ANN Optimized DA-STS Under Partial Shading Conditions
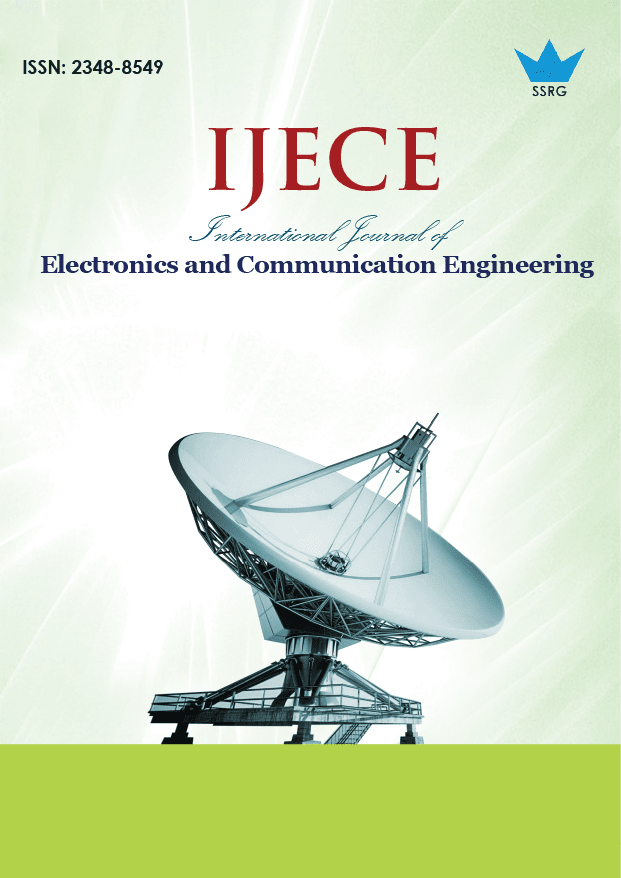
International Journal of Electronics and Communication Engineering |
© 2024 by SSRG - IJECE Journal |
Volume 11 Issue 9 |
Year of Publication : 2024 |
Authors : P. N. Praveen, D. Menaka |
How to Cite?
P. N. Praveen, D. Menaka, "ANN Optimized DA-STS Under Partial Shading Conditions," SSRG International Journal of Electronics and Communication Engineering, vol. 11, no. 9, pp. 47-55, 2024. Crossref, https://doi.org/10.14445/23488549/IJECE-V11I9P105
Abstract:
Solar energy constitutes the primary source of energy within the universe. A variety of methodologies may be employed to effectively harness this energy. The deployment of solar panels is distinguished as a widely adopted and innovative method for the accumulation of this energy. When compared to stationary panels, rotating panels have demonstrated the capacity to produce greater energy outputs under certain circumstances, like partial shading conditions. Solar Tracking Systems (STS) are one of the main methods to track sun movement. The objective of STSs is to optimize energy production by orienting the load, typically solar panels, towards the sun. This is achieved by minimizing the angle of incidence between the incoming sunlight and the Photovoltaic (PV) panel, thereby enhancing the quantity of energy generated. The existing system, Grey Wolf Optimization (GWO) with Maximum Power Point Tracking (MPPT) method, yields a diminished amount of energy, resulting in a significant tracking error. To reduce the tracking error and enhance energy efficiency, the initially proposed method employing Particle Swarm Optimization (PSO) with Artificial Neural Networks (ANNs) has been implemented. This Neural Network (NN) mainly consists of an Adaptive Neuro-Fuzzy Inference System (ANFIS), which includes a workflow from data collection to deployment. This method generates better results than the existing one because of proper training, testing, and data implementation.
Keywords:
ANN, PSO, Solar tracking systems, Solar energy, Partial shading conditions, Photovoltaic, Optimization.
References:
[1] Jinlong Gong, Can Li, and Michael R. Wasielewski, “Advances in Solar Energy Conversion,” Chemical Society Reviews, vol. 48, no. 7, pp. 1862-1864, 2019.
[CrossRef] [Google Scholar] [Publisher Link]
[2] Anshul Awasthi et al., “Review on Sun Tracking Technology in Solar PV System,” Energy Reports, vol. 6, pp. 392-405, 2020.
[CrossRef] [Google Scholar] [Publisher Link]
[3] Yongqiang Zhu, Jiahao Liu, and Xiaohua Yang, “Design and Performance Analysis of a Solar Tracking System with a Novel Single-Axis Tracking Structure to Maximize Energy Collection,” Applied Energy, vol. 264, 2020.
[CrossRef] [Google Scholar] [Publisher Link]
[4] Nadia AL-Rousan, Nor Ashidi Mat Isa, and Mohd Khairunaz Mat Desa, “Efficient Single and Dual Axis Solar Tracking System Controllers Based on Adaptive Neural Fuzzy Inference System,” Journal of King Saud University-Engineering Sciences, vol. 32, no. 7, pp. 459-469, 2020.
[CrossRef] [Google Scholar] [Publisher Link]
[5] Ahmad Haddad et al., “Study of Hybrid Energy System Coupling Fuel Cell, Solar Thermal System and Photovoltaic Cell,” International Journal of Hydrogen Energy, vol. 45, no. 25, pp. 13564-13574, 2020.
[CrossRef] [Google Scholar] [Publisher Link]
[6] A. Khosravi et al., “An Artificial Intelligence Approach for Thermodynamic Modeling of Geothermal Based-Organic Rankine Cycle Equipped with Solar System,” Geothermics, vol. 80, pp. 138-154, 2019.
