Current Developments in Crop Disease Identification Using Computer Vision: A Critical Review of Related Works and Techniques
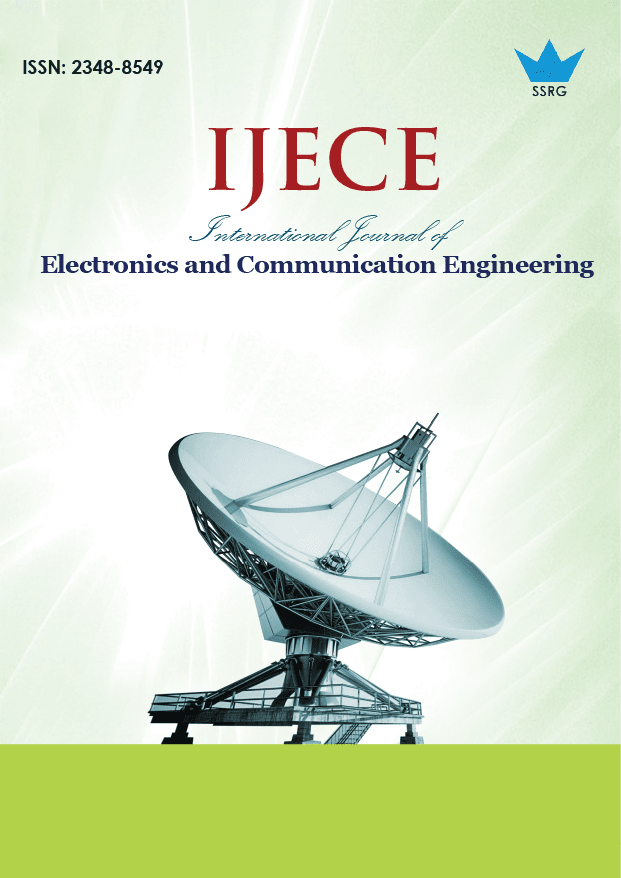
International Journal of Electronics and Communication Engineering |
© 2024 by SSRG - IJECE Journal |
Volume 11 Issue 9 |
Year of Publication : 2024 |
Authors : Anandraddi Naduvinamani, Jayshri Rudagi, Mallikarjun Anandhalli |
How to Cite?
Anandraddi Naduvinamani, Jayshri Rudagi, Mallikarjun Anandhalli, "Current Developments in Crop Disease Identification Using Computer Vision: A Critical Review of Related Works and Techniques," SSRG International Journal of Electronics and Communication Engineering, vol. 11, no. 9, pp. 174-191, 2024. Crossref, https://doi.org/10.14445/23488549/IJECE-V11I9P116
Abstract:
Computer vision and especially deep learning play a pivotal role in the recognition of patterns in diverse kinds of images. Recent years have seen increased attention from both academic and business communities for autonomous learning in this field. Deep Learning (DL) based strategies have found much usage in areas like NLP, speech recognition, and video analysis. At the same time, it has become a centre of attraction for scientists studying methods for agricultural plant protection, such as pest spread assessments or the identification of plant diseases. Researchers may avoid the pitfalls of selecting disease spot qualities subjectively, derive more accurate plant disease features, and speed up the rate of technological transformation, all by disease detection in plants using deep learning. In this study, we will take a look at how far the DL mechanism has come in the arena of detecting ailments in crop leaves during the past few years. In this article, we converse about the cutting-edge progressions in the automatic diagnosis of leaf diseases, utilizing both deep learning and standard image processing methods, as well as highlight the obstacles that must be overcome.
Keywords:
Deep Learning (DL), Natural language processing, Disease spot qualities, Identification of plant diseases.
References:
[1] Shima Ramesh et al., “Plant Disease Detection Using Machine Learning,” 2018 International Conference on Design Innovations for 3Cs Compute Communicate Control, Bangalore, India, pp. 41-45, 2018.
