Enhancing Social Media Fake News Detection Using PsychoLinguistic Multiplicative Attentive Net (PLiMA Net) with LIWC Features
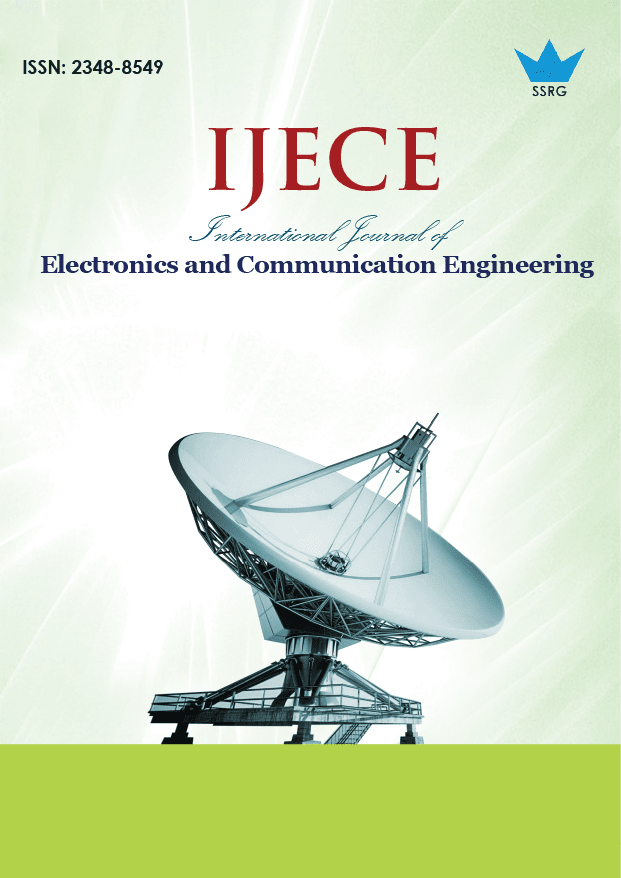
International Journal of Electronics and Communication Engineering |
© 2024 by SSRG - IJECE Journal |
Volume 11 Issue 9 |
Year of Publication : 2024 |
Authors : B. Hemalatha, M. Soranamageswari |
How to Cite?
B. Hemalatha, M. Soranamageswari, "Enhancing Social Media Fake News Detection Using PsychoLinguistic Multiplicative Attentive Net (PLiMA Net) with LIWC Features," SSRG International Journal of Electronics and Communication Engineering, vol. 11, no. 9, pp. 192-206, 2024. Crossref, https://doi.org/10.14445/23488549/IJECE-V11I9P117
Abstract:
Detecting fake news on social media platforms remains a critical challenge due to the rapid dissemination of information and the intricate nature of multimedia content. Traditional approaches inadequately capture the psycholinguistic features essential for understanding the social context, intent, and emotional undertones embedded in the text. To address these shortcomings, this study introduces a novel PsychoLinguistic Multiplicative Attentive Network (PLiMA Net) that incorporates psycholinguistic analysis and Luong attention mechanism into RNN for fake news detection systems, aiming to enhance the accuracy and reliability of identifying misleading content. Previous contributions: (a) The first work focused on text-based fake news detection through the "Integrated Hierarchical Granular Retentive XLNet with Fast Embedded Semantic Extraction" method. This approach utilized innovative components such as Structural Transition Enhanced Parsing (STEP), Multi-Granular Entity Resolution (MulGER), and Fast-X-Ref Semantic Feature Extraction, achieving a remarkable accuracy of 97.67%. (b) The second work extended this framework by incorporating emojis into sentiment analysis where the "Multi-Token Concatenated Embedding and SemantiXpert Probabilistic Classifier" method was proposed, featuring Multi-Run Byte Pair Encoding (MRBPE) and a Concat-ViLT model for merging textual and visual representations and achieved an accuracy of 95.83%. By incorporating psycholinguistic analysis with Luong attention mechanism into those previous two works, this PLiMA Net model improves its precision and adaptability to the evolving linguistic area of social media, ensuring robust and trustworthy detection of fake news.
