Advanced Traffic Forecasting Integrating Temporal and Spatial Dependencies Using Hybrid Deep Learning Models
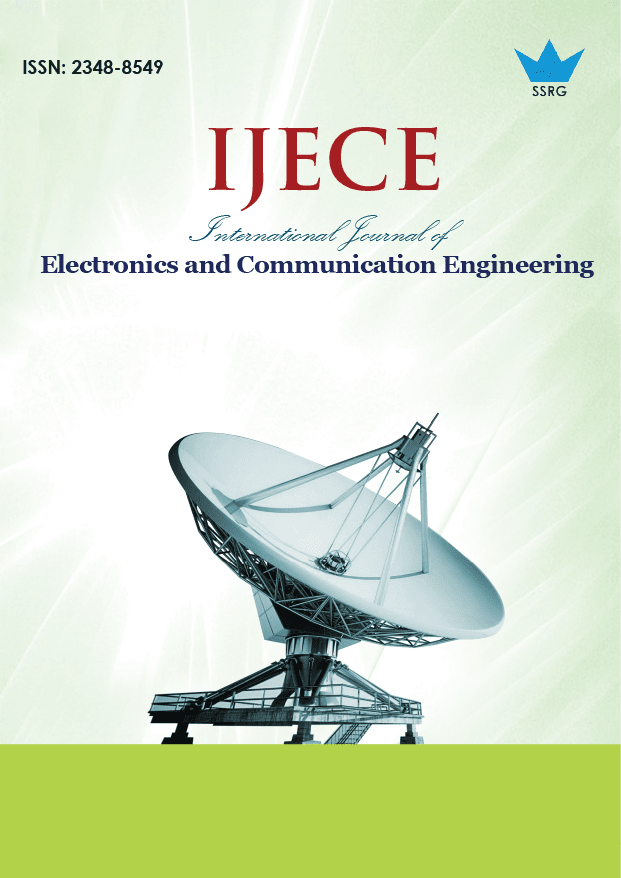
International Journal of Electronics and Communication Engineering |
© 2024 by SSRG - IJECE Journal |
Volume 11 Issue 9 |
Year of Publication : 2024 |
Authors : Abdirahman Ali Muse, Ali Musse Hassan |
How to Cite?
Abdirahman Ali Muse, Ali Musse Hassan, "Advanced Traffic Forecasting Integrating Temporal and Spatial Dependencies Using Hybrid Deep Learning Models," SSRG International Journal of Electronics and Communication Engineering, vol. 11, no. 9, pp. 64-76, 2024. Crossref, https://doi.org/10.14445/23488549/IJECE-V11I9P107
Abstract:
Accurate traffic forecasting is important for refining traffic management and planning to avoid congestion on the roads and enhance road safety. Traditional models often misfire on complex, nonlinear patterns in traffic. In this study, the hybrid LSTM-CNN model proposed in this paper would overcome the limitations by modeling both temporal and spatial dependencies, thus ensuring better accuracy and reliability in prediction. The study portrays the hybrid model of LSTM-CNN to overcome all such limitations and focus on capturing the temporal and spatial dependencies pertaining to traffic features. The paper uses a rich dataset comprising variables like volume, speed, and occupancy from highway sensors. It gives a model using LSTM layers in combination with CNN to perform better in prediction. Further refinements were done in training using hyperparameters; the evaluation of performance was executed on R², MAPE, and RMSE. The hybrid model gave the lowest validation loss of 0.05 and the lowest test MAPE of 0.08, which is better than the conventional models. More precisely, from the LSTM model, R² score = 0.081, MAPE = 3.66%, and RMSE = 0.248; from the CNN model, R² score = 0.029, MAPE = 4.07%, and RMSE = 0.255. R² of 0.063, MAPE of 3.84%, and RMSE of 0.250 were found for the hybrid model, with LSTM before CNN. In reversed order—that is, the hybrid model of CNN first—the values are as follows: the model recorded an R² of 0.054, a MAPE of 4.15%, and an RMSE of 0.252.
Keywords:
Traffic forecasting, Hybrid LSTM-CNN model, Temporal and spatial dependencies, Prediction accuracy, Integration of deep learning.