[CrossRef] [Google Scholar] [Publisher Link]
[2] Sunil S. Harakannanavar et al., “Plant Leaf Disease Detection Using Computer Vision and Machine Learning Algorithms,” Global Transitions Proceedings, vol. 3, no. 1, pp. 305-310, 2022.
[CrossRef] [Google Scholar] [Publisher Link]
[3] Anuradha Chug et al., “A Novel Framework for Image-Based Plant Disease Detection using Hybrid Deep Learning Approach,” Soft Computing, vol. 27, pp. 13613-13638, 2023.
[CrossRef] [Google Scholar] [Publisher Link]
[4] Muhammad Hammad Saleem, Johan Potgieter, and Khalid Mahmood Arif, “A Performance-Optimized Deep Learning-Based Plant Disease Detection Approach for Horticultural Crops of New Zealand,” IEEE Access, vol. 10, pp. 89798-89822, 2022.
[CrossRef] [Google Scholar] [Publisher Link]
[5] M. Nandhini et al., “Deep Learning Model of Sequential Image Classifier for Crop Disease Detection in Plantain Tree Cultivation,” Computers and Electronics in Agriculture, vol. 197, 2022.
[CrossRef] [Google Scholar] [Publisher Link]
[6] R. Arumuga Arun, and S. Umamaheswari, “Effective Multi-Crop Disease Detection Using Pruned Complete Concatenated Deep Learning Model,” Expert Systems with Applications, vol. 213, 2023.
[CrossRef] [Google Scholar] [Publisher Link]
[7] Junde Chen et al., “Stacking Ensemble Model of Deep Learning for Plant Disease Recognition,” Journal of Ambient Intelligence and Humanized Computing, vol. 14, pp. 12359-12372, 2023.
[CrossRef] [Google Scholar] [Publisher Link]
[8] S.K. Mahmudul Hassan, and Arnab Kumar Maji, “Plant Disease Identification Using a Novel Convolutional Neural Network,” IEEE Access, vol. 10, pp. 5390-5401, 2022.
[CrossRef] [Google Scholar] [Publisher Link]
[9] Poornima Singh Thakur, Tanuja Sheorey, and Aparajita Ojha, “VGG-ICNN: A Lightweight CNN Model for Crop Disease Identification,” Multimedia Tools and Applications, vol. 82, pp. 497-520, 2023.
[CrossRef] [Google Scholar] [Publisher Link]
[10] Yousef Methkal Abd Algani et al., “Leaf Disease Identification and Classification Using Optimized Deep Learning,” Measurement: Sensors, vol. 25, pp. 1-6, 2023.
[CrossRef] [Google Scholar] [Publisher Link]
[11] J. Arun Pandian et al., “Plant Disease Detection Using Deep Convolutional Neural Network,” Applied Sciences, vol. 12, no. 14, pp. 1-14, 2022.
[CrossRef] [Google Scholar] [Publisher Link]
[12] Rabbia Mahum et al., “A Novel Framework for Potato Leaf Disease Detection Using an Efficient Deep Learning Model,” Human and Ecological Risk Assessment: An International Journal, vol. 29, no. 2, pp. 303-326, 2022.
[CrossRef] [Google Scholar] [Publisher Link]
[13] Zhaoyi Chen et al., “Plant Disease Recognition Model Based on Improved YOLOv5,” Agronomy, vol. 12, no. 2, pp. 1-14, 2022.
[CrossRef] [Google Scholar] [Publisher Link]
[14] Yusuke Kawasaki et al., “Basic Study of Automated Diagnosis of Viral Plant Diseases Using Convolutional Neural Networks,” Advances in Visual Computing, Lecture Notes in Computer Science, vol. 9475, pp. 638-645, 2015.
[CrossRef] [Google Scholar] [Publisher Link]
[15] Halil Durmuş, Ece Olcay Güneş, and Mürvet Kırcı, “Disease Detection on the Leaves of the Tomato Plants by Using Deep Learning,” 2017 6th International Conference on Agro-Geoinformatics, Fairfax, VA, USA, pp. 1-5, 2017.
