Design of Multivariate Lung Cancer Dataset and Multistage Pre-processing to Augment Prediction of Complex Lung Cancer Datasets Using Data Mining Techniques
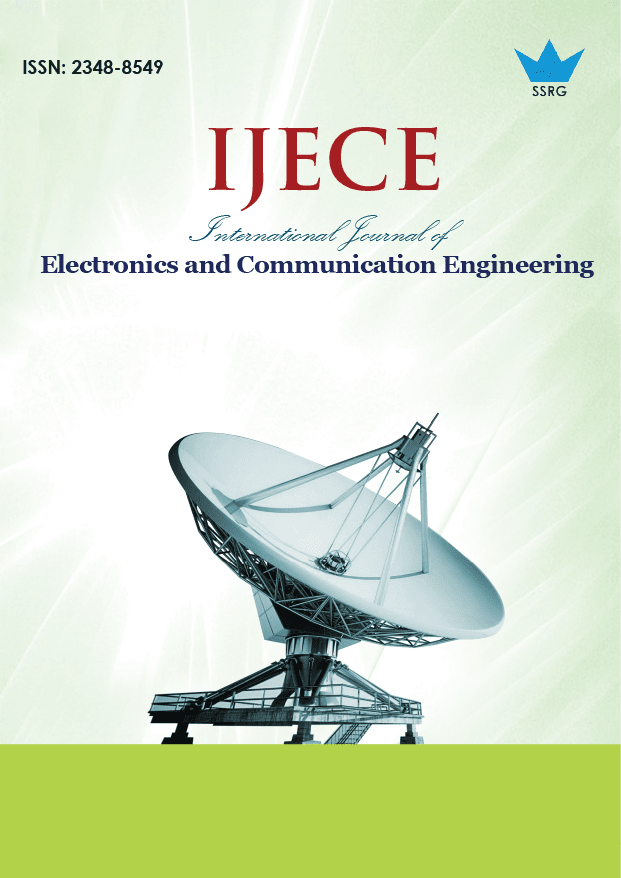
International Journal of Electronics and Communication Engineering |
© 2024 by SSRG - IJECE Journal |
Volume 11 Issue 9 |
Year of Publication : 2024 |
Authors : M. Amenraj, R. Vidya |
How to Cite?
M. Amenraj, R. Vidya, "Design of Multivariate Lung Cancer Dataset and Multistage Pre-processing to Augment Prediction of Complex Lung Cancer Datasets Using Data Mining Techniques," SSRG International Journal of Electronics and Communication Engineering, vol. 11, no. 9, pp. 163-173, 2024. Crossref, https://doi.org/10.14445/23488549/IJECE-V11I9P115
Abstract:
Progressions in genomic research have led to an increased focus on Single Nucleotide Polymorphisms (SNPs) as potential markers for various diseases, including lung cancer. This study introduces a novel approach to enhance the predictive accuracy of ensemble machine learning classifiers and design of a Multivariate Dataset for Lung Cancer for SNP-associated lung cancer through a three-stage pre-processing framework called Lung Cancer Data Pre-processing and Feature Engineering (LC-PreProFE). The framework comprises numerical analysis at the initial stage, followed by regression analysis and segmentation at the final stage objected to eliminate irrelevant features and optimize the construction of a multivariate dataset. The first stage involves rigorous numerical analysis to identify and quantify the significance of each SNP within the dataset. The stage eliminated 2 features with a 4% improvement in best predictions. The refined dataset undergoes regression analysis to model the relationships between identified SNPs and to filter out redundant or correlated features. This stage eliminated 4 features. Finally, in the segmentation process, 7 irrelevant features were eliminated. After completion of three stages, it was found that the accuracy has improved after irrelevant feature removal and the Region of Curve value reduced to show augmentation in the overall preprocessing stage.
Keywords:
Single Nucleotide Polymorphisms (SNPs), Multivariate lung cancer dataset, Lung Cancer Data Pre-processing and Feature Engineering (LC-PreProFE), Ensemble machine learning models, Irrelevant feature engineering.
References:
[1] Chang Gu et al., “A Cloud-Based Deep Learning Model in Heterogeneous Data Integration System for Lung Cancer Detection in Medical Industry 4.0,” Journal of Industrial Information Integration, vol. 30, 2022.
