Enhancing Mild Diabetic Retinopathy Detection: A Comparative Study of CLAHE-Preprocessed and Unprocessed Fundus Images Using a Minimal CNN Model
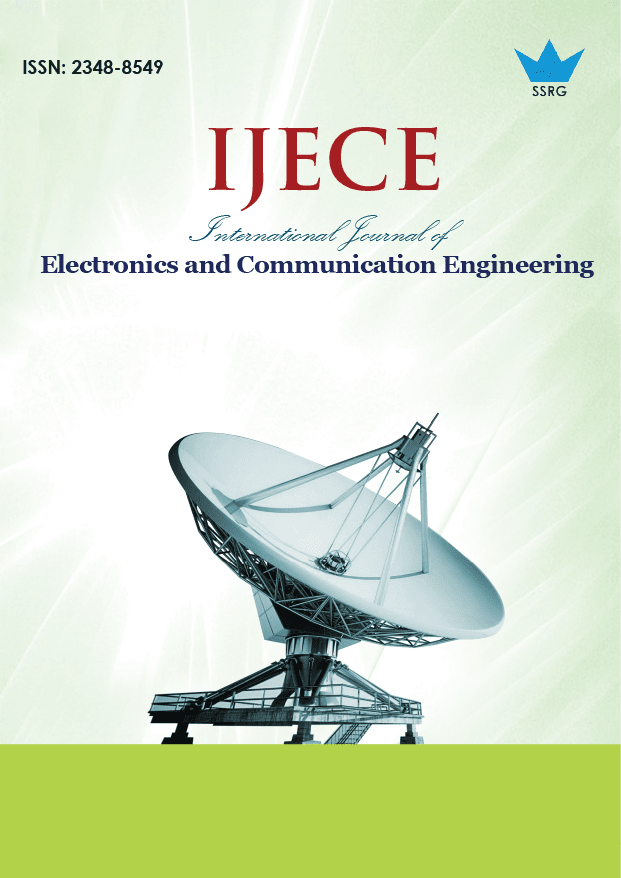
International Journal of Electronics and Communication Engineering |
© 2024 by SSRG - IJECE Journal |
Volume 11 Issue 9 |
Year of Publication : 2024 |
Authors : P.M. Ebin, P. Ranjana |
How to Cite?
P.M. Ebin, P. Ranjana, "Enhancing Mild Diabetic Retinopathy Detection: A Comparative Study of CLAHE-Preprocessed and Unprocessed Fundus Images Using a Minimal CNN Model," SSRG International Journal of Electronics and Communication Engineering, vol. 11, no. 9, pp. 280-289, 2024. Crossref, https://doi.org/10.14445/23488549/IJECE-V11I9P124
Abstract:
Early identification of Diabetic Retinopathy (DR) is crucial for preserving vision in individuals with diabetes, as this condition is a leading cause of sight loss among diabetic patients. Timely detection enables effective treatment interventions. Hence, we proposed a Minimal Convolutional Neural Network (MCNN) model for detecting mild DR symptoms using fundus images. Utilizing publicly available datasets from Kaggle and Messidor, the research applies Contrast Limited Adaptive Histogram Equalization (CLAHE) preprocessing to enhance image quality. The MCNN is then trained on both CLAHEprocessed and unprocessed versions of the same images. The research evaluates CLAHE preprocessing's effect on mild DR detection by analyzing the model's performance across both datasets, seeking to quantify any accuracy improvements. This approach leverages modern machine learning techniques to potentially improve early diagnosis of DR, addressing a critical need in ophthalmological practice and diabetic care.
Keywords:
AI, CLAHE, Deep learning, Diabetic Retinopathy, MCNN.
References:
[1] Sam E. Mansour et al., “The Evolving Treatment of Diabetic Retinopathy,” Clinical Ophthalmology, vol. 14, pp. 653-678, 2020.
[CrossRef] [Google Scholar] [Publisher Link]
[2] Skylar Stolte, and Ruogu Fang, “A Survey on Medical Image Analysis in Diabetic Retinopathy,” Medical Image Analysis, vol. 64, 2020.