References:
[1] Sai Usha Goparaju et al., “Optimization and Performance Evaluation of Hybrid Deep Learning Models for Traffic Flow Prediction,” 2023 IEEE 97th Vehicular Technology Conference, Florence, Italy, pp. 1-7, 2023.
[CrossRef] [Google Scholar] [Publisher Link]
[2] Shubhashish Goswami, and Abhimanyu Kumar, “Traffic Prediction Using Auxiliary Information Based on Mlbsae-A Hybrid Deep Learning Framework,” 2023 14th International Conference on Computing Communication and Networking Technologies, Delhi, India, pp. 1-5, 2023.
[CrossRef] [Google Scholar] [Publisher Link]
[3] Shengdong Du et al., “Traffic Flow Forecasting Based on Hybrid Deep Learning Framework,” 2017 12th International Conference on Intelligent Systems and Knowledge Engineering, Nanjing, China, pp. 1-6, 2017.
[CrossRef] [Google Scholar] [Publisher Link]
[4] Qu Zhaowei et al., “Short-Term Traffic Flow Forecasting Method with M-B-LSTM Hybrid Network,” IEEE Transactions on Intelligent Transportation Systems, vol. 23, no. 1, pp. 225-235, 2022.
[CrossRef] [Google Scholar] [Publisher Link]
[5] Akshada Chavan et al., “Traffic Congestions Prediction Using Machine Learning and Deep Learning Techniques,” 2023 4th International Conference on Computation, Automation and Knowledge Management, Dubai, United Arab Emirates, pp. 1-6, 2023.
[CrossRef] [Google Scholar] [Publisher Link]
[6] Haifeng Zheng et al., “A Hybrid Deep Learning Model With Attention-Based Conv-LSTM Networks for Short-Term Traffic Flow Prediction,” IEEE Transactions on Intelligent Transportation Systems, vol. 22, no. 11, pp. 6910-6920, 2021.
[CrossRef] [Google Scholar] [Publisher Link]
[7] Zeyang Cheng et al., “Short-Term Traffic Flow Prediction: An Integrated Method of Econometrics and Hybrid Deep Learning,” IEEE Transactions on Intelligent Transportation Systems, vol. 23, no. 6, pp. 5231-5244, 2022.
[CrossRef] [Google Scholar] [Publisher Link]
[8] C. Kaushik et al., “A Novel Approach for Real Time Traffic Prediction Using Deep Learning in VANET,” 2023 14th International Conference on Computing Communication and Networking Technologies, Delhi, India, pp. 1-6, 2023.
[CrossRef] [Google Scholar] [Publisher Link]
[9] Anupama Prasanth et al., “A Hybrid Approach for Web Traffic Prediction Using Deep Learning Algorithms,” 2022 9th International Conference on Electrical and Electronics Engineering, Alanya, Turkey, pp. 383-386, 2022.
[CrossRef] [Google Scholar] [Publisher Link]
[10] Richard Etengu et al., “Deep Learning-Assisted Traffic Prediction in Hybrid SDN/OSPF Backbone Networks,” NOMS 2022-2022 IEEE/IFIP Network Operations and Management Symposium, Budapest, Hungary, pp. 1-6, 2022.
[CrossRef] [Google Scholar] [Publisher Link]
[11] Farnaz Sarhangian, Rasha Kashef, and Muhammad Jaseemuddin, “Efficient Traffic Classification Using Hybrid Deep Learning,” 2021 IEEE International Systems Conference, Vancouver, BC, Canada, pp. 1-8, 2021.
[CrossRef] [Google Scholar] [Publisher Link]
[12] L.M.I. Leo Joseph et al., “A Novel Hybrid Deep Learning Algorithm for Smart City Traffic Congestion Predictions,” 2021 6th International Conference on Signal Processing, Computing and Control, Solan, India, pp. 561-565, 2021.
[CrossRef] [Google Scholar] [Publisher Link]
[13] Smita Mahajan, R. Harikrishnan, and Ketan Kotecha, “Prediction of Network Traffic in Wireless Mesh Networks Using Hybrid Deep Learning Model,” IEEE Access, vol. 10, pp. 7003-7015, 2022.
