Transforming E-commerce with a Novel Multifaceted Data-Decision Framework
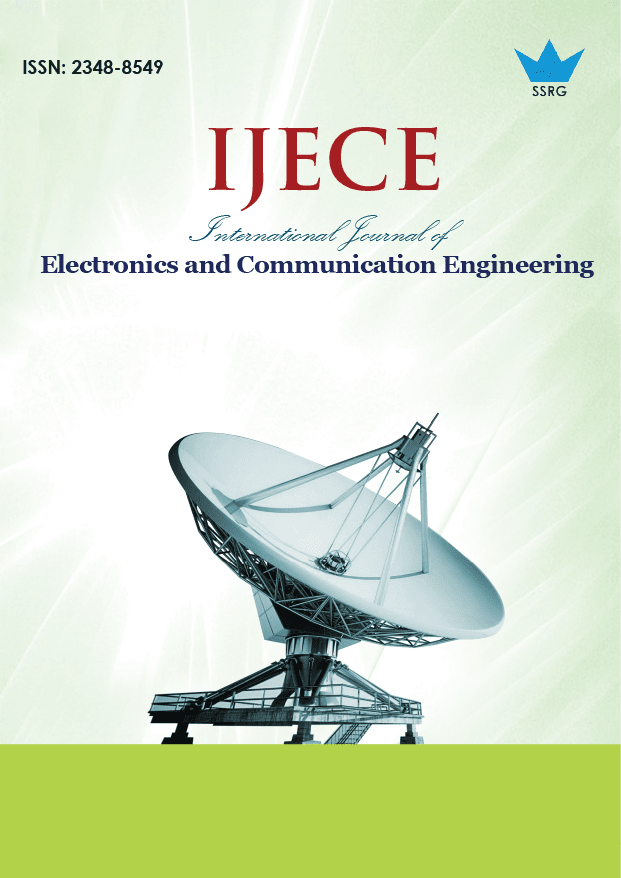
International Journal of Electronics and Communication Engineering |
© 2024 by SSRG - IJECE Journal |
Volume 11 Issue 9 |
Year of Publication : 2024 |
Authors : Revelle Akshara, Ajay Jain |
How to Cite?
Revelle Akshara, Ajay Jain, "Transforming E-commerce with a Novel Multifaceted Data-Decision Framework," SSRG International Journal of Electronics and Communication Engineering, vol. 11, no. 9, pp. 120-134, 2024. Crossref, https://doi.org/10.14445/23488549/IJECE-V11I9P112
Abstract:
With the quickly evolving nature of e-commerce, numerous businesses face a range of challenges, such as constrained investment, customer dissatisfaction, delivery delays, product-market misalignment, and a lack of understanding of customer preferences and satisfaction. These issues often contribute to the failure of many enterprises. This paper proposes a Novel Multifaceted Data-Decision Framework designed to navigate these challenges by guiding businesses from data collection to actionable insights using advanced analytics. The framework integrates various essential elements: data collection and processing, descriptive analytics to discern past occurrences, diagnostic analytics to unveil causative factors, and predictive analytics to estimate what could happen in the future. Prescriptive analytics provides detailed advice on how to respond and machine learning classifiers to analyze complex datasets. The framework's effectiveness is illustrated using an e-commerce dataset, showing how businesses of all sizes can leverage analytics for informed decision-making. By adopting this Multifaceted Data-Decision Framework, e-commerce businesses, from small to large-scale, can make informed decisions using data that enhance customer fulfilment, streamline operations, and promote sustainable growth, enabling them to overcome challenges and succeed in a competitive environment.
Keywords:
Data-decision framework, Descriptive analytics, Diagnostic analytics, E-Commerce, Machine learning classifiers, Predictive analytics, Prescriptive analytics.
References:
[1] Thomas H. Davenport, and Jill Dyché, “Big Data in Big Companies,” International Institute for Analytics, vol. 3, pp. 1-31, 2013.
[Google Scholar] [Publisher Link]
[2] Amir Gandomi, and Murtaza Haider, “Beyond the Hype: Big Data Concepts, Methods, and Analytics,” International Journal of Information Management, vol. 35, no. 2, pp. 137-144, 2015.
[CrossRef] [Google Scholar] [Publisher Link]
[3] Hsinchun Chen, Roger H.L. Chiang, and Veda C. Storey, “Business Intelligence and Analytics: From Big Data to Big Impact,” MIS quarterly, vol. 36, no. 4, pp. 1165-1188, 2012.
[CrossRef] [Google Scholar] [Publisher Link]
[4] Matthew A. Waller, and Stanley E. Fawcett, “Data Science, Predictive Analytics, and Big Data: A Revolution that Will Transform Supply Chain Design and Management,” Journal of Business Logistics, vol. 34, no. 2, pp. 77-84, 2013.