[CrossRef] [Google Scholar] [Publisher Link]
[3] David A. Antonetti, Paolo S. Silva, and Alan W. Stitt, “Current Understanding of the Molecular and Cellular Pathology of Diabetic Retinopathy,” Nature Reviews Endocrinology, vol. 17, pp. 195-206, 2021.
[CrossRef] [Google Scholar] [Publisher Link]
[4] Maximilian W.M. Wintergerst et al., “Diabetic Retinopathy Screening using Smartphone-Based Fundus Imaging in India,” Ophthalmology, vol. 127, no. 11, pp. 1529-1538, 2020.
[CrossRef] [Google Scholar] [Publisher Link]
[5] K. Shankar et al., “Hyperparameter Tuning Deep Learning for Diabetic Retinopathy Fundus Image Classification,” IEEE Access, vol. 8, pp. 118164-118173, 2020.
[CrossRef] [Google Scholar] [Publisher Link]
[6] Gilbert Lim et al., “Different Fundus Imaging Modalities and Technical Factors in AI Screening for Diabetic Retinopathy: A Review,” Eye and Vision, vol. 7, pp. 1-13, 2020.
[CrossRef] [Google Scholar] [Publisher Link]
[7] Pelin Görgel, “Greedy Segmentation Based Diabetic Retinopathy Identification using Curvelet Transform and Scale Invariant Features,” Journal of Engineering Research, vol. 9, no. 1, pp. 134-150, 2021.
[CrossRef] [Google Scholar] [Publisher Link]
[8] Wejdan L. Alyoubi, Wafaa M. Shalash, and Maysoon F. Abulkhair, “Diabetic Retinopathy Detection through Deep Learning Techniques: A Review,” Informatics in Medicine Unlocked, vol. 20, pp. 1-11, 2020.
[CrossRef] [Google Scholar] [Publisher Link]
[9] K. Shankar et al., “Automated Detection and Classification of Fundus Diabetic Retinopathy Images Using Synergic Deep Learning Model,” Pattern Recognition Letter, vol. 133, pp. 210-216, 2020.
[CrossRef] [Google Scholar] [Publisher Link]
[10] Morgan Heisler et al., “Ensemble Deep Learning for Diabetic Retinopathy Detection Using Optical Coherence Tomography Angiography,” Translational Vision Science & Technology, vol. 9, no. 2, pp. 1-11, 2020.
[CrossRef] [Google Scholar] [Publisher Link]
[11] Ibrahem Kandel, and Mauro Castelli, “Transfer Learning with Convolutional Neural Networks for Diabetic Retinopathy Image Classification. A Review,” Applied Sciences, vol. 10, no. 6, pp. 1-24, 2020.
[CrossRef] [Google Scholar] [Publisher Link]
[12] Rupa Patel, and Anita Chaware, “Transfer Learning with Fine-Tuned MobileNetV2 for Diabetic Retinopathy,” 2020 International Conference for Emerging Technology (INCET), Belgaum, India, pp. 1-4, 2020.
[CrossRef] [Google Scholar] [Publisher Link]
[13] David Le et al., “Transfer Learning for Automated OCTA Detection of Diabetic Retinopathy,” Translational Vision Science & Technology, vol. 9, no. 2, pp. 1-9, 2020.
[CrossRef] [Google Scholar] [Publisher Link]
[14] Nikki Gaikwad et al., “Automated System for Detection for Diabetic Retinopathy using Image Processing,” International Research Journal of Engineering and Technology, vol. 7, no. 8, pp. 4980-4985, 2020.
[Google Scholar] [Publisher Link]
[15] Sarni Suhaila Rahim, Vasile Palade, and Andreas Holzinger, Image Processing and Machine Learning Techniques for Diabetic Retinopathy Detection: A Review, Artificial Intelligence and Machine Learning for Digital Pathology, pp. 136-154, 2020.
[CrossRef] [Google Scholar] [Publisher Link]
[16] Sehrish Qummar et al., “A Deep Learning Ensemble Approach for Diabetic Retinopathy Detection,” IEEE Access, vol. 7, pp. 150530- 150539, 2019.
[CrossRef] [Google Scholar] [Publisher Link]
[17] Along He et al., “CABNet: Category Attention Block for Imbalanced Diabetic Retinopathy Grading,” IEEE Transactions on Medical Imaging, vol. 40, no. 1, pp. 143-153, 2021.
