A Deep Ensemble Feature Extraction and Classification Framework for Melanoma Identification
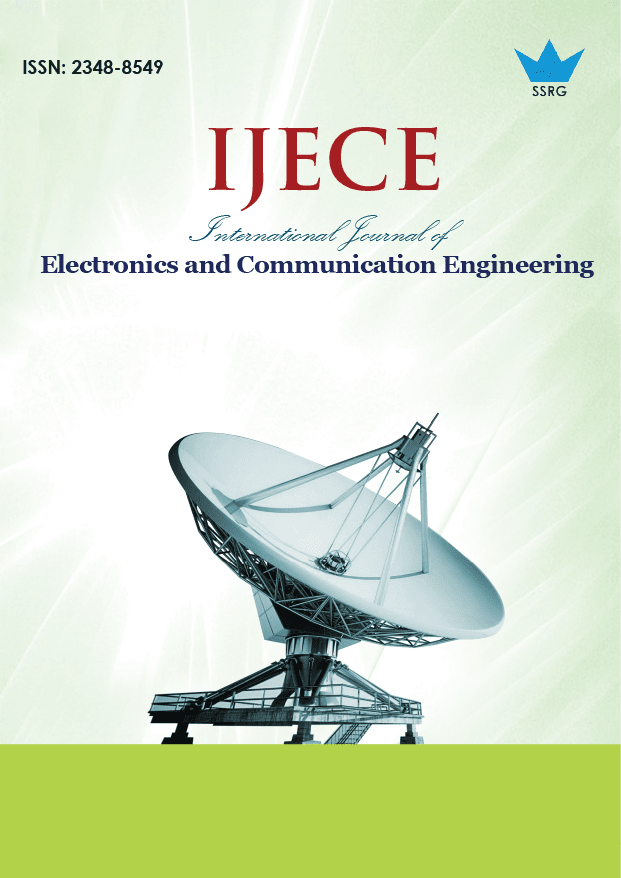
International Journal of Electronics and Communication Engineering |
© 2024 by SSRG - IJECE Journal |
Volume 11 Issue 8 |
Year of Publication : 2024 |
Authors : Soumya Gadag, V. Panduranga Rao Malode |
How to Cite?
Soumya Gadag, V. Panduranga Rao Malode, "A Deep Ensemble Feature Extraction and Classification Framework for Melanoma Identification," SSRG International Journal of Electronics and Communication Engineering, vol. 11, no. 8, pp. 325-334, 2024. Crossref, https://doi.org/10.14445/23488549/IJECE-V11I8P131
Abstract:
Skin cancer is a highly severe manifestation of the disease, with the potential to metastasize to other regions of the body if not identified in its early stages. According to WHO and the American Cancer Society (ACS), it is one of the leading causes of mortality in the worldwide population. Early discovery of skin cancer, however, can aid in a proper diagnosis to lessen the disease's effects on people. Dermoscopy Several methods have been introduced to develop automated Computer-Aided Diagnosis (CAD) systems where machine learning based solutions are widely adopted in this domain. To improve the detection accuracy, researchers have introduced deep learning-based solutions. However, the detection performance is affected by several factors, such as the uneven boundary of skin cancer, pigmentation, and hairs on dermoscopy images. Therefore, a novel approach for feature extraction by using handcrafted shape and texture features is introduced in this work. Moreover, the proposed approach adopts pre-trained deep learning architectures for deep feature extraction. The acquired features are combined and subjected to an ensemble classification technique, which uses decision trees, random forests, and support vector machines. A majority voting categorization is used to arrive at the final choice. The outcome of this approach is validated on publically accessible datasets such as PH2, ISIC 2017, and ISIC 2018.
Keywords:
Classification, Deep Learning, Feature extraction, Machine Learning, Melanoma.
References:
[1] Sunil Kumar et al., “A Chaos Study of Tumor and Effector Cells in Fractional Tumor-Immune Model for Cancer Treatment,” Chaos, Solitons & Fractals, vol. 141, 2020.
[CrossRef] [Google Scholar] [Publisher Link]
[2] Rebecca L. Siegel et al., “Cancer Statistics, 2021,” CA: A Cancer Journal for Clinicians, vol. 71, no. 1, pp. 7-33, 2021.
