Comparison of Supervised Classifiers and Strategies for Dealing with Missing Data for Chronic Kidney Disease Diagnosis
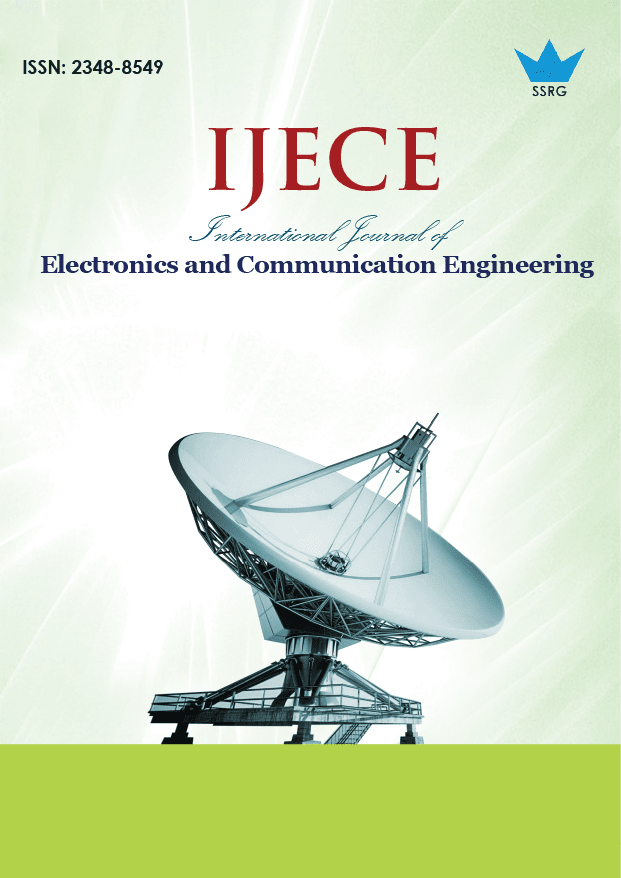
International Journal of Electronics and Communication Engineering |
© 2024 by SSRG - IJECE Journal |
Volume 11 Issue 9 |
Year of Publication : 2024 |
Authors : A. Swathi, Golda Dilip, A Vani Vathsala |
How to Cite?
A. Swathi, Golda Dilip, A Vani Vathsala, "Comparison of Supervised Classifiers and Strategies for Dealing with Missing Data for Chronic Kidney Disease Diagnosis," SSRG International Journal of Electronics and Communication Engineering, vol. 11, no. 9, pp. 99-107, 2024. Crossref, https://doi.org/10.14445/23488549/IJECE-V11I9P110
Abstract:
Chronic Kidney Disease (CKD) has been identified as a major global health issue since it is often asymptomatic and associated with diseases such as diabetes and hypertension. This research focuses on the need to develop better prediction models to ensure early detection and management. The goal of this study is to improve the accuracy of CKD prediction using a set of supervised machine learning methods combined with efficient missing data imputation strategies based on a dataset containing longitudinal clinical data of 10,000 patients. Thus, based on the Random Forest, Decision Tree, and Support Vector Machine algorithms, a comparative analysis is applied, which considers the usage of efficient data imputation techniques for handling missing clinical data. The experimental assessment shows that Random Forest is the best model for predicting customer churn with an average accuracy of 85% as compared to Decision Tree (79%) and Support Vector Machine (81%). Furthermore, the study also emphasizes the importance of feature selection and ensemble learning techniques for enhancing prediction reliability. These outcomes thus highlight the applicability of sophisticated machine learning algorithms in identifying and distinguishing patients in the initial stages of CKD and estimating their risk of developing further complications to allow for timely medical management. Future implications include the addition of genetic information and biomarkers of the patient to increase the level of prediction. Thus, the results of this research will help to improve the overall clinical decision-making and outcomes for CKD patients worldwide through the provision of individualized treatment regimens and more efficient utilization of healthcare resources
Keywords:
Chronic kidney disease, Machine learning, Predictive modeling, Data imputation, Healthcare, Personalized medicine.
References:
[1] DM Vivekanand Jha et al., “Chronic Kidney Disease: Global Dimension and Perspectives,” The Lancet, vol. 382, no. 9888, pp. 260-272, 2013.
[Google Scholar] [Publisher Link]
[2] Polishetty Pranay, Mandadi Rahul Reddy, and K. Venkatesh Sharma, “Advancing Chronic Kidney Disease Diagnosis: A Predictive Model Using Random Forest Classifier,” International Journal of Computer Engineering in Research Trends, vol. 10, no. 10, pp. 1- 7, 2023.
[CrossRef] [Google Scholar] [Publisher Link]
[3] Andrew S. Levey, and Josef Coresh, “Chronic Kidney Disease,” The Lancet, vol. 379, no. 9811, pp. 165-180, 2012.
[Google Scholar] [Publisher Link]
[4] Gunasekaran Manogaran and Daphne Lopez, “A survey of big data architectures and machine learning algorithms in healthcare,” International Journal of Biomedical Engineering and Technology, vol. 25, no. 2-4, pp. 182-211, 2017.