[CrossRef] [Google Scholar] [Publisher Link]
[18] Teresa Araújo et al., “Data Augmentation for Improving Proliferative Diabetic Retinopathy Detection in Eye Fundus Images,” IEEE Access, vol. 8, pp. 182462-182474, 2020.
[CrossRef] [Google Scholar] [Publisher Link]
[19] Lifeng Qiao, Ying Zhu, and Hui Zhou, “Diabetic Retinopathy Detection Using Prognosis of Microaneurysm and Early Diagnosis System for Non-Proliferative Diabetic Retinopathy Based on Deep Learning Algorithms,” IEEE Access, vol. 8, pp. 104292-104302, 2020.
[CrossRef] [Google Scholar] [Publisher Link]
[20] Nikhil Barhate et al., “Reducing Overfitting in Diabetic Retinopathy Detection using Transfer Learning,” 2020 IEEE 5th International Conference on Computing Communication and Automation (ICCCA), Greater Noida, India, pp. 298-301, 2020.
[CrossRef] [Google Scholar] [Publisher Link]
[21] Xiaomeng Li et al., “CANet: Cross-Disease Attention Network for Joint Diabetic Retinopathy and Diabetic Macular Edema Grading,” IEEE Transactions on Medical Imaging, vol. 39, no. 5, pp. 1483-1493, 2020.
[CrossRef] [Google Scholar] [Publisher Link]
[22] Zubair Khan et al., “Diabetic Retinopathy Detection Using VGG-NIN a Deep Learning Architecture,” IEEE Access, vol. 9, pp. 61408- 61416, 2021.
[CrossRef] [Google Scholar] [Publisher Link]
[23] Fahman Saeed, Muhammad Hussain, and Hatim A. Aboalsamh, “Automatic Diabetic Retinopathy Diagnosis Using Adaptive Fine-Tuned Convolutional Neural Network,” IEEE Access, vol. 9, pp. 41344-41359, 2021.
[CrossRef] [Google Scholar] [Publisher Link]
[24] Cam-Hao Hua et al., “Convolutional Network with Twofold Feature Augmentation for Diabetic Retinopathy Recognition from Multi-Modal Images,” IEEE Journal of Biomedical and Health Informatics, vol. 25, no. 7, pp. 2686-2697, 2021.
[CrossRef] [Google Scholar] [Publisher Link]
[25] Harshit Kaushik et al., “Diabetic Retinopathy Diagnosis From Fundus Images Using Stacked Generalization of Deep Models,” IEEE Access, vol. 9, pp. 108276-108292, 2021.
[CrossRef] [Google Scholar] [Publisher Link]
[26] R.S. Rajkumar et al., “Transfer Learning Approach for Diabetic Retinopathy Detection using Residual Network,” 2021 6th International Conference on Inventive Computation Technologies (ICICT), Coimbatore, India, pp. 1189-1193, 2021.
[CrossRef] [Google Scholar] [Publisher Link]
[27] G. Kalyani et al., “Diabetic Retinopathy Detection and Classification using Capsule Networks,” Complex & Intelligent Systems, vol. 9, pp. 2651-2664, 2023.
[CrossRef] [Google Scholar] [Publisher Link]
[28] Md. Nahiduzzaman et al., “Diabetic Retinopathy Identification using Parallel Convolutional Neural Network based Feature Extractor and ELM Classifier,” Expert Systems with Applications, vol. 217, pp. 1-11, 2023.
[CrossRef] [Google Scholar] [Publisher Link]
[29] Tiwalade Modupe Usman et al., “Diabetic Retinopathy Detection using Principal Component Analysis Multi-Label Feature Extraction and Classification,” International Journal of Cognitive Computing in Engineering, vol. 4, pp. 78-88, 2023.
[CrossRef] [Google Scholar] [Publisher Link]
[30] Rajasekhar Kommaraju, and M.S. Anbarasi, “Diabetic Retinopathy Detection Using Convolutional Neural Network with Residual Blocks,” Biomedical Signal Processing and Control, vol. 87, no. A, 2024.
[CrossRef] [Google Scholar] [Publisher Link]