[CrossRef] [Google Scholar] [Publisher Link]
[16] Peng Jiang et al., “Real-Time Detection of Apple Leaf Diseases using Deep Learning Approach Based on Improved Convolutional Neural Networks, IEEE Access, vol. 7, pp. 59069-59080, 2019.
[CrossRef] [Google Scholar] [Publisher Link]
[17] Jayme Garcia Arnal Barbedo, “Plant Disease Identification from Individual Lesions and Spots Using Deep Learning,” Biosystems Engineering, vol. 180, pp. 96-107, 2019.
[CrossRef] [Google Scholar] [Publisher Link]
[18] Sharifah Farhana Syed-Ab-Rahman, Mohammad Hesam Hesamian, and Mukesh Prasad, “Citrus Disease Detection and Classification Using End-to-End Anchor-Based Deep Learning Model,” Applied Intelligence, vol. 52, pp. 927-938, 2022.
[CrossRef] [Google Scholar] [Publisher Link]
[19] Wu Dake, and Ma Chengweim, “The Support Vector Machine (SVM) Based Near-Infrared Spectrum Recognition of Leaves Infected by the Leafminers,” First International Conference on Innovative Computing, Information and Control (ICICIC'06), Beijing, China, vol. 1, pp. 448-451, 2006.
[CrossRef] [Google Scholar] [Publisher Link]
[20] Julio M. Duarte-Carvajalino et al., “Evaluating Late Blight Severity in Potato Crops using Unmanned Aerial Vehicles and Machine Learning Algorithms,” Remote Sensing, vol. 10, no. 10, pp. 1-17, 2018.
[CrossRef] [Google Scholar] [Publisher Link]
[21] Shan-e-Ahmed Raza et al., “Automatic Detection of Diseased Tomato Plants Using Thermal and Stereo Visible Light Images,” PLoS One, vol. 10, no. 4, pp. 1-20, 2015.
[CrossRef] [Google Scholar] [Publisher Link]
[22] Usama Mokhtar et al., “Identifying Two of Tomatoes Leaf Viruses Using Support Vector Machine,” Information Systems Design and Intelligent Applications: Proceedings of Second International Conference INDIA 2015, pp. 771-782, 2015.
[CrossRef] [Google Scholar] [Publisher Link]
[23] Mohammed Brahimi, Kamel Boukhalfa, and Abdelouahab Moussaoui, “Deep Learning for Tomato Diseases: Classification and Symptoms Visualization,” Applied Artificial Intelligence, vol. 31, no. 4, pp. 299-315, 2017.
[CrossRef] [Google Scholar] [Publisher Link]
[24] Ian Goodfellow et al., “Generative Adversarial Nets,” Advances in Neural Information Processing Systems, vol. 27, 2014.
[Google Scholar] [Publisher Link]
[25] Marko Arsenovic et al., “Solving Current Limitations of Deep Learning Based Approaches for Plant Disease Detection,” Symmetry, vol. 11, no. 7, pp. 1-21, 2019.
[CrossRef] [Google Scholar] [Publisher Link]
[26] Yan Zhang et al., “Automatic Plant Disease Detection based on Tranvolution Detection Network with GAN Modules using Leaf Images,” Frontiers in Plant Science, vol. 13, pp. 1-20, 2022.
[CrossRef] [Google Scholar] [Publisher Link]
[27] Yiping Chen, and Qiufeng Wu, “Grape Leaf Disease Identification with Sparse Data via Generative Adversarial Networks and Convolutional Neural Networks,” Precision Agriculture, vol. 24, pp. 235-253, 2023.
[CrossRef] [Google Scholar] [Publisher Link]
[28] Mamta Gehlot, and Geeta Chhabra Gandhi, “EffiNet-TS”: A Deep Interpretable Architecture Using EfficientNet for Plant Disease Detection and Visualization,” Journal of Plant Diseases and Protection, vol. 130, pp. 413-430, 2023.