[CrossRef] [Google Scholar] [Publisher Link]
[2] V. Vasudha Rani, Smritilekha Das, and Tamal Kr. Kundu, “Risk Prediction Model for Lung Cancer Disease Using Machine Learning Techniques,” Innovations in Computer Science and Engineering, Lecture Notes in Networks and Systems, Singapore, vol. 385, pp. 417-425, 2022.
[CrossRef] [Google Scholar] [Publisher Link]
[3] Roman Jaksik, and Jarosław Śmieja, “Prediction of Lung Cancer Survival Based on Multiomic Data,” Intelligent Information and Database Systems, Lecture Notes in Computer Science, Ho Chi Minh City, Vietnam, vol. 13758, pp. 116-127, 2022.
[CrossRef] [Google Scholar] [Publisher Link]
[4] Negar Maleki, and Seyed Taghi Akhavan Niaki, “An Intelligent Algorithm for Lung Cancer Diagnosis Using Extracted Features from Computerized Tomography Images,” Healthcare Analytics, vol. 3, pp. 1-16, 2023.
[CrossRef] [Google Scholar] [Publisher Link]
[5] Devendra K. Tayal et al., “A Novel Hybrid Approach for Dimensionality Reduction in Microarray Data,” Proceedings of the International Conference on Intelligent Computing, Communication and Information Security, Algorithms for Intelligent Systems, Singapore, pp. 213-226, 2023.
[CrossRef] [Google Scholar] [Publisher Link]
[6] Rabia Musheer Aziz, “Application of Nature Inspired Soft Computing Techniques for Gene Selection: A Novel Frame Work for Classification of Cancer,” Soft Computing, vol. 26, pp. 12179-12196, 2022.
[CrossRef] [Google Scholar] [Publisher Link]
[7] Muthuperumal Periyaperumal Ramkumar et al., “Deep Maxout Network for Lung Cancer Detection Using Optimization Algorithm in Smart Internet of Things,” Concurrency and Computation: Practice and Experience, vol. 34, no. 25, 2022.
[CrossRef] [Google Scholar] [Publisher Link]
[8] C. Venkatesan et al., “Efficient Machine Learning Technique for Tumor Classification Based on Gene Expression Data,” 2022 8th International Conference on Advanced Computing and Communication Systems, Coimbatore, India, pp. 1982-1986, 2022.
[CrossRef] [Google Scholar] [Publisher Link]
[9] Christopher J. Hanley et al., “Single-Cell Analysis Reveals Prognostic Fibroblast Subpopulations Linked to Molecular and Immunological Subtypes of Lung Cancer,” Nature Communications, vol. 14, pp. 1-18, 2023.
[CrossRef] [Google Scholar] [Publisher Link]
[10] Kanchan Pradhan, Priyanka Chawla, and Sanyog Rawat, “A Deep Learning-Based Approach for Detection of Lung Cancer Using Self Adaptive Sea Lion Optimization Algorithm (SA-SLnO),” Journal of Ambient Intelligence and Humanized Computing, vol. 14, pp. 12933-12947, 2023.
[CrossRef] [Google Scholar] [Publisher Link]
[11] Guillaume Chassagnon et al., “Artificial Intelligence in Lung Cancer: Current Applications and Perspectives,” Japanese Journal of Radiology, vol. 41, pp. 235-244, 2023.
[CrossRef] [Google Scholar] [Publisher Link]
[12] Kai Zhang et al., “Content-Based Image Retrieval with a Convolutional Siamese Neural Network: Distinguishing Lung Cancer and Tuberculosis in CT Images,” Computers in Biology and Medicine, vol. 140, 2022.
[CrossRef] [Google Scholar] [Publisher Link]
[13] Na Sun et al., “A Novel 14-Gene Signature for Overall Survival in Lung Adenocarcinoma Based on the Bayesian Hierarchical Cox Proportional Hazards Model,” Scientific Reports, vol. 12, pp. 1-11, 2022.
[CrossRef] [Google Scholar] [Publisher Link]
[14] Sergey P. Primakov et al., “Automated Detection and Segmentation of Non-Small Cell Lung Cancer Computed Tomography Images,” Nature Communications, vol. 13, pp. 1-12, 2022.
