Federated Learning-Based Futuristic Fault Diagnosis and Standardization in Rotating Machinery
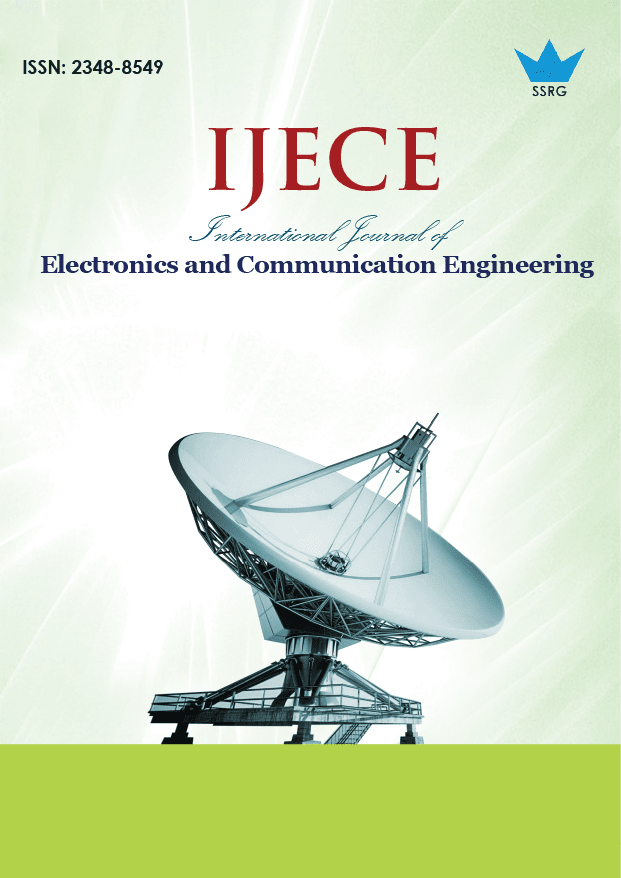
International Journal of Electronics and Communication Engineering |
© 2024 by SSRG - IJECE Journal |
Volume 11 Issue 9 |
Year of Publication : 2024 |
Authors : Vijayalakshmi K, Amuthakkannan Rajakannu,Ramachandran KP, Sri Rajkavin A V |
How to Cite?
Vijayalakshmi K, Amuthakkannan Rajakannu,Ramachandran KP, Sri Rajkavin A V, "Federated Learning-Based Futuristic Fault Diagnosis and Standardization in Rotating Machinery," SSRG International Journal of Electronics and Communication Engineering, vol. 11, no. 9, pp. 223-236, 2024. Crossref, https://doi.org/10.14445/23488549/IJECE-V11I9P120
Abstract:
In the industrial sector, intelligent sensors in fault diagnosis are becoming more critical in recent technological improvements. The prediction accuracy can be enhanced in fault diagnosis using 3-dimensional, sequential, real-time, and image data. Sensors that capture the vibration, sound, and image data are more critical in predicting unbalancing, tool wear, crack, misalignment, etc, in the rotating machinery to increase productivity and to provide an effective maintenance management system. Due to the fast development of industry 4.0 techniques, monitoring of mechanical machinery is experiencing explosive growth and getting more attention in the area of Fault Diagnosis (FD). Machine learning and Deep learning methods give promising results and accuracy in predicting faults in rotating machinery on shop floors. The success of AI-based models is due to the availability of comprehensive labeled data. Federated Learning (FL) is the machine learning subfield aiming to train an algorithm with a heterogeneous dataset. Data transmission from local facilities to a central server in AI models creates data privacy and security issues. Heterogeneous data analysis is a complicated process in predicting the machine fault in the central server because of millions of data during real-time condition monitoring. Decentralized data handling and analysis is mandatory in condition monitoring because of heterogeneity in data processing, data privacy, and security advantages. The application of federated learning in fault diagnosis has been getting more attention in recent days, and this study is a review of FL applications that address fault diagnosis in rotating machinery in the first phase. A comparison between the types of FL approaches in FD, and the use of aggregation algorithms and their applications will also be discussed in Phase 1. In phase 2, a novel methodology has been proposed using Federated learning to diagnose rotating machinery faults. The proposed method, FLOACOS, addresses how prediction challenges are solved using federated learning approaches by optimizing and standardizing the data at local facilities. This work will be helpful for future condition monitoring researchers and gives an overview of a novel method of the FL technique used in predicting faults and the progress made in the maintenance management of rotating machinery.