[CrossRef] [Google Scholar] [Publisher Link]
[29] Muhammad Attique Khan et al., “Cucumber Leaf Diseases Recognition Using Multi Level Deep Entropy-ELM Feature Selection,” Applied Sciences, vol. 12, no. 2, pp. 1-19, 2022.
[CrossRef] [Google Scholar] [Publisher Link]
[30] L.G. Divyanth, Aanis Ahmad, and Dharmendra Saraswa, “A Two-Stage Deep-Learning Based Segmentation Model for Crop Disease Quantification based on Corn Field Imagery,” Smart Agricultural Technology, vol. 3, pp. 1-12, 2023.
[CrossRef] [Google Scholar] [Publisher Link]
[31] Rabindra Nath Nandi et al., “Device-Friendly Guava Fruit and Leaf Disease Detection Using Deep Learning,” Machine Intelligence and Emerging Technologies, pp. 49-59, 2023.
[CrossRef] [Google Scholar] [Publisher Link]
[32] Lai Zhi Yong et al., “Automatic Disease Detection of Basal Stem Rot Using Deep Learning and Hyperspectral Imaging,” Agriculture, vol. 13, no. 1, pp. 1-16, 2022.
[CrossRef] [Google Scholar] [Publisher Link]
[33] Wei Ma et al., “Crop Disease Detection Against Complex Background Based on Improved Atrous Spatial Pyramid Pooling,” Electronics, vol. 12, no. 1, pp. 1-14, 2023.
[CrossRef] [Google Scholar] [Publisher Link]
[34] Antonio Guerrero-Ibañez, and Angelica Reyes-Muñoz, “Monitoring Tomato Leaf Disease through Convolutional Neural Networks,” Electronics, vol. 12, no. 1, pp. 1-19, 2023.
[CrossRef] [Google Scholar] [Publisher Link]
[35] Alaa Saeed et al., “Smart Detection of Tomato Leaf Diseases Using Transfer Learning-Based Convolutional Neural Networks,” Agriculture, vol. 13, no. 1, pp. 1-14, 2023.
[CrossRef] [Google Scholar] [Publisher Link]
[36] Aanis Ahmad, Aly El Gamal, and Dharmendra Saraswat, “Toward Generalization of Deep Learning-Based Plant Disease Identification under Controlled and Field Conditions,” IEEE Access, vol. 11, pp. 9042-9057, 2023.
[CrossRef] [Google Scholar] [Publisher Link]
[37] Hasan Ulutaş, and Veysel Aslantaş, “Design of Efficient Methods for the Detection of Tomato Leaf Disease Utilizing Proposed Ensemble CNN Model,” Electronics, vol. 12, no. 4, pp. 1-21, 2023.
[CrossRef] [Google Scholar] [Publisher Link]
[38] Mercelin Francis et al., “Smart Farm-Care Using a Deep Learning Model on Mobile Phones,” Emerging Science Journal, vol. 7, no. 2, pp. 480-497, 2023.
[CrossRef] [Google Scholar] [Publisher Link]
[39] Jan Behmann, Jörg Steinrücken, and Lutz Plümer, “Detection of Early Plant Stress Responses in Hyperspectral Images,” ISPRS Journal of Photogrammetry and Remote Sensing, vol. 93, pp. 98-111, 2014.
[CrossRef] [Google Scholar] [Publisher Link]
[40] Youssef Es-Saady et al., “Automatic Recognition of Plant Leaves Diseases Based on Serial Combination of Two SVM Classifiers,” 2016 International Conference on Electrical and Information Technologies, Tangiers, Morocco, pp. 561-566, 2016.
[CrossRef] [Google Scholar] [Publisher Link]
[41] Chuanqi Xie, Ce Yang, and Yong He, “Hyperspectral Imaging for Classification of Healthy and Gray Mold Diseased Tomato Leaves with Different Infection Severities,” Computers and Electronics in Agriculture, vol. 135, pp. 154-162, 2017.