[CrossRef] [Google Scholar] [Publisher Link]
[5] Shahriar Akter et al., “How to Improve Firm Performance Using Big Data Analytics Capability and Business Strategy Alignment?,” International Journal of Production Economics, vol. 182, pp. 113-131, 2016.
[CrossRef] [Google Scholar] [Publisher Link]
[6] Jan Kietzmann, Jeannette Paschen, and Emily Treen, “Artificial Intelligence in Advertising: How Marketers Can Leverage Artificial Intelligence Along the Customer Journey,” Journal of Advertising Research, vol. 58, no. 3, pp. 263-267, 2018.
[CrossRef] [Google Scholar] [Publisher Link]
[7] Dimitris Bertsimas, and Nathan Kallus, “From Predictive to Prescriptive Analytics,” Management Science, vol. 66, no. 3, pp. 1025-1044, 2019.
[CrossRef] [Google Scholar] [Publisher Link]
[8] Sohini Roychowdhury et al., “Categorizing Online Shopping Behavior from Cosmetics to Electronics: An Analytical Framework,” arXiv, 2020.
[CrossRef] [Google Scholar] [Publisher Link]
[9] Weiqing Zhuang et al., “Big Data Analytics in E-commerce for the US and China through Literature Reviewing,” Journal of Systems Science and Information, vol. 9, no. 1, pp. 16-44, 2021.
[CrossRef] [Google Scholar] [Publisher Link]
[10] Mingxi Chen, and Qunying Du, “E-commerce Marketing Strategy Based on Big Data Statistical Analysis,” 2021 13th International Conference on Measuring Technology and Mechatronics Automation (ICMTMA), Beihai, China, pp. 686-689, 2021.
[CrossRef] [Google Scholar] [Publisher Link]
[11] Ayman Abdalmajeed Alsmadi et al., “Big Data Analytics and Innovation in E-Commerce: Current Insights and Future Directions,” Journal of Financial Services Marketing, pp. 1-18, 2023.
[CrossRef] [Google Scholar] [Publisher Link]
[12] Anagha Ramkumar et al., “Big Data Analytics and its Application in E-commerce,” AIP Conference Proceedings, 2023.
[CrossRef] [Google Scholar] [Publisher Link]
[13] Shivinder Nijjer et al., “Customer Analytics: Deep Dive into Customer Data,” Encyclopedia of Data Science and Machine Learning, pp. 1092-1107, 2023.
[CrossRef] [Google Scholar] [Publisher Link]
[14] Foster Provost, and Tom Fawcett, Data Science for Business: What you need to know about Data Mining and Data-Analytic Thinking, O'Reilly Media, 2013.
[Google Scholar] [Publisher Link]
[15] Leo Breiman, “Random Forests,” Machine Learning, vol. 45, pp. 5-32, 2001.
[CrossRef] [Google Scholar] [Publisher Link]
[16] Jerome H. Friedman, “Greedy Function Approximation: A Gradient Boosting Machine,” Annals of Statistics, vol. 29, no. 5, pp. 1189-1232, 2001.
[CrossRef] [Google Scholar] [Publisher Link]
[17] Yoav Freund, and Robert E. Schapire, “A Decision-Theoretic Generalization of on-Line Learning and an Application to Boosting,” Journal of Computer and System Sciences, vol. 55, no. 1, pp. 119-139, 1997.
[CrossRef] [Google Scholar] [Publisher Link]
[18] Tianqi Chen, and Carlos Guestrin, “XgBoost: A Scalable Tree Boosting System,” Proceedings of the 22nd ACM SIGKDD International Conference on Knowledge Discovery and Data Mining, pp. 785-794, 2016.
[CrossRef] [Google Scholar] [Publisher Link]
[19] Guolin Ke et al., “LightGBM: A Highly Efficient Gradient Boosting Decision Tree,” Advances in Neural Information Processing Systems, 2017.
[Google Scholar] [Publisher Link]
[20] Leo Breiman, “Bagging Predictors,” Machine Learning, vol. 24, pp. 123-140, 1996.
[CrossRef] [Google Scholar] [Publisher Link]
[21] David H. Wolpert, “Stacked Generalization,” Neural Networks, vol. 5, no. 2, pp. 241-259, 1992.
[CrossRef] [Google Scholar] [Publisher Link]
[22] R. Divakar et al., “Prescriptive and Predictive Analysis of Intelligible Big Data,” 2022 6th International Conference on Computing Methodologies and Communication (ICCMC), Erode, India, pp. 845-851, 2022.
[CrossRef] [Google Scholar] [Publisher Link]
[23] Sankalp Jain, and Naresh Kumar Nagwani, “A Study on Significance of Feature Selection on Product Valuation,” 2021 6th International Conference for Convergence in Technology (I2CT), Maharashtra, India, pp. 1-5, 2021.
[CrossRef] [Google Scholar] [Publisher Link]