Keywords:
Fault diagnosis, Federated Learning, Internet of Things, Machine learning, Rotating machinery, Condition monitoring.
References:
[1] Manan Mehta et al., “A Federated Learning Approach to Mixed Fault Diagnosis In Rotating Machinery,” Journal of Manufacturing Systems, vol. 68, pp. 687-694, 2023.
[CrossRef] [Google Scholar] [Publisher Link]
[2] Weihua Li et al., “Clustering Federated Learning for Bearing Fault Diagnosis in Aerospace Applications with a Self-Attention Mechanism,” Aerospace, vol. 9, no. 9, pp. 1-19, 2022.
[CrossRef] [Google Scholar] [Publisher Link]
[3] Yaguo Lei et al., “Applications of Machine Learning to Machine Fault Diagnosis: A Review and Roadmap,” Mechanical Systems and Signal Processing, vol. 138, 2020.
[CrossRef] [Google Scholar] [Publisher Link]
[4] Ji Liu et al., “From Distributed Machine Learning to Federated Learning: A Survey,” Knowledge and Information Systems, vol. 64, pp. 885-917, 2022.
[CrossRef] [Google Scholar] [Publisher Link]
[5] Yaguo Lei, and Ming J. Zuo, “Gear Crack Level Identification Based on Weighted K Nearest Neighbor Classification Algorithm,” Mechanical Systems and Signal Processing, vol. 23, no. 5, pp. 1535-1547, 2009.
[CrossRef] [Google Scholar] [Publisher Link]
[6] B. Samanta, “Gear Fault Detection Using Artificial Neural Networks and Support Vector Machines with Genetic Algorithms,” Mechanical Systems and Signal Processing, vol. 18, no. 3, pp. 625-644, 2004.
[CrossRef] [Google Scholar] [Publisher Link]
[7] Ruonan Liu et al., “Artificial Intelligence for Fault Diagnosis of Rotating Machinery: A Review,” Mechanical Systems and Signal Processing, vol. 108, pp. 33-47, 2018.
[CrossRef] [Google Scholar] [Publisher Link]
[8] Rui Zhao et al., “Deep Learning and Its Applications to Machine Health Monitoring,” Mechanical Systems and Signal Processing, vol. 115, pp. 213-237, 2019.
[CrossRef] [Google Scholar] [Publisher Link]
[9] Qizhao Wang et al., “Efficient Federated Learning for Fault Diagnosis in Industrial Cloud-Edge Computing,” Computing, vol. 103, pp. 2319-2337, 2021.
[CrossRef] [Google Scholar] [Publisher Link]
[10] Zexi Yao et al., “DiagNet: Machine Fault Diagnosis Using Federated Transfer Learning in Low Data Regimes,” AAAI 2022 FL Workshop, 2022.
[Google Scholar] [Publisher Link]
[11] Wei Zhang et al., “Federated Learning for Machinery Fault Diagnosis with Dynamic Validation and Self-Supervision,” Knowledge-Based Systems, vol. 213, 2021.
[CrossRef] [Google Scholar] [Publisher Link]
[12] Manar Abdelmaksoud et al., “Convolutional-Neural-Network-Based Multi-Signals Fault Diagnosis of Induction Motor Using Single and Multi-Channels Datasets,” Alexandria Engineering Journal, vol. 73, pp. 231-248, 2023.
[CrossRef] [Google Scholar] [Publisher Link]
[13] Hao Lu et al., “Federated Learning with Uncertainty-Based Client Clustering for Fleet-Wide Fault Diagnosis,” Mechanical Systems and Signal Processing, vol. 210, 2024.