[CrossRef] [Google Scholar] [Publisher Link]
[7] Kah Yung Yap, Charles R. Sarimuthu, and Joanne Mun-Yee Lim, “Artificial Intelligence Based MPPT Techniques for Solar Power System: A Review,” Journal of Modern Power Systems and Clean Energy, vol. 8, no. 6, pp. 1043-1059, 2020.
[CrossRef] [Google Scholar] [Publisher Link]
[8] Kunal Sandip Garud, Simon Jayaraj, and Moo-Yeon Lee, “A Review on Modeling of Solar Photovoltaic Systems Using Artificial Neural Networks, Fuzzy Logic, Genetic Algorithm and Hybrid Models,” International Journal of Energy Research, vol. 45, no. 1, pp. 6-35, 2021.
[CrossRef] [Google Scholar] [Publisher Link]
[9] Abdul Rahim Pazikadin et al., “Solar Irradiance Measurement Instrumentation and Power Solar Generation Forecasting Based on Artificial Neural Networks (ANN): A Review of Five Years Research Trend,” Science of the Total Environment, vol. 715, 2020.
[CrossRef] [Google Scholar] [Publisher Link]
[10] Omar A. Al-Shahri et al., “Solar Photovoltaic Energy Optimization Methods, Challenges and Issues: A Comprehensive Review,” Journal of Cleaner Production, vol. 284, 2021.
[CrossRef] [Google Scholar] [Publisher Link]
[11] Toufik Arrif et al., “Optimisation of Heliostat Field Layout for Solar Power Tower Systems using Iterative Artificial Bee Colony Algorithm: A Review And Case Study,” International Journal of Ambient Energy, vol. 42, no. 1, pp. 65-80, 2021.
[CrossRef] [Google Scholar] [Publisher Link]
[12] G. Satheesh Krishnan et al., “MPPT in PV Systems Using Ant Colony Optimisation with Dwindling Population,” IET Renewable Power Generation, vol. 14, no. 7, pp. 1105-1112, 2020.
[CrossRef] [Google Scholar] [Publisher Link]
[13] Ahmed G. Abo-Khalil et al., “Maximum Power Point Tracking of PV Systems Under Partial Shading Conditions Based on Opposition-Based Learning Firefly Algorithm,” Sustainability, vol. 13, no. 5, pp. 1-18, 2021.
[CrossRef] [Google Scholar] [Publisher Link]
[14] Jose A. Carballo et al., “New Approach for Solar Tracking Systems Based on Computer Vision, Low-Cost Hardware and Deep Learning,” Renewable Energy, vol. 133, pp. 1158-1166, 2019.
[CrossRef] [Google Scholar] [Publisher Link]
[15] Chaowanan Jamroen et al., “A Low-Cost Dual-Axis Solar Tracking System Based on Digital Logic Design: Design and Implementation,” Sustainable Energy Technologies and Assessments, vol. 37, 2020.
[CrossRef] [Google Scholar] [Publisher Link]
[16] Muhammad E.H. Chowdhury et al., “A Low-Cost Closed-Loop Solar Tracking System Based on the Sun Position Algorithm,” Journal of Sensors, vol. 2019, no. 1, pp. 1-11, 2019.
[CrossRef] [Google Scholar] [Publisher Link]
[17] Jose A. Carballo et al., “New Approach for Solar Tracking Systems Based on Computer Vision, Low-Cost Hardware and Deep Learning,” Renewable Energy, vol. 133, pp. 1158-1166, 2019.
[CrossRef] [Google Scholar] [Publisher Link]
[18] Hyuna Kang et al., “Techno-Economic Performance Analysis of the Smart Solar Photovoltaic Blinds Considering the Photovoltaic Panel Type and the Solar Tracking Method,” Energy and Buildings, vol. 193, pp. 1-14, 2019.
[CrossRef] [Google Scholar] [Publisher Link]
[19] A.B. Gurulakshmi et al., “Scheduled Line of Symmetry Solar Tracker with MPT and IoT,” IoT Based Control Networks and Intelligent Systems, pp. 265-274, 2023.
[CrossRef] [Google Scholar] [Publisher Link]
[20] Subhash C. Singh et al., “Solar-Trackable Super-Wicking Black Metal Panel for Photothermal Water Sanitation,” Nature Sustainability, vol. 3, no. 11, pp. 938-946, 2020.
[CrossRef] [Google Scholar] [Publisher Link]
[21] Amit Kumer Podder, Naruttam Kumar Roy, and Hemanshu Roy Pota, “MPPT Methods for Solar PV Systems: A Critical Review Based on Tracking Nature,” IET Renewable Power Generation, vol. 13, no. 10, pp. 1615-1632, 2019.
[CrossRef] [Google Scholar] [Publisher Link]
[22] Jane Oktavia Kamadinata, Tan Lit Ken, and Tohru Suwa, “Sky Image-Based Solar Irradiance Prediction Methodologies Using Artificial Neural Networks,” Renewable Energy, vol. 134, pp. 837-845, 2019.
[CrossRef] [Google Scholar] [Publisher Link]
[23] Ankur Kumar Gupta, Yogesh K. Chauhan, and Tanmoy Maity, “A New Gamma Scaling Maximum Power Point Tracking Method for Solar Photovoltaic Panel Feeding Energy Storage System,” IETE Journal of Research, vol. 67, no. 1, pp. 15-35, 2021.
[CrossRef] [Google Scholar] [Publisher Link]
[24] Hwa-Dong Liu et al., “A Novel SLOPDM Solar Maximum Power Point Tracking Control Strategy for the Solar Photovoltaic Power System,” Processes, vol. 10, no. 8, 2022.
[CrossRef] [Google Scholar] [Publisher Link]