[CrossRef] [Google Scholar] [Publisher Link]
[42] Romain Bebronne et al., “In-Field Proximal Sensing of Septoria Tritici Blotch, Stripe Rust and Brown Rust in Winter Wheat by Means of Reflectance and Textural Features from Multispectral Imagery,” Biosystems Engineering, vol. 197, pp. 257-269, 2020.
[CrossRef] [Google Scholar] [Publisher Link]
[43] Ismail El Massi et al., “Hybrid Combination of Multiple SVM Classifiers for Automatic Recognition of the Damages and Symptoms on Plant Leaves,” Image and Signal Processing, Lecture Notes in Computer Science, vol. 9680, pp. 40-50, 2016.
[CrossRef] [Google Scholar] [Publisher Link]
[44] Shan Suthaharan, Support Vector Machine, Machine Learning Models and Algorithms for Big Data Classification, pp. 207-235, 2016.
[CrossRef] [Google Scholar] [Publisher Link]
[45] Y.H. Gu et al., “BLITE-SVR: New Forecasting Model for Late Blight on Potato Using Support-Vector Regression,” Computers and Electronics in Agriculture, vol. 130, pp. 169-176, 2016.
[CrossRef] [Google Scholar] [Publisher Link]
[46] Anshul Bhatia, Anuradha Chug, and Amit Prakash Singh, “Hybrid SVM-LR Classifier for Powdery Mildew Disease Prediction in Tomato Plant,” 2020 7th International Conference on Signal Processing and Integrated Networks, Noida, India, pp. 218-223, 2020.
[CrossRef] [Google Scholar] [Publisher Link]
[47] Harshadkumar B. Prajapati, Jitesh Shah, and Vipul K. Dabhi, “Detection and Classification of Rice Plant Diseases,” Intelligent Decision Technologies, vol. 11, no. 3, pp. 357-373, 2017.
[CrossRef] [Google Scholar] [Publisher Link]
[48] Daniel Atherton, Ruplal Choudhary, and Dennis Watson, “Hyperspectral Remote Sensing for Advanced Detection of Early Blight (Alternaria solani) Disease in Potato (Solanum tuberosum) Plants Prior to Visual Disease Symptoms,” Proceedings of the 2017 ASABE Annual International Meeting, 2017.
[CrossRef] [Google Scholar] [Publisher Link]
[49] Chuanqi Xie et al., “Detection of Early Blight and Late Blight Diseases on Tomato Leaves Using Hyperspectral Imaging,” Scientific Reports, vol. 5, pp. 1-11, 2015.
[CrossRef] [Google Scholar] [Publisher Link]
[50] Hongyan Zhu et al., “Hyperspectral Imaging for Presymptomatic Detection of Tobacco Disease with Successive Projections Algorithm and Machine-Learning Classifiers,” Scientific Reports, vol. 7, pp. 1-12, 2017.
[CrossRef] [Google Scholar] [Publisher Link]
[51] Mohammed Brahimi et al., Deep Learning for Plant Diseases: Detection and Saliency Map Visualisation, Human and Machine Learning, Springer, Cham, pp. 93-117, 2018.
[CrossRef] [Google Scholar] [Publisher Link]
[52] Xiu Jin et al., “Classifying Wheat Hyperspectral Pixels of Healthy Heads and Fusarium Head Blight Disease Using a Deep Neural Network in the Wild Field,” Remote Sensing, vol. 10, no. 3, pp. 1-20, 2018.
[CrossRef] [Google Scholar] [Publisher Link]
[53] Artzai Picon et al., “Crop Conditional Convolutional Neural Networks for Massive Multi-Crop Plant Disease Classification Over Cell Phone Acquired Images Taken on Real Field Conditions,” Computers and Electronics in Agriculture, vol. 167, 2019.