[CrossRef] [Google Scholar] [Publisher Link]
[5] Vinuthna Papana, Devireddy Sritha Reddy, and Kistipati Priyatham Reddy, “Transformative Approaches in Integrating Data Science for Disease Outbreak Prediction: A Comprehensive Survey in Epidemiology,” International Journal of Computer Engineering in Research Trends, vol. 10, no. 11, pp. 55-65, 2023.
[CrossRef] [Publisher Link]
[6] Claus Garbe, Isabelle Hoorens, and Lieve Brochez, “SkinNet360: A Comprehensive 3D Imaging and Analysis System for Skin Cancer Detection Using Deep Learning,” Frontiers in Collaborative Research, vol. 1, no. 3, pp. 1-10, 2023.
[Google Scholar] [Publisher Link]
[7] AHelmie Arif Wibawa, Indra Malik, and Nurdin Bahtiar, “Evaluation of Kernel-Based Extreme Learning Machine Performance for Prediction of Chronic Kidney Disease,” 2nd International Conference on Informatics and Computational Sciences (ICICoS), Semarang, Indonesia, pp. 1-4, 2018.
[CrossRef] [Google Scholar] [Publisher Link]
[8] Robbi Rahim, and Abdul Wahid, “Advancements in Plant Disease Detection: Integrating Machine Learning, Image Processing, and Precision Agriculture,” International Journal of Computer Engineering in Research Trends, vol. 10, no. 8, pp. 19-25, 2023.
[CrossRef] [Google Scholar] [Publisher Link]
[9] Guneet Kaur; Ajay Sharma, “Predict chronic kidney disease using data mining algorithms in hadoop," 2017 International Conference on Inventive Computing and Informatics (ICICI), Coimbatore, India, pp. 973-979, 2017.
[CrossRef] [Google Scholar] [Publisher Link]
[10] Njoud Abdullah Almansour et al., “Neural Network and Support Vector Machine for the Prediction of Chronic Kidney Disease: A Comparative Study,” Computers in Biology and Medicine, vol. 109, pp. 101-111, 2019.
[CrossRef] [Google Scholar] [Publisher Link]
[11] Abdulhamit Subasi, Emina Alickovic, and Jasmin Kevric, “Diagnosis of Chronic Kidney Disease by Using Random Forest,” CMBEBIH 2017: Proceedings of the International Conference on Medical and Biological Engineering 2017, Sarajevo, B&H, vol. 62, pp. 589-594, 2017.
[CrossRef] [Google Scholar] [Publisher Link]
[12] Hamida Ilyas et al., “Chronic Kidney Disease Diagnosis Using Decision Tree Algorithms,” BMC Nephrology, vol. 22, no. 1, pp. 1- 11, 2021.
[CrossRef] [Google Scholar] [Publisher Link]
[13] Tsehay Admassu Assegie, “Automated Chronic Kidney Disease Detection Model with Knearest Neighbor,” International Journal of Computer and Information Technology, vol. 10, no. 3, pp. 111-115, 2021.
[CrossRef] [Google Scholar] [Publisher Link]
[14] Merve Dogruyol Basar, and Aydin Akan, “Detection of Chronic Kidney Disease by Using Ensemble Classifiers,” 2017 10th International Conference on Electrical and Electronics Engineering, Bursa, Turkey, pp. 544-547, 2017.
[Google Scholar] [Publisher Link]
[15] A Victor Ikechukwu et al., “Diagnosis of Chronic Kidney Disease Using Naïve Bayes Algorithm Supported by Stage Prediction Using eGFR,” International Journal of Computer Engineering in Research Trends, vol. 7, no. 10, pp. 6-12, 2020.
[Google Scholar] [Publisher Link]
[16] Ravi Kumar Tirandasu, Antonio Trujillo Narcía, and Georgina Córdova Ballona, “Spatial-Temporal Disease Dynamics in Banana Crops: A Predictive Analytics Approach for Sustainable Production,” Synthesis a Multidisciplinary Research, vol. 1, no. 2, pp. 1- 11, 2023.
[Google Scholar] [Publisher Link]
[17] Mansoor Iqbal, Chronic Kidney Disease Dataset, Kaggle, 2017. [Online]. Available: https://www.kaggle.com/datasets/mansoordaku/ckdisease/data
[18] P. SumanPrakash et al., “Learning-Driven Continuous Diagnostics and Mitigation Program for Secure Edge Management through Zero-Trust Architecture,” Computer Communications, vol. 220, pp. 94-107, 2024.
[CrossRef] [Google Scholar] [Publisher Link]
[19] Ebrahime Mohammed Senan et al., “Diagnosis of Chronic Kidney Disease Using Effective Classification Algorithms and Recursive Feature Elimination Techniques,” Journal of Healthcare Engineering, vol. 2021, no. 1, pp. 1-10, 2021.
[CrossRef] [Google Scholar] [Publisher Link]