[CrossRef] [Google Scholar] [Publisher Link]
[15] Ebtasam Ahmad Siddiqui, Vijayshri Chaurasia, and Madhu Shandilya, “Detection and Classification of Lung Cancer Computed Tomography Images Using a Novel Improved Deep Belief Network with Gabor Filters,” Chemometrics and Intelligent Laboratory Systems, vol. 235, 2023.
[CrossRef] [Google Scholar] [Publisher Link]
[16] Hariprasath Manoharan et al., “Aerial Separation and Receiver Arrangements on Identifying Lung Syndromes Using the Artificial Neural Network,” Computational Intelligence and Neuroscience, vol. 2022, no. 1, pp. 1-8, 2022.
[CrossRef] [Google Scholar] [Publisher Link]
[17] Jovan Andjelkovic et al., “Sequential Machine Learning in Prediction of Common Cancers,” Informatics in Medicine Unlocked, vol. 30, pp. 1-10, 2022.
[CrossRef] [Google Scholar] [Publisher Link]
[18] Nashat Alrefai, and Othman Ibrahim, “Optimized Feature Selection Method Using Particle Swarm Intelligence with Ensemble Learning for Cancer Classification Based on Microarray Datasets,” Neural Computing and Applications, vol. 34, pp. 13513-13528, 2022.
[CrossRef] [Google Scholar] [Publisher Link]
[19] Ridhima Rani et al., “Big Data Dimensionality Reduction Techniques in IoT: Review, Applications and Open Research Challenges,” Cluster Computing, vol. 25, pp. 4027-4049, 2022.
[CrossRef] [Google Scholar] [Publisher Link]
[20] P.N. Senthil Prakash, and N. Rajkumar, “HSVNN: An Efficient Medical Data Classification Using Dimensionality Reduction Combined with Hybrid Support Vector Neural Network,” The Journal of Supercomputing, vol. 78, pp. 15439-15462, 2022.
[CrossRef] [Google Scholar] [Publisher Link]
[21] Wei Yao et al., “Noninvasive Method for Predicting the Expression of Ki67 and Prognosis in Non-Small-Cell Lung Cancer Patients: Radiomics,” Journal of Healthcare Engineering, vol. 2022, no. 1, pp. 1-9, 2022.
[CrossRef] [Google Scholar] [Publisher Link]
[22] Tarneem Elemam, and Mohamed Elshrkawey, “A Highly Discriminative Hybrid Feature Selection Algorithm for Cancer Diagnosis,” The Scientific World Journal, vol. 2022, no. 1, pp. 1-15, 2022.
[CrossRef] [Google Scholar] [Publisher Link]
[23] Surbhi Gupta, and Yogesh Kumar, “Cancer Prognosis Using Artificial Intelligence-Based Techniques,” SN Computer Science, vol. 3, 2022.
[CrossRef] [Google Scholar] [Publisher Link]
[24] Bikram Kar, and Bikash Kanti Sarkar, “A Hybrid Feature Reduction Approach for Medical Decision Support System,” Mathematical Problems in Engineering, vol. 2022, no. 1, pp. 1-20, 2022.
[CrossRef] [Google Scholar] [Publisher Link]
[25] Suli Liu, and Wu Yao, “Prediction of Lung Cancer Using Gene Expression and Deep Learning with KL Divergence Gene Selection,” BMC Bioinformatics, vol. 23, pp. 1-11, 2022.
[CrossRef] [Google Scholar] [Publisher Link]
[26] Saksham Gupta et al., “A Novel Transfer Learning-Based Model for Ultrasound Breast Cancer Image Classification,” Computational Vision and Bio-Inspired Computing, Advances in Intelligent Systems and Computing, Singapore, vol. 1439, pp. 511- 523, 2023.
[CrossRef] [Google Scholar] [Publisher Link]
[27] Moshood A. Hambali et al., “Feature Selection and Computational Optimization in High-Dimensional Microarray Cancer Datasets Via InfoGain-Modified Bat Algorithm,” Multimedia Tools and Applications, vol. 81, pp. 36505-36549, 2022.
[CrossRef] [Google Scholar] [Publisher Link]