[CrossRef] [Google Scholar] [Publisher Link]
[54] Azeddine Elhassouny, and Florentin Smarandache, “Smart Mobile Application to Recognize Tomato Leaf Diseases Using Convolutional Neural Networks,” 2019 International Conference of Computer Science and Renewable Energies, Agadir, Morocco, pp. 1-4, 2019.
[CrossRef] [Google Scholar] [Publisher Link]
[55] Utpal Barman et al., “Comparison of Convolution Neural Networks for Smartphone Image Based Real Time Classification of Citrus Leaf Disease,” Computers and Electronics in Agriculture, vol. 177, 2020.
[CrossRef] [Google Scholar] [Publisher Link]
[56] Chongke Bi et al., “MobileNet Based Apple Leaf Diseases Identification,” Mobile Networks and Applications, vol. 27, pp. 172-180, 2022.
[CrossRef] [Google Scholar] [Publisher Link]
[57] Maryam Ouhami et al., “Deep Transfer Learning Models for Tomato Disease Detection,” Image Signal Processing, 9 th International Conference, Marrakesh, Morocco, pp. 65-73, 2020.
[CrossRef] [Google Scholar] [Publisher Link]
[58] Ümit Atila et al., “Plant Leaf Disease Classification using EfficientNet Deep Learning Model,” Ecological Informatics, vol. 61, 2021.
[CrossRef] [Google Scholar] [Publisher Link]
[59] X. Wang et al., “Spectral Prediction of Phytophthora Infestans Infection on Tomatoes Using Artificial Neural Network (ANN), International Journal of Remote Sensing, vol. 29, no. 6, pp. 1693-1706, 2008.
[CrossRef] [Google Scholar] [Publisher Link]
[60] Sarah L. MacDonald et al., “Remote Hyperspectral Imaging of Grapevine Leafroll-Associated Virus 3 in Cabernet Sauvignon Vineyards,” Computers and Electronics in Agriculture, vol. 130, pp. 109-117, 2016.
[CrossRef] [Google Scholar] [Publisher Link]
[61] Johanna Albetis et al., “Detection of Flavescence Dorée Grapevine Disease Using Unmanned Aerial Vehicle (UAV) Multispectral Imagery,” Remote Sensing, vol. 9, no. 4, pp. 1-20, 2017.
[CrossRef] [Google Scholar] [Publisher Link]
[62] Everton Castelão Tetila et al., “Identification of Soybean Foliar Diseases Using Unmanned Aerial Vehicle Images,” IEEE Geoscience and Remote Sensing Letters, vol. 14, no. 12, pp. 2190-2194, 2017.
[CrossRef] [Google Scholar] [Publisher Link]
[63] Jinya Su et al., “Wheat Yellow Rust Monitoring by Learning from Multispectral UAV Aerial Imagery,” Computers and Electronics in Agriculture, vol. 155, pp. 157-166, 2018.
[CrossRef] [Google Scholar] [Publisher Link]
[64] Jaafar Abdulridha, Ozgur Batuman, and Yiannis Ampatzidis, “UAV-Based Remote Sensing Technique to Detect Citrus Canker Disease Utilizing Hyperspectral Imaging and Machine Learning,” Remote Sensing, vol. 11, no. 11, pp. 1-22, 2019.
[CrossRef] [Google Scholar] [Publisher Link]
[65] Yubin Lan et al., “Comparison of Machine Learning Methods for Citrus Greening Detection on UAV Multispectral Images,” Computers and Electronics in Agriculture, vol. 171, 2020.
[CrossRef] [Google Scholar] [Publisher Link]
[66] T. Poblete et al., “Detection of Xylella Fastidiosa Infection Symptoms with Airborne Multispectral and Thermal Imagery: Assessing Bandset Reduction Performance from Hyperspectral Analysis,” ISPRS Journal of Photogrammetry and Remote Sensing, vol. 162, pp. 27- 40, 2020.