Keywords:
Sentiment analysis, Fake news detection, Social media news, Psycholinguistic features, Recurrent neural network, Attention mechanism.
References:
[1] Xiaochang Fang et al., “NSEP: Early Fake News Detection via News Semantic Environment Perception,” Information Processing & Management, vol. 61, no. 2, pp. 1-17, 2024.
[CrossRef] [Google Scholar] [Publisher Link]
[2] Alok Debnath et al., “Semantic Textual Similarity of Sentences with Emojis,” Companion Proceedings of the Web Conference, New York, United States, pp. 426-430, 2020.
[CrossRef] [Google Scholar] [Publisher Link]
[3] Alaa Altheneyan, and Aseel Alhadlaq, “Big Data ML-Based Fake News Detection Using Distributed Learning,” IEEE Access, vol. 11, pp. 29447-29463, 2023.
[CrossRef] [Google Scholar] [Publisher Link]
[4] Eslam Amer, Kyung-Sup Kwak, and Shaker El-Sappagh, “Context-Based Fake News Detection Model Relying on Deep Learning Models,” Electronics, vol. 11, no. 8, pp. 1-13, 2022.
[CrossRef] [Google Scholar] [Publisher Link]
[5] Ferdaous Benrouba, and Rachid Boudour, “Emotional Sentiment Analysis of Social Media Content for Mental Health Safety,” Social Network Analysis and Mining, vol. 13, 2023.
[CrossRef] [Google Scholar] [Publisher Link]
[6] Lisa Holthoff, “The Emoji Sentiment Lexicon: Analysing Consumer Emotions in Social Media Communication,” Proceedings of the 49th European Marketing Academy (EMAC) Annual Conference, pp. 1-10, 2020.
[Google Scholar] [Publisher Link]
[7] Yingxu Wang, and James Y. Xu, An Autonomous Fake News Recognition System by Semantic Learning and Cognitive Computing, Transactions on Computational Science XL, vol. 13850, pp. 88-109, 2023.
[CrossRef] [Google Scholar] [Publisher Link]
[8] Zahra Ahanin, and Maizatul Akmar Ismai, “Feature Extraction Based on Fuzzy Clustering and Emoji Embeddings for Emotion Classification,” International Journal of Technology Management and Information System, vol. 2, no. 1, pp. 102-112, 2020.
[Google Scholar] [Publisher Link]
[9] Pawan Kumar Verma et al., “MCred: Multi-Modal Message Credibility for Fake News Detection using BERT and CNN,” Journal of Ambient Intelligence and Humanized Computing, vol. 14, pp. 10617-10629, 2023.
[CrossRef] [Google Scholar] [Publisher Link]
[10] Ashfia Jannat Keya et al., “Fake-BERT: Handling Imbalance through Augmentation of Fake News Using BERT to Enhance the Performance of Fake News Classification,” Applied Sciences, vol. 12, no. 17, pp. 1-21, 2022.
[CrossRef] [Google Scholar] [Publisher Link]
[11] Wesam Shishah, “Fake News Detection Using BERT Model with Joint Learning,” Arabian Journal for Science and Engineering, vol. 46, pp. 9115-9127, 2021.
[CrossRef] [Google Scholar] [Publisher Link]
[12] Tong Zhang et al., “BDANN: BERT-based Domain Adaptation Neural Network for Multi-Modal Fake News Detection,” International Joint Conference on Neural Networks, Glasgow, UK, pp. 1-8, 2020.
[CrossRef] [Google Scholar] [Publisher Link]
[13] Bagus Satria Wiguna et al., “Sarcasm Detection Engine for Twitter Sentiment Analysis Using Textual and Emoji Feature,” Journal of Computer and Information Science, vol. 14, no. 1, pp. 1-8, 2021.
[CrossRef] [Google Scholar] [Publisher Link]
[14] K.E. Naresh Kumar, and V. Uma, “Intelligent Sentinet-Based Lexicon for Context-Aware Sentiment Analysis: Optimized Neural Network for Sentiment Classification on Social Media,” The Journal of Supercomputing, vol. 77, pp. 12801-12825, 2021.