[CrossRef] [Google Scholar] [Publisher Link]
[14] Xianlei Fu et al., “A Hybrid Deep Learning Approach for Real-Time Estimation of Passenger Traffic Flow in Urban Railway Systems,” Buildings, vol. 13, no. 6, pp. 1-17, 2023.
[CrossRef] [Google Scholar] [Publisher Link]
[15] Gouse Pasha Mohammed et al., “Autonomous Short-Term Traffic Flow Prediction Using Pelican Optimization with Hybrid Deep Belief Network in Smart Cities,” Applied Sciences, vol. 12, no. 21, pp. 1-16, 2022.
[CrossRef] [Google Scholar] [Publisher Link]
[16] Yan Li et al., “Deep Learning-Powered Vessel Traffic Flow Prediction with Spatial-Temporal Attributes and Similarity Grouping,” Engineering Applications of Artificial Intelligence, vol. 126, no. B, pp. 1-25, 2023.
[CrossRef] [Google Scholar] [Publisher Link]
[17] Xianhui Zong et al., “An Intelligent Deep Learning Framework for Traffic Flow Imputation and Short-term Prediction Based on Dynamic Features,” Knowledge-Based Systems, vol. 300, 2024.
[CrossRef] [Google Scholar] [Publisher Link]
[18] Shtwai Alsubai, Ashit Kumar Dutta, and Abdul Rahaman Wahab Sait, “Hybrid Deep Learning-Based Traffic Congestion Control in IoT Environment Using Enhanced Arithmetic Optimization Technique,” Alexandria Engineering Journal, vol. 105, pp. 331-340, 2024.
[CrossRef] [Google Scholar] [Publisher Link]
[19] Pietro Casabianca et al., “Vehicle Destination Prediction Using Bidirectional LSTM with Attention Mechanism,” Sensors, vol. 21, no. 24, pp. 1-15, 2021.
[CrossRef] [Google Scholar] [Publisher Link]
[20] Manuel Méndez, Mercedes G. Merayo, and Manuel Núñez, “Long-Term Traffic Flow Forecasting Using a Hybrid CNN-BiLSTM Model,” Engineering Applications of Artificial Intelligence, vol. 121, pp. 1-15, 2023.
[CrossRef] [Google Scholar] [Publisher Link]
[21] Bowen Wang, Traffic Flow Forecast Data, Mendeley Data, 2022.
[CrossRef] [Publisher Link]
[22] Fouzi Harrou et al., “Enhancing Road Traffic Flow Prediction with Improved Deep Learning Using Wavelet Transforms,” Results in Engineering, vol. 23, pp. 1-14, 2024.
[CrossRef] [Google Scholar] [Publisher Link]
[23] Sachin Ranjan et al., “Large-Scale Road Network Traffic Congestion Prediction Based on Recurrent High-Resolution Network,” Applied Sciences, vol. 13, no. 9, pp. 1-16, 2023.
[CrossRef] [Google Scholar] [Publisher Link]
[24] Yunuo Zhang et al., “Adaboosting Graph Attention Recurrent Network: A Deep Learning Framework for Traffic Speed Forecasting in Dynamic Transportation Networks with Spatial-Temporal Dependencies,” Engineering Applications of Artificial Intelligence, vol. 127, no. A, 2024.
[CrossRef] [Google Scholar] [Publisher Link]
[25] Fouzi Harrou et al., “Forecasting of Bicycle and Pedestrian Traffic Using Flexible and Efficient Hybrid Deep Learning Approach,” Applied Sciences, vol. 12, no. 9, pp. 1-21, 2022.
[CrossRef] [Google Scholar] [Publisher Link]
[26] Mohammad Tamim Kashifi, Mohammed Al-Turki, and Abdul Wakil Sharify, “Deep Hybrid Learning Framework for Spatiotemporal Crash Prediction Using Big Traffic Data,” International Journal of Transportation Science and Technology, vol. 12, no. 3, pp. 793-808, 2023.
[CrossRef] [Google Scholar] [Publisher Link]