[CrossRef] [Google Scholar] [Publisher Link]
[14] Funa Zhou et al., “Fault Diagnosis Based on Federated Learning Driven by Dynamic Expansion for Model Layers of Imbalanced Client,” Expert Systems with Applications, vol. 238, 2024.
[CrossRef] [Google Scholar] [Publisher Link]
[15] Funa Zhou, Zhiqiang Zhang, and Sijie Li, “Research on Federated Learning Method for Fault Diagnosis in Multiple Working Conditions,” Complex Engineering Systems, vol. 1, no. 7, pp. 1-17, 2021.
[CrossRef] [Google Scholar] [Publisher Link]
[16] Subrato Bharati et al., “Federated Learning: Applications, Challenges and Future Directions,” arXiv, pp. 1-35, 2022.
[CrossRef] [Google Scholar] [Publisher Link]
[17] Jie Wen et al., “A Survey on Federated Learning: Challenges and Applications,” International Journal of Machine Learning and Cybernetics, vol. 14, pp. 513-535, 2023.
[CrossRef] [Google Scholar] [Publisher Link]
[18] Li Li et al., “A Review of Applications in Federated Learning,” Computers & Industrial Engineering, vol. 149, 2020.
[CrossRef] [Google Scholar] [Publisher Link]
[19] Afifa Salsabil Fathima et al., “Federated Learning Based Futuristic Biomedical Bigdata Analysis and Standardization,” PLoS ONE, vol. 18, no. 10, pp. 1-16, 2023.
[CrossRef] [Google Scholar] [Publisher Link]
[20] Krista Rizman Žalik, and Mitja Žalik, “A Review of Federated Learning in Agriculture,” Sensors, vol. 23, no. 23, pp. 1-20, 2023.
[CrossRef] [Google Scholar] [Publisher Link]
[21] Funa Zhou et al., “Federated Learning Based Fault Diagnosis Driven by Intra-Client Imbalance Degree,” Entropy, vol. 25, no. 4, pp. 1- 18, 2023.
[CrossRef] [Google Scholar] [Publisher Link]
[22] Mohammad Moshawrab et al., “Reviewing Federated Learning Aggregation Algorithms; Strategies, Contributions, Limitations and Future Perspectives,” Electronics, vol. 12, no. 10, pp. 1-35, 2023.
[CrossRef] [Google Scholar] [Publisher Link]
[23] Hongyu Yang, Joseph Mathew, and Lin Ma, “Vibration Feature Extraction Techniques for Fault Diagnosis of Rotating Machinery: A Literature Survey,” Asia-Pacific Vibration Conference, pp. 1-7, 2003.
[Google Scholar] [Publisher Link]
[24] E. Athanasopoulou, and C.N. Hadjicostis, “Synchronization-Based Fault Detection in Discrete Event Systems,” 2004 43rd IEEE Conference on Decision and Control, Nassau, Bahamas, vol. 1, pp. 57-62, 2004.
[CrossRef] [Google Scholar] [Publisher Link]
[25] Thien Duc Nguyen et al., “DÏoT: A Federated Self-learning Anomaly Detection System for IoT,” arXiv, pp. 1-12, 2018.
[CrossRef] [Google Scholar] [Publisher Link]
[26] Yan Song, and Peng Liu, “Federated Domain Generalization for Intelligent Fault Diagnosis Based on Pseudo-Siamese Network And Robust Global Model Aggregation,” International Journal of Machine Learning and Cybernetics, vol. 15, pp. 685-696, 2024.
[CrossRef] [Google Scholar] [Publisher Link]
[27] Tarek Berghout et al., “Federated Learning for Condition Monitoring of Industrial Processes: A Review on Fault Diagnosis Methods, Challenges, and Prospects,” Electronics, vol. 12, no. 1, pp. 1-20, 2023.
[CrossRef] [Google Scholar] [Publisher Link]
[28] Shiyi Jiang et al., “Federated Clustered Multi-Domain Learning for Health Monitoring,” Scientific Reports, vol. 14, no. 1, pp. 1-12, 2024.
[CrossRef] [Google Scholar] [Publisher Link]