[CrossRef] [Google Scholar] [Publisher Link]
[15] Byungkyu Yoo, and Julia Taylor Rayz, “Understanding Emojis for Sentiment Analysis,” The International FLAIRS Conference Proceedings, vol. 34, pp. 1-4, 2021.
[CrossRef] [Google Scholar] [Publisher Link]
[16] Rohit Kumar Kaliyar, Anurag Goswami, and Pratik Narang, “FakeBERT: Fake News Detection in Social Media with a BERT-Based Deep Learning Approach,” Multimedia Tools and Applications, vol. 80, pp. 11765-11788, 2021.
[CrossRef] [Google Scholar] [Publisher Link]
[17] Muhammad Imran Nadeem et al., “EFND: A Semantic, Visual, and Socially Augmented Deep Framework for Extreme Fake News Detection,” Sustainability, vol. 15, no. 1, pp. 1-24, 2023.
[CrossRef] [Google Scholar] [Publisher Link]
[18] Balasubramanian Palani, Sivasankar Elango, and K. Vignesh Viswanathan, “CB-Fake: A Multimodal Deep Learning Framework for Automatic Fake News Detection Using Capsule Neural Network and BERT,” Multimedia Tools and Applications, vol. 81, pp. 5587-5620, 2022.
[CrossRef] [Google Scholar] [Publisher Link]
[19] Mohammadreza Samadi, and Saeedeh Momtazi, “Fake News Detection: Deep Semantic Representation with Enhanced Feature Engineering,” International Journal of Data Science and Analytics, pp. 1-12, 2023.
[CrossRef] [Google Scholar] [Publisher Link]
[20] Ehab Essa, Karima Omar, and Ali Alqahtani, “Fake News Detection Based on a Hybrid BERT and LightGBM Models,” Complex & Intelligent Systems, vol. 9, pp. 6581–6592, 2023.
[CrossRef] [Google Scholar] [Publisher Link]
[21] Murtuza Shahzad et al., “Predicting Facebook Sentiments towards Research,” Natural Language Processing Journal, vol. 3, pp. 1-10, 2023.
[CrossRef] [Google Scholar] [Publisher Link]
[22] Qianwen Ariel Xu, Chrisina Jayne, and Victor Chang, “An Emoji Feature-Incorporated Multi-View Deep Learning for Explainable Sentiment Classification of Social Media Reviews,” Technological Forecasting and Social Change, vol. 202, pp. 1-23, 2024.
[CrossRef] [Google Scholar] [Publisher Link]
[23] Shelley Gupta, Archana Singh, and Vivek Kumar, “Emoji, Text, and Sentiment Polarity Detection Using Natural Language Processing,” Information, vol. 14, no. 4, pp. 1-18, 2023.
[CrossRef] [Google Scholar] [Publisher Link]
[24] Amira Samy Talaat, “Sentiment Analysis Classification System Using Hybrid BERT Models,” Journal of Big Data, vol. 10, pp. 1-18, 2023.
[CrossRef] [Google Scholar] [Publisher Link]
[25] Chuchu Liu et al., “Improving Sentiment Analysis Accuracy with Emoji Embedding,” Journal of Safety Science and Resilience, vol. 2, no. 4, pp. 246-252, 2021.
[CrossRef] [Google Scholar] [Publisher Link]
[26] Nilesh Shelke et al., “An Efficient Way of Text-Based Emotion Analysis from Social Media Using LRA-DNN,” Neuroscience Informatics, vol. 2, no. 3, pp. 1-10, 2022.
[CrossRef] [Google Scholar] [Publisher Link]
[27] Rolandos Alexandros Potamias, Georgios Siolas, and Andreas - Georgios Stafylopatis, “A Transformer-Based Approach to Irony and Sarcasm Detection,” Neural Computing and Applications, vol. 32, pp. 17309-17320, 2020.
[CrossRef] [Google Scholar] [Publisher Link]
[28] Muhammad Asif et al., “Sentiment Analysis of Extremism in Social Media from Textual Information,” Telematics and Informatics, vol. 48, 2020.
[CrossRef] [Google Scholar] [Publisher Link]