[CrossRef] [Google Scholar] [Publisher Link]
[3] Faouzi Adjed et al., “Classification of Skin Cancer Images Using Local Binary Pattern and SVM Classifier,” AIP Conference Proceedings, vol. 1787, no. 1, 2016.
[CrossRef] [Google Scholar] [Publisher Link]
[4] Pamela Hallquist Viale, “The American Cancer Society’s Facts & Figures: 2020 Edition,” Journal of the Advanced Practitioner in Oncology, vol. 11, no. 2, pp. 135-136, 2020.
[CrossRef] [Google Scholar] [Publisher Link]
[5] Melina Tziomaka, and Ilias Maglogiannis, “Ensembles of Deep Convolutional Neural Networks for Detecting Melanoma in Dermoscopy Images,” Computational Collective Intelligence: 13th International Conference, Rhodes, Greece, pp. 523-535, 2021.
[CrossRef] [Google Scholar] [Publisher Link]
[6] Ni Zhang et al., “Skin Cancer Diagnosis Based on Optimized Convolutional Neural Network,” Artificial Intelligence in Medicine, vol. 102, 2020.
[CrossRef] [Google Scholar] [Publisher Link]
[7] Ahmed Alsayyah, “Differentiating between Early Melanomas and Melanocytic Nevi: A State-of-the-Art Review,” Pathology - Research and Practice, vol. 249, 2023.
[CrossRef] [Google Scholar] [Publisher Link]
[8] Saqib Ali et al., “State-of-the-Art Challenges and Perspectives in Multi-Organ Cancer Diagnosis via Deep Learning-Based Methods,” Cancers, vol. 13, no. 21, pp. 1-23, 2021.
[CrossRef] [Google Scholar] [Publisher Link]
[9] Anamika Dhillon, and Gyanendra K. Verma, “Convolutional Neural Network: A Review of Models, Methodologies and Applications to Object Detection,” Progress in Artificial Intelligence, vol. 9, pp. 85-112, 2020.
[CrossRef] [Google Scholar] [Publisher Link]
[10] Praveen Banasode, Minal Patil, and Nikhil Ammanagi, “A Melanoma Skin Cancer Detection Using Machine Learning Technique: Support Vector Machine,” IOP Conference Series: Materials Science and Engineering: 1 st International Conference on Frontiers in Engineering Science and Technology, Mangalore, India, vol. 1065, pp. 1-5, 2020.
[CrossRef] [Google Scholar] [Publisher Link]
[11] Adekanmi A. Adegun, and Serestina Viriri, “Deep Learning-Based System for Automatic Melanoma Detection,” IEEE Access, vol. 8, pp. 7160-7172, 2019.
[CrossRef] [Google Scholar] [Publisher Link]
[12] Samira Lafraxo, Mohamed El Ansari, and Said Charfi, “MelaNet: An Effective Deep Learning Framework for Melanoma Detection Using Dermoscopic Images,” Multimedia Tools and Applications, vol. 81, pp. 16021-16045, 2022.
[CrossRef] [Google Scholar] [Publisher Link]
[13] Xinrong Lu, and Y.A. Firoozeh Abolhasani Zadeh, “Deep Learning-Based Classification for Melanoma Detection Using XceptionNet,” Journal of Healthcare Engineering, vol. 2022, no. 1, pp. 1-10, 2022.
[CrossRef] [Google Scholar] [Publisher Link]
[14] Usharani Bhimavarapu, and Gopi Battineni, “Skin Lesion Analysis for Melanoma Detection Using the Novel Deep Learning Model Fuzzy GC-SCNN,” Healthcare, vol. 10, no. 5, pp. 1-14, 2022.
[CrossRef] [Google Scholar] [Publisher Link]
[15] Shubhendu Banerjee et al., “Melanoma Diagnosis Using Deep Learning and Fuzzy Logic,” Diagnostics, vol. 10, no. 8, pp. 1-26, 2020.
[CrossRef] [Google Scholar] [Publisher Link]
[16] R.D. Seeja, and A. Suresh, “Deep Learning Based Skin Lesion Segmentation and Classification of Melanoma Using Support Vector Machine (SVM),” Asian Pacific Journal of Cancer Prevention, vol. 20, no. 5, pp. 1555-1561, 2019.
[CrossRef] [Google Scholar] [Publisher Link]
[17] Amjad Rehman et al., “Microscopic Melanoma Detection and Classification: A Framework of Pixel-Based Fusion and Multilevel Features Reduction,” Microscopy Research and Technique, vol. 83, no. 4, pp. 410-423, 2020.
