Oropharyngeal Cancer Prediction and Optimization Using Improved VGG16 with Grey Wolf Optimizer
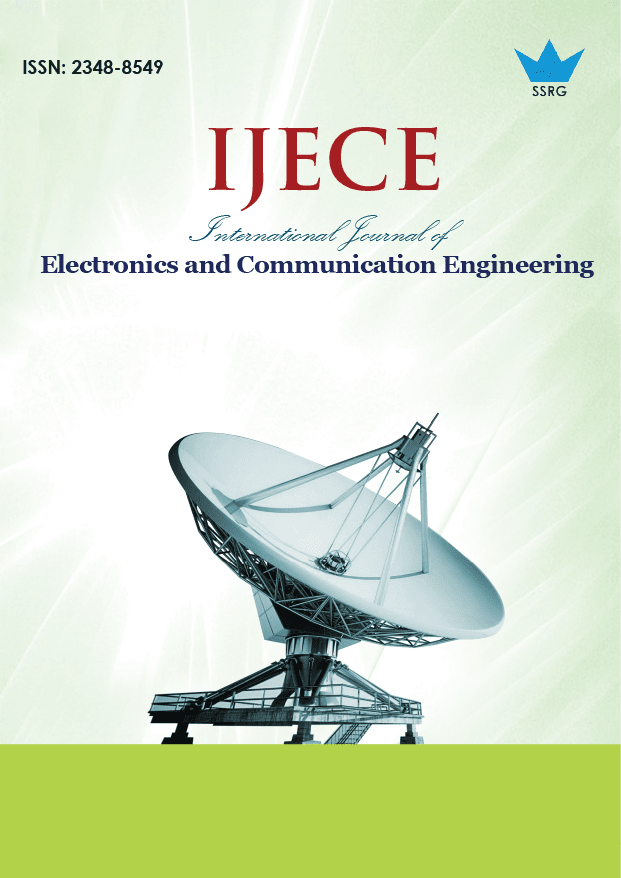
International Journal of Electronics and Communication Engineering |
© 2024 by SSRG - IJECE Journal |
Volume 11 Issue 9 |
Year of Publication : 2024 |
Authors : R. Kumar, S. Pazhanirajan |
How to Cite?
R. Kumar, S. Pazhanirajan, "Oropharyngeal Cancer Prediction and Optimization Using Improved VGG16 with Grey Wolf Optimizer," SSRG International Journal of Electronics and Communication Engineering, vol. 11, no. 9, pp. 108-119, 2024. Crossref, https://doi.org/10.14445/23488549/IJECE-V11I9P111
Abstract:
Oropharyngeal cancer, a subtype of head and neck cancer, presents significant challenges in early detection and treatment. In this paper, we propose a novel approach for predicting and optimizing oropharyngeal cancer using an improved VGG16 architecture with the Grey Wolf Optimizer (GWO) algorithm. The utilization of deep learning techniques has shown promise in medical image analysis, particularly in cancer detection, due to their ability to extract complex features from imaging data. The VGG16 architecture, known for its deep convolutional layers, is enhanced with additional layers and regularization techniques to improve its predictive performance. We utilize a comprehensive dataset comprising high-resolution medical images of oropharyngeal tissues for training and evaluation purposes. The dataset is preprocessed to enhance image quality and standardize features across samples. Subsequently, the proposed model is trained using this dataset, with the objective of accurately classifying images as either cancerous or non-cancerous. Experimental results demonstrate the effectiveness of the proposed approach in predicting oropharyngeal cancer with high accuracy and efficiency. Compared to traditional methods, the improved VGG16 architecture combined with the Grey Wolf Optimizer achieves superior performance in terms of both prediction accuracy and convergence speed. Moreover, the model exhibits robustness against variations in imaging conditions and patient demographics.
Keywords:
Deep learning techniques, Early detection, Grey wolf optimizer, Oropharyngeal cancer, VGG16 architecture.
References:
[1] Masahiro Adachi et al., “Extracting Interpretable Features for Pathologists Using Weakly Supervised Learning to Predict P16 Expression in Oropharyngeal Cancer,” Scientific Reports, vol. 14, no. 1, pp. 1-12, 2024.
[CrossRef] [Google Scholar] [Publisher Link]
[2] Rasheed Omobolaji Alabi et al., “An Interpretable Machine Learning Prognostic System for Risk Stratification in Oropharyngeal Cancer,” International Journal of Medical Informatics, vol. 168, pp. 1-12, 2022.
[CrossRef] [Google Scholar] [Publisher Link]
[3] P. Ashok Babu et al., “An Explainable Deep Learning Approach for Oral Cancer Detection,” Journal of Electrical Engineering & Technology, vol. 19, no. 3, pp. 1837-1848, 2024.
[CrossRef] [Google Scholar] [Publisher Link]
[4] Jason W. Chan et al., “Artificial Intelligence-Guided Prediction of Dental Doses Before Planning of Radiation Therapy for Oropharyngeal Cancer: Technical Development and Initial Feasibility of Implementation,” Advances in Radiation Oncology, vol. 7, no. 2, pp. 1-8, 2022.
[CrossRef] [Google Scholar] [Publisher Link]
[5] Nai-Ming Cheng et al., “Deep Learning for Fully Automated Prediction of Overall Survival in Patients with Oropharyngeal Cancer Using FDG-PET Imaging,” Clinical Cancer Research, vol. 27, no. 14, pp. 3948-3959, 2021.
[CrossRef] [Google Scholar] [Publisher Link]
[6] Alessia De Biase et al., “Deep Learning-Based Outcome Prediction Using PET/CT and Automatically Predicted Probability Maps of Primary Tumor in Patients with Oropharyngeal Cancer,” Computer Methods and Programs in Biomedicine, vol. 244, pp. 1-10, 2024.