[CrossRef] [Google Scholar] [Publisher Link]
[67] Jaafar Abdulridha et al., “Detecting Powdery Mildew Disease in Squash at Different Stages Using UAV-Based Hyperspectral Imaging and Artificial Intelligence,” Biosystems Engineering, vol. 197, pp. 135-148, 2020.
[CrossRef] [Google Scholar] [Publisher Link]
[68] Tyr Wiesner-Hanks et al., “Image Set for Deep Learning: Field Images of Maize Annotated with Disease Symptoms,” BMC Research Notes, vol. 11, pp. 1-3, 2018.
[CrossRef] [Google Scholar] [Publisher Link]
[69] Mohamed Kerkech, Adel Hafiane, and Raphael Canals, “Deep Leaning Approach with Colorimetric Spaces and Vegetation Indices for Vine Diseases Detection in UAV Images,” Computers and Electronics in Agriculture, vol. 155, pp. 237-243, 2018.
[CrossRef] [Google Scholar] [Publisher Link]
[70] Harvey Wu et al., “Autonomous Detection of Plant Disease Symptoms Directly from Aerial Imagery,” The Plant Phenome Journal, vol. 2, no. 1, pp. 1-9, 2019.
[CrossRef] [Google Scholar] [Publisher Link]
[71] Xin Zhang et al., “A Deep Learning-Based Approach for Automated Yellow Rust Disease Detection from High-Resolution Hyper-Spectral UAV Images,” Remote Sensing, vol. 11, no. 13, pp. 1-16, 2019.
[CrossRef] [Google Scholar] [Publisher Link]
[72] Gensheng Hu et al., “Recognition of Diseased Pinus Trees in UAV Images Using Deep Learning and Adaboost Classifier,” Biosystems Engineering, vol. 194, pp. 138-151, 2020.
[CrossRef] [Google Scholar] [Publisher Link]
[73] Mohamed Kerkech, Adel Hafiane, and Raphael Canals, “VddNet: Vine Disease Detection Network Based on Multispectral Images and Depth Map,” Remote Sensing, vol. 12, no. 20, pp. 1-18, 2020.
[CrossRef] [Google Scholar] [Publisher Link]
[74] Yi Fang, and Ramaraja P. Ramasamy, “Current and Prospective Methods for Plant Disease Detection,” Biosensors, vol. 5, no. 3, pp. 537- 561, 2015.
[CrossRef] [Google Scholar] [Publisher Link]
[75] Federico Martinelli et al., “Advanced Methods of Plant Disease Detection. A Review,” Agronomy for Sustainable Development, vol. 35, pp. 1-25, 2015.
[CrossRef] [Google Scholar] [Publisher Link]
[76] Sudau Eh Teet, and Norhashila Hashim, “Recent Advances of Application of Optical Imaging Techniques for Disease Detection in Fruits and Vegetables: A Review,” Food Control, vol. 152, 2023.
[CrossRef] [Google Scholar] [Publisher Link]
[77] Stefan Thomas et al., “Benefits of Hyperspectral Imaging for Plant Disease Detection and Plant Protection: A Technical Perspective,” Journal of Plant Diseases and Protection, vol. 125, pp. 5-20, 2018.
[CrossRef] [Google Scholar] [Publisher Link]
[78] Tiago Domingues, Tomás Brandão, and João C. Ferreira, “Machine Learning for Detection and Prediction of Crop Diseases and Pests: A Comprehensive Survey,” Agriculture, vol. 12, no. 9, pp. 1-23, 2022.
[CrossRef] [Google Scholar] [Publisher Link]
[79] Anshul Bhatia, Anuradha Chug, and Amit Prakash Singh, “Hybrid SVM-LR Classifier for Powdery Mildew Disease Prediction in Tomato Plant,” 2020 7th International Conference on Signal Processing and Integrated Networks (SPIN), Noida, India, pp. 218-223, 2020.
[CrossRef] [Google Scholar] [Publisher Link]