[CrossRef] [Google Scholar] [Publisher Link]
[18] Priyanti Paul Tumpa, and Md Ahasan Kabir, “An Artificial Neural Network Based Detection and Classification of Melanoma Skin Cancer Using Hybrid Texture Features,” Sensors International, vol. 2, pp. 1-8, 2021.
[CrossRef] [Google Scholar] [Publisher Link]
[19] Kai Hu et al., “Classification of Melanoma Based on Feature Similarity Measurement for Codebook Learning in the Bag-of-Features Model,” Biomedical Signal Processing and Control, vol. 51, pp. 200-209, 2019.
[CrossRef] [Google Scholar] [Publisher Link]
[20] Jason R. Hagerty et al., “Deep Learning and Handcrafted Method Fusion: Higher Diagnostic Accuracy for Melanoma Dermoscopy Images,” IEEE Journal of Biomedical and Health Informatics, vol. 23, no. 4, pp. 1385-1391, 2019.
[CrossRef] [Google Scholar] [Publisher Link]
[21] Sümeyya İlkin et al., “hybSVM: Bacterial Colony Optimization Algorithm Based SVM for Malignant Melanoma Detection,” Engineering Science and Technology, an International Journal, vol. 24, no. 5, pp. 1059-1071, 2021.
[CrossRef] [Google Scholar] [Publisher Link]
[22] Hyunju Lee, and Kiwoon Kwon, “Diagnostic Techniques for Improved Segmentation, Feature Extraction, and Classification of Malignant Melanoma,” Biomedical Engineering Letters, vol. 10, pp. 171-179, 2020.
[CrossRef] [Google Scholar] [Publisher Link]
[23] Khairul Islam et al., “Melanoma Skin Lesions Classification Using Deep Convolutional Neural Network with Transfer Learning,” 2021 1 st International Conference on Artificial Intelligence and Data Analytics, Riyadh, Saudi Arabia, pp. 48-53, 2021.
[CrossRef] [Google Scholar] [Publisher Link]
[24] Amer Sallam et al., “Early Detection of Glaucoma Using Transfer Learning from Pre-Trained CNN Models,” 2021 International Conference of Technology, Science and Administration, Taiz, Yemen, pp. 1-5, 2021.
[CrossRef] [Google Scholar] [Publisher Link]
[25] Muhammad Zain Amin, and Amir Ali, “Performance Evaluation of Supervised Machine Learning Classifiers for Predicting Healthcare Operational Decisions,” Wavy AI Research Foundation, pp. 1-7, 2018.
[Google Scholar]
[26] Siti Salbiah Samsudin et al., “Skin Lesion Classification Using Multi-Resolution Empirical Mode Decomposition and Local Binary Pattern,” Plos One, vol. 17, no. 9, pp. 1-14, 2022.
[CrossRef] [Google Scholar] [Publisher Link]
[27] Alex Krizhevsky, Ilya Sutskever, and Geoffrey E. Hinton, “ImageNet Classification with Deep Convolutional Neural Networks,” Communications of the ACM, vol. 60, no. 6, pp. 84-90, 2012.
[CrossRef] [Google Scholar] [Publisher Link]
[28] Karen Simonyan, and Andrew Zisserman, “Very Deep Convolutional Networks for Large-Scale Image Recognition,” arXiv, pp. 1-14, 2014.
[CrossRef] [Google Scholar] [Publisher Link]
[29] Brett Koonce, ResNet 50, Convolutional Neural Networks with Swift for Tensorflow, Apress, Berkeley, CA, pp. 63-72, 2021.
[CrossRef] [Google Scholar] [Publisher Link]
[30] Noel C.F. Codella et al., “Skin Lesion Analysis Toward Melanoma Detection: A Challenge at the 2017 International Symposium on Biomedical Imaging (ISBI), Hosted by the International Skin Imaging Collaboration,” 2018 IEEE 15th International Symposium on Biomedical Imaging, Washington, DC, USA, pp. 168-172, 2018.
[CrossRef] [Google Scholar] [Publisher Link]
[31] PH² Database, Automatic Computer-Based Diagnosis System for Dermoscopy Images, 2022. [Online]. Available: https://www.fc.up.pt/addi/ph2%20database.html