[CrossRef] [Google Scholar] [Publisher Link]
[7] Alessia De Biase et al., “Deep Learning Aided Oropharyngeal Cancer Segmentation with Adaptive Thresholding for Predicted Tumor Probability in FDG PET and CT Images,” Physics in Medicine & Biology, vol. 68, no. 5, pp. 1-16, 2023.
[CrossRef] [Google Scholar] [Publisher Link]
[8] Carole Fakhry et al., “Development and Validation of Nomograms Predictive of Overall and Progression-Free Survival in Patients with Oropharyngeal Cancer,” Journal of Clinical Oncology, vol. 35, no. 36, pp. 4057-4065, 2017.
[CrossRef] [Google Scholar] [Publisher Link]
[9] Michael R. Folkert et al., “Predictive Modeling of Outcomes Following Definitive Chemoradiotherapy for Oropharyngeal Cancer Based on FDG-PET Image Characteristics,” Physics in Medicine & Biology, vol. 62, no. 13, pp. 1-17, 2017.
[CrossRef] [Google Scholar] [Publisher Link]
[10] Noriyuki Fujima et al., “Prediction of the Local Treatment Outcome in Patients with Oropharyngeal Squamous Cell Carcinoma Using Deep Learning Analysis of Pretreatment FDG-PET Images,” BMC Cancer, vol. 21, pp. 1-13, 2021.
[CrossRef] [Google Scholar] [Publisher Link]
[11] Nadimul Hoda et al., “Artificial Intelligence Based Assessment and Application of Imaging Techniques for Early Diagnosis in Oral Cancers,” International Surgery Journal, vol. 11, no. 2, pp. 318-322, 2024.
[CrossRef] [Google Scholar] [Publisher Link]
[12] Daniel M. Lang et al., “Deep Learning Based HPV Status Prediction for Oropharyngeal Cancer Patients,” Cancers, vol. 13, no. 4, pp. 1-11, 2021.
[CrossRef] [Google Scholar] [Publisher Link]
[13] Ryan J. Langdon et al., “Epigenetic Prediction of Complex Traits and Mortality in a Cohort of Individuals with Oropharyngeal Cancer,” Clinical Epigenetics, vol. 12, pp. 1-14, 2020.
[CrossRef] [Google Scholar] [Publisher Link]
[14] Eman Shawky Mira et al., “Early Diagnosis of Oral Cancer Using Image Processing and Artificial Intelligence,” Fusion: Practice and Applications, vol. 14, no. 1, pp. 293-308, 2024.
[CrossRef] [Google Scholar] [Publisher Link]
[15] Shriya Sawant et al., “Oral Microbial Signatures of Tobacco Chewers and Oral Cancer Patients in India,” Pathogens, vol. 12, no. 1, pp. 1-12, 2023.
[CrossRef] [Google Scholar] [Publisher Link]
[16] Chunhao Wang et al., “Dose-Distribution-Driven PET Image-Based Outcome Prediction (DDD-PIOP): A Deep Learning Study for Oropharyngeal Cancer IMRT Application,” Frontiers in Oncology, vol. 10, pp. 1-10, 2020.
[CrossRef] [Google Scholar] [Publisher Link]
[17] Jia Wu et al., “Tumor Subregion Evolution-Based Imaging Features to Assess Early Response and Predict Prognosis in Oropharyngeal Cancer,” Journal of Nuclear Medicine, vol. 61, no. 3, pp. 327-336, 2020.
[CrossRef] [Google Scholar] [Publisher Link]
[18] Xiaoshuai Xu et al., “Deep Learning Assisted Contrast-Enhanced CT–Based Diagnosis of Cervical Lymph Node Metastasis of Oral Cancer: A Retrospective Study of 1466 Cases,” European Radiology, vol. 33, no. 6, pp. 4303-4312, 2023.
[CrossRef] [Google Scholar] [Publisher Link]
[19] Abdelbaki Souid, Nizar Sakli, and Hedi Sakli, “Classification and Predictions of Lung Diseases from Chest X-Rays Using Mobilenet V2,” Applied Sciences, vol. 11, no. 6, pp. 1-16, 2021.
[CrossRef] [Google Scholar] [Publisher Link]
[20] N. Nanda Prakash et al., “A DenseNet CNN-Based Liver Lesion Prediction and Classification for Future Medical Diagnosis,” Scientific African, vol. 20, pp. 1-16, 2023.
[CrossRef] [Google Scholar] [Publisher Link]
[21] Sara Saad Abd Al-jabar, Nasseer Moyasser Basheer, and Omar Ibrahim Alsaif, “Alzheimer’s Diseases Classification Using Yolov2 Object Detection Technique,” International Journal of Reconfigurable and Embedded Systems, vol. 11, no. 3, pp. 240-248, 2022.
[CrossRef] [Google Scholar] [Publisher Link]
[22] V. Alamelu, and S. Thilagamani, “Lion Based Butterfly Optimization with Improved YOLO-V4 for Heart Disease Prediction Using IoMT,” Information Technology and Control, vol. 51, no. 4, pp. 692-703, 2022.
[CrossRef] [Google Scholar] [Publisher Link]
[23] Shagun Sharma et al., “A Deep Learning Based Convolutional Neural Network Model with VGG16 Feature Extractor for the Detection of Alzheimer Disease Using MRI Scans,” Measurement:Sensors, vol. 24, pp. 1-8, 2022.
[CrossRef] [Google Scholar] [Publisher Link]
[24] Dataset-Benchmark. [Online]. Available: https://www.kaggle.com/general/248718