal Tract,” IEEE Journal of Biomedical and Health Informatics, vol. 25, no. 2, pp. 514-525, 2020.
[CrossRef] [Google Scholar] [Publisher Link]
[15] Dimitris K. Iakovidis et al., “Detecting and Locating Gastrointestinal Anomalies Using Deep Learning and Iterative Cluster Unification,” IEEE Transactions on Medical Imaging, vol. 37, no. 10, pp. 2196-2210, 2018.
[CrossRef] [Google Scholar] [Publisher Link]
[16] Shohei Igarashi et al., “Anatomical Classification of Upper Gastrointestinal Organs under Various Image Capture Conditions Using AlexNet,” Computers in Biology and Medicine, vol. 124, 2020.
[CrossRef] [Google Scholar] [Publisher Link]
[17] Muhammad Owais et al., “Artificial Intelligence-Based Classification of Multiple Gastrointestinal Diseases Using Endoscopy Videos for Clinical Diagnosis,” Journal of Clinical Medicine, vol. 8, no. 7, pp. 1-33, 2019.
[CrossRef] [Google Scholar] [Publisher Link]
[18] Chang Seok Bang, Jae Jun Lee, and Gwang Ho Baik, “Computer-Aided Diagnosis of Gastrointestinal Ulcer and Hemorrhage Using Wireless Capsule Endoscopy: Systematic Review and Diagnostic Test Accuracy Meta-analysis,” Journal of Medical Internet Research, vol. 23, no. 12, pp. 1-22, 2021.
[CrossRef] [Google Scholar] [Publisher Link]
[19] Abdul Majid et al., “Classification of Stomach Infections: A Paradigm of Convolutional Neural Network Along with Classical Features Fusion and Selection,” Microscopy Research and Technique, vol. 83, no. 5, pp. 562-576, 2020.
[CrossRef] [Google Scholar] [Publisher Link]
[20] Muhammad Owais et al., “Automated Diagnosis of Various Gastrointestinal Lesions using a Deep Learning–Based Classification and Retrieval Framework with a Large Endoscopic Database: Model Development and Validation,” Journal of Medical Internet Research, vol. 22, no. 11, pp. 1-22, 2020.
[CrossRef] [Google Scholar] [Publisher Link]
[21] Grace Lai-Hung Wong et al., “Machine Learning Model to Predict Recurrent Ulcer Bleeding in Pataients with History of Idiopathic Gastroduodenal Ulcer Bleeding,” APT-Aliment, Pharmacology, Therapeutics, vol. 49, no. 7, pp. 912-918, 2019.
[CrossRef] [Google Scholar] [Publisher Link]
[22] Konstantin Pogorelov et al., “KVASIR: A Multi-Class Image Dataset for Computer Aided Gastrointestinal Disease Detection,” Proceedings of the 8th ACM on Multimedia Systems Conference, Taipei, Taiwan, pp. 164-169, 2017.
[CrossRef] [Google Scholar] [Publisher Link]
[23] Sen Wang et al., “Second Glance Framework (secG): Enhanced Ulcer Detection with Deep Learning on a Large Wireless Capsule Endoscopy Dataset,” Proceedings of the Fourth International Workshop on Pattern Recognition, Nanjing, China, vol. 11198, 2019.
[CrossRef] [Google Scholar] [Publisher Link]
[24] Jessica Escobar et al., “Accurate Deep Learning-Based Gastrointestinal Disease Classification via Transfer Learning Strategy,” 2021 XXIII Symposium on Image, Signal Processing and Artificial Vision (STSIVA), Popayán, Colombia, pp. 1-5, 2021.
[CrossRef] [Google Scholar] [Publisher Link]